改进BP神经网络模型及其稳定性分析
张国翊,胡铮
(北京邮电大学 泛网无线通信教育部重点实验室,北京,100876)
摘 要:针对传统BP算法抗干扰能力差、学习速率慢且易陷入局部极小点等缺点,提出一种基于变更传递函数倾斜度和动态调节不同学习速率的BP改进算法,并在此基础上采用Lyapunov稳定性原理分析改进算法的收敛性。该算法综合考虑网络训练方式和学习率的不足,设计新的复合误差函数,同时采用一种分层动态调整不同学习率的新方法,并采用批量样本进行训练,以加快传统BP算法的收敛速度和避免陷入局部极小值点。在此基础上,将该算法应用于带钢表面缺陷图像检测中,并比较改进算法与传统算法在缺陷检测中的性能参数。研究结果表明:该改进算法能够提高缺陷识别率,检测速度快,更能满足钢板表面质量检测的实时性要求,是一种行之有效的 方法。
关键词:BP神经网络;缺陷检测;复合误差函数;Lyapunov稳定性
中图分类号:TP183 文献标志码:A 文章编号:1672-7207(2011)01-0115-10
Improved BP neural network model and its stability analysis
ZHANG Guo-yi, HU Zheng
(Key Laboratory of Universal Wireless Communications of Ministry of Education,
Beijing University of Posts and Telecommunications, Beijing 100876, China)
Abstract: As for shortcomings of classical BP algorithm such as bad anti-jamming ability, low learning rate and easy plunging into local minimum, a new kind of improved BP algorithm was proposed with varying slope of activation function and dynamically adjusting different learning rates. Moreover, the convergence of this improved algorithm was analyzed based on the principle of Lyapunov stability. Considering the deficiency of network training and insufficient learning rate, a new composite error function was invented. A new method of dynamic adjustment of different learning rate was adopted to accelerate the convergence of classical BP algorithm, and to avoid plunging into the local minimum point. The proposed algorithm was applied to the inspection of the surface defective image of steel strips and compared with traditional algorithm with defect detection performance parameters. The results show that the improved BP algorithm has many merits such as high inspection speed, high discrimination and real-time capacity which can satisfy the demand of defect detection on steel plate surface, so it is an effective method.
Key words: BP neural network; defect detection; composite error function; Lyapunov stability
未来的通信网络将是一个泛在、异构的网络模式,需要能够实时感知周围的网络环境,通过对所处环境的理解,实时调整通信网络的配置,智能地适应所处环境的变化,同时它还具备从变化中学习的能力,且能用于未来的决策中。在这种情况下,寻找一种能够实时感知网络环境变化,实现网络对环境自适应的方法就显得尤为重要。人工神经网络理论作为一门新兴学科,近年来被广泛应用于通信领域(如网络自配置、自优化与自管理)和工业领域(如缺陷识别与分类)中,其所具有的实时性、容错性和学习性等特征为各领域问题的解决提供了有效的理论和技术支撑。然而,目前,普遍采用基于误差逆传播的BP学习算法,主要存在2个问题,即收敛速度慢和易陷入局部极小。从神经网络在模式识别中的发展来看,大型网络结构的优化问题和网络学习问题还亟待进一步解决和提 高[1-2]。目前网络模型的改进主要包括网络结构和学习算法改进2个方面。BP网络结构的改进主要在互连方式、转换函数以及隐含层节点等方面,而算法改进主要通过修改误差函数、增大激励函数的导数以及与其他智能算法相结合等来提高网络性能。在此,本文作者主要通过构造了复合误差函数来避免局部极小,同时采用一种分层调整不同学习率的新方法来加快收敛速度,提出一种新的学习算法,并在此基础上采用Lyapunov稳定性原理分析改进算法的收敛性。
1 传统BP算法
这里,以3层BP网络为例。假设输入层、隐含层和输出层的神经元个数分别为N,M和L,对应任意输入样本向量为
, 1≤k≤P,实际输出向量为
,期望输出向量为
,输入层到隐含层的连接权值为wij(1≤i≤N, 1≤j≤M),隐含层至输出层的连接权值为vjt(1≤j≤M, 1≤t≤L),隐含层各单元的输出阈值为
(1≤j≤M),输出层各单元的输出阈值为
(1≤t≤L),g(x)和f(x)分别为隐含层和输出层的传递函数。设m为训练网络的迭代次数,连接权值和实际输出都是m的函数。
用第k个输入样本
,连接权值wij和阈值
计算隐含层各神经元的输入
,然后,用
通过g(x)传递函数计算隐含层各神经元的输出
:
;j=1, 2, …, M (1)
;j=1, 2, …, M (2)
同样,用隐含层的输出
、连接权值vjt和阈值
计算输出层各神经元的输入
,然后,用
通过f(x)传递函数计算输出层各神经元的响应
:
;t=1, 2, …, L (3)
;t=1, 2, …, L (4)
则对于所有训练样本,神经网络实际输出值
与理想期望值
间的全局均方误差函数表示为:

(5)
式中:
,表示网络第m次迭代时输出层第t个神经元的误差。
对于传统BP算法,通常选择以Sigmoid型函数作为输出层和隐含层的传递函数,即
(6)
式中:
。
从优化理论上来说,BP算法是一种梯度下降法,传统BP算法中的学习率就是梯度下降法的步长。BP算法中网络参数每次调节的幅度,均以一个与网络误差函数成正比的学习率
进行。在传统BP算法中,无论是在隐含层还是在输出层,在其连接权值和阈值参数的修正过程中,学习率
总是固定不变的[1-2]。
2 传统BP算法的不足
在实际应用中,传统BP算法主要存在以下几个不足[2-3]:
(1) 学习算法的收敛速度比较慢。在处理图像缺陷在线检测这样一个比较复杂的问题时,由于系统实时性要求很高,这就要求学习算法的收敛速度要更快。
(2) 易陷入局部极小值。BP网络采用梯度下降法,训练是从某一起始点开始的斜面逐渐达到误差的最小值。对于复杂网络,其误差函数为多维空间的曲面,因而在其训练过程中,可能陷入某一局部极小值。虽然传统算法在权值更新时增加了新的冲量项以避免陷入局部极小值,但效果并不明显。
(3) 网络隐含层的层数以及隐含层神经元数的选取尚无理论指导,而是根据经验选取,因此,网络往往有很大的冗余性,也增加了网络学习的时间。
(4) 网络的学习、记忆具有不稳定性,新加入的样本会影响到已学完的样本。
3 新的BP改进算法
3.1 网络训练方式及传递函数的改进
在冷轧带钢表面缺陷检测系统中,由于训练网络时的样本数量较多,而且具有一定数量的重复样本,所以,在BP改进算法中,尝试采用分组批处理的训练方式,即假设先把所有P个训练样本分为n组,每组的样本数为Pi(1≤i≤n),然后对Pi个样本进行网络训练,让每组样本循环经过1次学习后再调整学习率,而不是每输入1个样本就调整1次学习率。采用分组批处理方式训练网络的优点是:既能使不同学习率在每组样本图像循环训练完成后得到适当调整,又能减小调整学习率的时间。因此,分组批处理的训练方式中,对于各分组Pi (1≤i≤n)个训练样本,神经网络实际输出值(
)与理想输出值(
)间的全局均方误差函数表示为:
(7)
其中:
,表示输出层第t个神经元的误差。
同时,对于新的BP改进算法,这里选择以
为倾斜度参数的双曲正切S型函数作为隐含层的传递函数,即
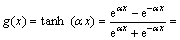
,
(8)
该传递函数是以
为倾斜度参数的单调递增的有界函数,它的基本特性包括:
,
,
,

(9)
图1所示为倾斜度参数
取5,3,1和0.5这4个不同值时的传递函数
曲线;图2所
示为用
线性逼近传递函数时的误差率。
图1中横坐标的步长取0.1,图2中横坐标的 步长取0.01,纵坐标的计算公式为:
。从图1和图2
可以看出:
越小,非线性传递函数g(x)越接近于线性函数
,并且线性逼近的误差率也越小。基于以上传递函数的基本特性,隐含层的修正量
和阈值
与非线性传递函数以及倾斜度参数
是成比例的。为了花费更少的训练时间和减少每次训练的计算量,期望降低传递函数的
,使非线性运算用线性运算代替;但降低
使得整个非线性网络更趋于线性网络,这在一定程度上大大降低了多层非线性网络在缺陷检测识别应用上的优势,因此,必须选择1个传递函数最佳倾斜度
,以权衡网络训练的速度和缺陷检测的效果[4-5]。
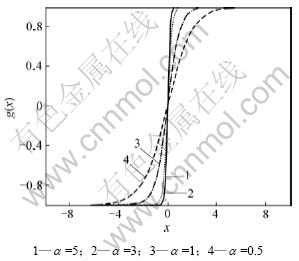
图1 传递函数曲线
Fig.1 Transfer function curves
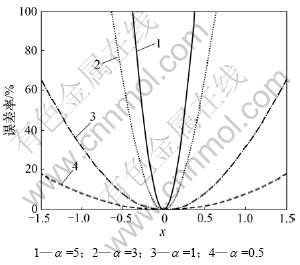
图2 线性逼近传递函数的误差率
Fig.2 Error rate of linear approximation transfer function
3.2 复合误差函数的设计
BP算法本质上是以误差平方和函数为目标函数,用梯度下降法求其最小值的算法。在训练样本的初始阶段,输出值远离期望值,E相对较大,
有较大的下降空间,所以,E对加快网络的收敛速度有较大的贡献;随着训练样本以及训练次数的逐渐增加,输出值逐渐靠近期望值,E不断减小,
的下降空间也不断缩小,此时,网络的收敛速度将变得非常缓慢。同时,由于全局均方误差函数E是一个非线性函数,意味着由E构成的连接空间不是只有1个极小点的抛物面,而是存在多个局部极小点的超曲面,因此,误差逆传播网络的收敛过程很可能进入局部极小点,而无法最终收敛到全局最小点。导致这一缺陷的原因是BP学习规则采用了按误差函数梯度下降方向进行收敛[3, 6-7]。为此,本文构造了复合误差函数
,并用
来代替传统算法中的全局均方误差函数E(m)。复合误差函数
的具体展开式为:


(10)
其中:
;
,为误差变化量;
为误差变化率;E(m)是分组样本在第m次循环学习时的全局均方 误差。
在训练样本的初始阶段网络的实际输出与期望输出相差较大,
,此时
,即为全局均方误差函数,网络收敛速度加快;随着训练样本以及训练次数的逐渐增加,E不断减小,当λ从1趋向于0时,
部分贡献值增大,此时,
,网络收敛速度同样加快,这在一定程度上克服了传统BP算法收敛速度慢的缺点。对于函数
收敛性,当
时,复合误差函数能使E(m)和EG(m)同时达到极小,收敛性与E(m)的收敛性是一致的。
采用
与作为λ参数的原因是:当?E(m)>0时,E(m)>E(m-1),说明全局均方误差E(m)增大,输出值远离期望值。由于误差变化量?E(m)相对E(m)要小得多,此时
能够迅速减小,使得λ趋于1,λE(m)部分贡献值增大,能够加快网络收敛速度;当?E(m)<0时,E(m)<E(m-1),说明全局均方误差E(m)减小,输出值靠近期望值。此时,虽然误差变化量?E(m)很小,但误差变化率
相对?E(m)要大得多,因此,
能够迅速增大,使得λ趋于0,(1-λ)EG(m)部分贡献值增大,同样也能加快网络收敛速度。
3.3 分层动态调节不同学习速率
BP算法中网络参数每次调节的幅度均以1个与网络误差函数成正比的学习率η进行。在传统BP算法中,无论是在隐含层还是在输出层,在其连接权值和阈值参数的修正过程中,学习率η总是固定不变的。其原因是误差函数E是一个复杂的非线性函数,很难通过求极小值的方法得到最佳步长η。在误差曲面较平坦处,连接权值和阈值参数的调节幅度较小,以致需要经过多次调整才能将误差函数曲面降低;而在误差曲面较陡处,连接权值和阈值参数的调节幅度较大,误差函数在最小点附近将发生过冲现象,使得权值参数调节路径呈现锯齿形,难以收敛到最小值点。这也是传统BP算法收敛速度慢的一个重要原因[5, 8]。
本文采用一种分层动态调整不同学习率的新方法,即分开调整输出层学习率η1和隐含层学习率η2,从而替代了传统算法中固定不变的学习率η。根据Delta学习规则,隐含层至输出层的连接权值vjt和输入层到隐含层的连接权值wij的修正量分别为:
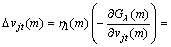
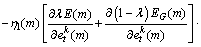
(11)
其中:
;
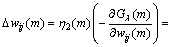
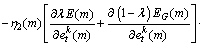

(12)
其中:


同时,在BP改进算法中,对学习率
和
分别采用如下修正公式:
, 0<
<1
, 0<
<1 (13)
式中:
是分组样本在第m+1次循环学习时输出层的学习率;
是分组样本在第m+1次循环学习时隐含层的学习率;
为分组样本在第m次循环学习时的组内均方误差变化率;
为常数,取值范围为0<
<1,这里统一取
=0.5。
采用误差学习率作为修正参数的原因是:当
>0时,说明学习误差增大,输出值远离期望值,权值调整过大,需要减小
和
。由式(11)和式(12)可知:若降低学习率
和
,
和
也必然减小。同时由式(13)可以看出:
<
,
<
,
和
减小,故能加快网络收敛速度;当
<0时,说明学习误差减小,输出值靠近期望值,需要增大
和
。但此时误差已经很小,
的变化也小,收敛速度很慢,但
比
要大得多,因此,从式(13)可以看出,
>
,
>
,
和
明显增大,同样也能加快网络收敛速度[9]。
因此,BP改进算法中输出层和隐含层各神经元的连接权值vjt和wij修正公式分别为:
(14)
(15)
3.4 BP改进算法稳定性分析
学习率的选择对神经网络的运行性能至关重要,学习率太小会使网络收敛太慢,学习率太大则会使网络的运行不稳定。为了高效地训练神经网络,从离散型的Lyapunov稳定性方法出发,以BP改进算法为例,给出保证神经网络稳定性的自适应学习率[10-11]。
结论1:若
满足:
0<
<
,
则由上述学习算法所表达的对权值
的迭代学习过程稳定收敛。
结论2:若
满足:
0<
<
,
则由上述学习算法所表达的对权值
的迭代学习过程稳定收敛。
首先证明结论1。
定义Lyapunov能量函数与传统误差函数类似,其表达式为:
(16)
于是,有:

(17)
学习过程的误差可以表示为:

(18)
其中隐含层到输出层连接权值
的修正量是:

(19)
于是,有:
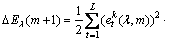
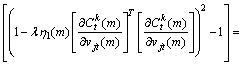
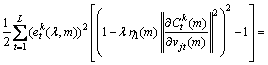
(20)
其中:

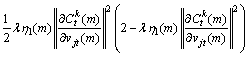
这里把
看成1个
维度向量。注意到BP改进算法中,选择Sigmoid函数作为输出层的传递函数,即
,而0<
≤1/4,所以,
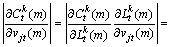
(21)
按照欧氏范数的定义,有:
(22)
从而,当0<
<
时,
有
>0,此时
<0。根据离散型Lyapunov稳定性原理,当
时,
将收敛到0。由于
时,
,此时
收敛到0,所以可知训练误差
将收敛到零,结论1证毕。
同理可以证明结论2。
由上述定理的结论可知:当
满足0<
<
时,可保证网络训练收敛。但当
时,
达到最小,即网络能以最快速度收敛。若近似认为
,则取
,可以保证网络以较快速度收敛。同理,可取
,以保证网络较快收敛。
4 缺陷检测试验及分析
4.1 算法描述
基于上述分析,采用BP改进算法进行缺陷检测程序的具体实现步骤如下。
(1) 初始化连接权值(wij和vjt)、阈值(θj和γt)、学习率(η1和η2)和传递函数倾斜度参数
。假设把所有P个训练样本分为n组,每组的样本数为Pi(1≤i≤n),设定组内期望误差标准ε以及最大循环迭代次数M。设
,1≤k≤Pi为组内第m次迭代时网络的实际输出量,
,1≤k≤Pi为组内期望输出量,m为循环迭代次数,令m=0。
(2) 随机输入该组内任意样本
,1≤k≤Pi。
(3) 对输入样本
,按式(1)和(3)分别计算隐含层和输出层的输入值;按式(2)和(4)分别计算隐含层和输出层的输出值。其中隐含层的传递函数选择以
为倾斜度参数的双曲正切S型函数
,输出层的传递函数选择常见的Sigmoid型函数
。
(4) 由期望输出值yk和上一步求得的该样本实际输出值Ck(m),计算该样本的均方误差
。判断该组所有Pi个训练样本是否都学习完毕,若是,则按式(9)计算该组训练样本的全局均方误差,并令m=m+1;否则,转至(2)。
(5) 判断循环学习次数m是否大于最大循环迭代次数M,若是,则转至(9),否则判断该组训练样本的第m次循环迭代的全局均方误差|E(m)|是否小于期望误差标准ε;或者判断连接权值的变化是否已经很小,即|vjt(m+1)-vjt(m)|<ε,|wij(m+1)-wij(m)|<ε,若是,则转至(7),否则转至(6)。
(6) 判断?E,若?E>0.001,则需要重新设置该组样本不同学习率η1(m),η2(m)和传递函数倾斜度参数
,并重新返回(2);若?E<0.001,则对于该组所有输入样本
,1≤k≤Pi,先按式(13)修正学习率η1(m)和η2(m),接着按式(14)和(15)分别修正输出层各神经元的连接权值vjt(m)和隐含层各神经元的连接权值wij(m)。
(7) 对于同组训练样本,在学习率(η1(m)和η2(m))不变的条件下,判断
改变次数是否超过3次,若是,则转至(8);否则,适当改变传递函数倾斜度
,并重新返回(2)。
(8) 比较相同学习率(η1(m)和η2(m))和不同
参数值条件下,该组训练样本全局均方误差E,得出使该组样本均方误差最小时的
。
(9) 算法结束。
BP改进算法流程如图3所示。
4.2 训练样本选择与获取
试验中选取带钢常见的6种表面缺陷样本图像训练网络,包括压痕、氧化色、擦伤、飞边、孔洞和辊印。训练样本图像的获取方式如下:专业质检人员对现场采集的冷轧带钢样本图像的缺陷图像和标准图像进行集中标定后,从中挑选出750张典型的、能代表各类缺陷的样本图像作为训练样本图像,其中绝大部分都是本文所涉及的常见的几种缺陷类型,但缺陷图像质量以及缺陷部分的方位不尽相同,如:由于摄像机现场受外界干扰导致拍摄图像放大或缩小比例不同,使得缺陷部分清晰度不一样;由于冷轧机器设备等原因造成同类缺陷的缺陷方向或位置不一致,训练图像是经过水平旋转不同度数如45°,90°,135°或180°形成的。值得提出的是:由于冷轧带钢生产现场环境很复杂,存在着各种各样干扰和噪声,所以,采集得到的冷轧带钢样本图像难免有不同程度的失真现象,这就要求系统在提取样本图像特征向量之前先进行图像的预处理工作(去噪、滤波),否则容易引起系统误检,从而得出错误的结论。
4.3 试验结果分析
冷轧带钢表面缺陷检测系统中采用以上BP改进算法,网络仍采用3层结构检测方式采用样本图像内任意区域的实时在线检测方法,即对于样本图像中有缺陷的部分,用任意矩形框将该区域框起来后,对该矩形框区域内的所有像素点从右到左、从下到上进行扫描,计算出各像素点的灰度,并提取该矩形框区域内图像的NMI特征[12]、低阶不变矩(M1,M2,M3和M4)特征,矩形框区域所有像素点的灰度平均值和灰度方差总共7个特征量作为缺陷图像的特征矢量,并将上述特征矢量作为网络的输入,隐含层设有15个神经元,随机初始权值和初始阈值范围均为-0.5~0.5,期望误差标准ε=0.000 5,最大迭代次数设为2 000。
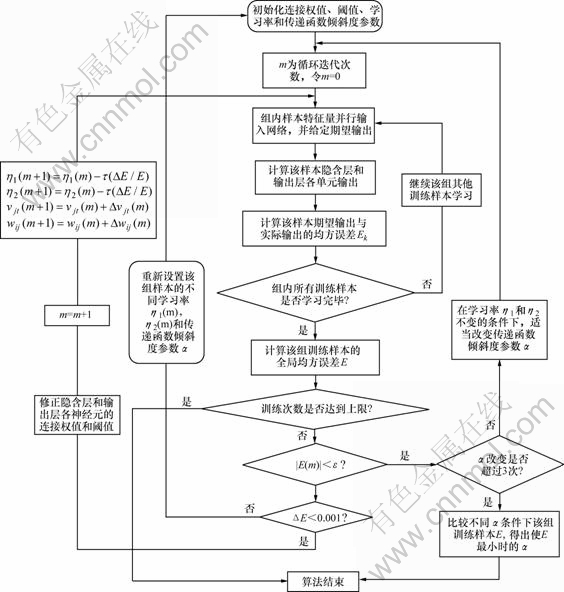
图3 BP改进算法流程图
Fig.3 Flow chart of improved BP algorithm
同时,考虑到带钢表面质量检测时,有可能出现误判,因此,只简单考虑网络的输出响应为“有缺 陷”(0, 0)和“无缺陷”(1, 1) 2种情况,所以,输出层神经元数目为2。系统中选择隐含层的传递函数为倾斜度参数
的双曲正切S型函数g(x),输出层的传递函数为常见的Sigmoid型函数f(x)。为避免学习算法不收敛,提高学习速度,设期望输出为相应小数,即(0.000 1, 0.000 1)判为“有缺陷”,(0.999 9, 0.999 9)判为“无缺陷”。
在缺陷检测试验中,首先把挑选出来的750个训练样本分成10组,前4组每组样本数为90,后6组每组样本数为65。对于各组样本,在学习率η1和η2一定的条件下,比较3个不同倾斜度参数
所对应的组内全局均方误差,其中中间
所对应的组内全局均方误差最小,具体实验结果如表1所示。从表1可以看出:对于数量较多的训练样本组,在学习率η1和η2一定的条件下,倾斜度参数
不同取值所对应的组内全局均方误差相差较大;而对于数量较少的训练样本组,在学习率η1和η2一定的条件下,倾斜度参数
不同取值所对应的组内全局均方误差相差较小。
为研究倾斜度参数
取不同值时对BP改进算法收敛情况以及各组组内均方误差的影响,从表1的10组训练样本中分别选取第1,3,6和9组进行BP网络训练仿真实验,仿真结果如图4所示。其中:第1和3组每组样本数为90,第6和9组每组样本数为65。对于各组训练样本,在学习率η1和η2一定的条件下,比较倾斜度参数
取3个不同值时所对应的组内均方误差和训练次数。从图4可以看出:当
取中间值时,BP改进算法收敛速度最快,网络在250~350步之间就收敛,并且此时所对应的组内全局均方误差能达到最小值,即
取中间值的点划线总是位于仿真图的下方;因此,根据图4训练次数和均方误差,同样可确定每组训练样本中
的最佳值。从图4还可以看出:对于数量较多的第1和3组训练样本,在学习率η1和η2一定的条件下,
不同取值所对应的组内均方误差相差较大;而对于数量较少的第6和9组训练样本,在学习率η1和η2一定的条件下,
不同取值所对应的组内均方误差相差较小。
表1 BP改进算法分组训练实验结果
Table 1 Improved BP algorithm group training results
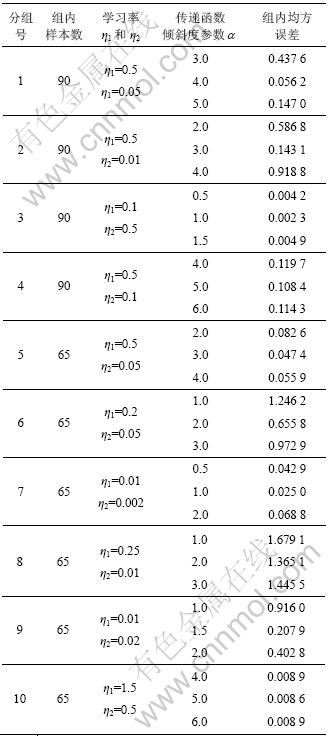
4.4 算法性能比较
为了便于分组比较传统算法和改进算法的性能,从现场采集的冷轧带钢缺陷图像中同样选取750个测试样本进行组内全局均方误差的计算。考虑到现场采集情况复杂,这750个测试样本不免与表1中分组训练网络的学习样本有较大重复,在计算BP改进算法的组内全局均方误差时,可以采用表1中各分组的最佳参数值,具体分组测试实验结果如表2所示。
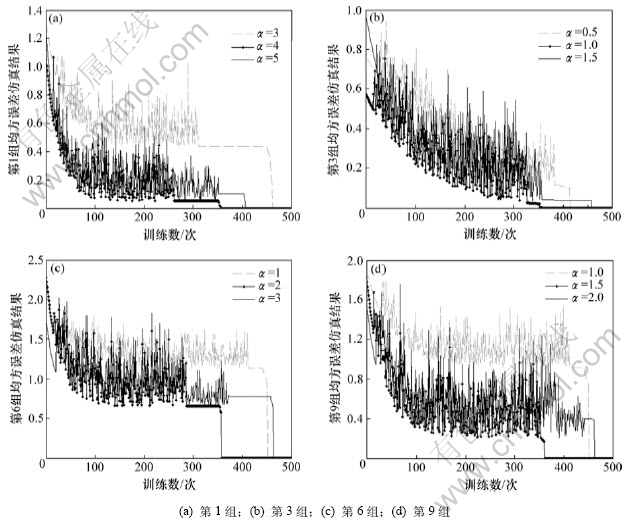
图4 BP改进算法分组网络训练仿真结果
Fig.4 Simulation results for improved BP algorithm group training
表2 BP传统算法和BP改进算法分组测试实验结果
Table 2 Experimental results comparison between traditional and improved BP algorithm
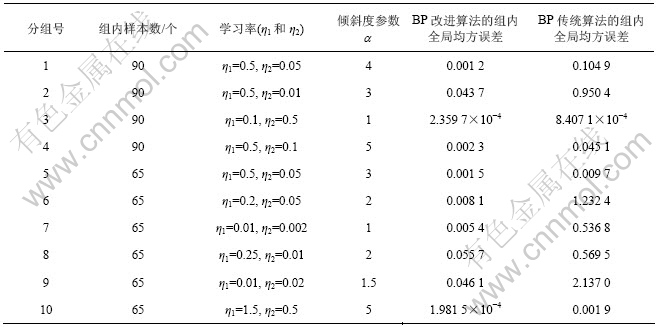
从表2可以看出:各组中BP改进算法的组内全局均方误差均明显小于BP传统算法的组内全局均方误差,而对于总体750个测试样本,BP改进算法的总体全局均方误差为0.016 81,BP传统算法的总体全局均方误差0.558 90,BP改进算法的全局均方误差只有传统算法的1/30。该改进算法通过变更传递函数倾斜度以及动态调节不同学习速率,很好地克服了传统BP神经网络抗干扰能力差、学习速度慢且易陷入局部极小点等缺点,使得传统BP算法性能进一步提高。
5 结论
(1) 通过分析传统BP算法的缺点及其成因,改进了网络训练方式及传递函数,构造了新的复合误差函数,同时采用了一种分层动态调整不同学习率的新方法,得到一种新的BP改进算法,并采用Lyapunov稳定性方法对该算法的收敛性进行了分析。
(2) 改进算法在收敛速度和避免局部极小方面明显优于传统BP算法,很好地克服了传统BP神经网络抗干扰能力差、学习速度慢且易陷入局部极小点等缺点,使得BP算法性能进一步提高,是一种训练BP网络的有效方法。
参考文献
[1] 张海东, 赖康生, 代东明, 等. 钢板无损检测中基于模糊神经网络的参数识别[J]. 计算机测量与控制, 2003, 11(1): 14-17.
ZHANG Hai-dong, LAI Kang-sheng, DAI Dong-ming, et al. Application of fuzzy neural network on parameter recognition of steel strip’s nondestructive testing[J]. Computer Automated Measurement & Control, 2003, 11(1): 14-17.
[2] 王越, 曹长修. BP网络局部极小产生的原因分析及避免[J]. 计算机工程, 2002, 28(6): 35-37.
WANG Yue, CAO Chang-xiu. Analysis of local minimization for BP algorithm and its avoidance methods[J]. Computer Engineering, 2002, 28(6): 35-37.
[3] 陈斌, 万江文, 吴银锋, 等. 神经网络和证据理论融合的管道泄漏诊断方法[J]. 北京邮电大学学报, 2009, 32(1): 9-13.
CHEN Bin, WAN Jiang-wen, WU Yin-feng, et al. A pipeline leakage diagnosis for fusing neural network and evidence theory[J]. Journal of Beijing University of Posts and Telecommunications, 2009, 32(1): 9-13.
[4] 周辉仁, 郑丕谔, 牛犇, 等. 基于递阶遗传算法和BP网络的模式分类方法[J]. 系统仿真学报, 2009, 29(8): 2243-2247.
ZHOU Hui-ren, ZHENG Pi-e, NIU Ben, et al. HGA-BP-based pattern classification method[J]. Journal of System Simulation, 2009, 29(8): 2243-2247.
[5] Kamarthi S V, Pittner S. Accelerating neural network training using weight extrapolations[J]. Neural Networks, 1999, 12(9): 1285-1299.
[6] Martin F, Moller S. A scaled conjugate gradient algorithm for fast supervised learning[J]. Neural Networks, 1993, 6(3): 525-533.
[7] Yu C C, Liu B D. A backpropagation algorithm with adaptive learning rate and momentum coefficient[C]//Proceedings of the 2002 International Joint Conference on Neural Networks (IJCNN’02). Honolulu, 2002: 1218-1223.
[8] WANG Wei, YU Bo.Text categorization based on combination of modified back propagation neural network and latent semantic analysis[J]. Neural Computing and Applications, 2009, 18(8): 875-881.
[9] WU Wei, SHAO Hong-mei, QU Di. Strong convergence for gradient methods for BP networks training[C]//ZHAO Ming-sheng, SHI Zhong-zhi. Proceedings of 2005 International Conference on Neural Networks and Brains. Beijing: IEEE Press, 2005: 332-334.
[10] MAN Zhi-hong, WU Hong-ren, LIU S, et al. A new adaptive backpropagation algorithm based on Lyapunov stability theory for neural networks[J]. IEEE Trans on Neural Networks, 2006, 17(6): 1580-1591.
[11] Wong W K, Yuan C W M, Fan D D. Stitching defect detection and classification using wavelet transform and BP neural network[J]. Expert Systems with Applications, 2009, 36: 3845-3856.
[12] 刘勍, 许录平, 马义德, 等. 基于脉冲耦合神经网络的图像NMI特征提取及检索方法[J]. 自动化学报, 2010, 7(4): 931-938.
LIU Qing, XU Lu-ping, MA Yi-de, et al. Image NMI feature extraction and retrieval method based on pulse coupled neural networks[J]. Acta Automatica Sinica, 2010, 7(4): 931-938.
(编辑 杨幼平)
收稿日期:2010-02-06;修回日期:2010-07-10
基金项目:国家科技重大专项(2009ZX03004-001)
通信作者:张国翊(1982-),男,湖南长沙人,博士研究生,从事无线泛在网络、认知无线网络、移动互联网服务与应用和模式识别研究;电话:13426083917;E-mail: zgysam@gmail.com