分布式多分类支持向量机
郭一楠1,程健1, 2,肖大伟 1,杨梅 1
(1. 中国矿业大学 信息与电气工程学院, 江苏 徐州,221116;2. 清华大学 自动化系, 北京,100084)
摘要:实际中存在大量基于多分类的大样本数据集的问题。针对单一SVM解决该类问题时计算时间长、占用内存大等缺点,提出了一种分布式多分类支持向量机模型,并针对其中的子多分类SVM模型的参数选择问题,采用遗传算法来寻找具有最优性能的超参数组合,即通过最小输出编码方式,将解决多分类问题的多个二分类支持向量机整合形成单个多分类支持向量机,并把多分类支持向量机的分类正确率作为遗传算法的适应度函数,所得超参数对应的子多分类SVM模型具有较好的泛化性能。最后将分布式多分类支持向量机模型用于手写数字识别中,通过对仿真结果的对比和分析,表明该模型具有较高的精度,提高了模型的泛化能力。
关键词:分布式支持向量机;多分类;遗传算法;超参数
中图分类号:TP301 文献标志码:A 文章编号:1672-7207(2011)S1-0829-06
Distributed multi-classification support vector machines
GUO Yi-Nan1, CHENG Jian1, 2, XIAO Da-Wei1, YANG Mei1
(1. College of Information and Electronic Engineering,
China University of Mining and Technology, Xuzhou 221116, China;
2. Department of Automation, Tsinghua University, Beijing 100084, China)
Abstract: Large samples problems based on multi-classification exist in practical. Aiming at the problem of higher computational complexity and larger computer memory by single support vector machines when dealing with such problem, a distributed multi-classification support vector machines model was established and genetic algorithm was adopted in selection of multi-classification support vector machines models’ hyper-parameters. Some binary classification support vector machines were made as a multi-classification support vector machines through minimum output code method and its classification accuracy rate was used for fitness function of genetic algorithm. Support vector machines with optimal hyper-parameters can ensure generalization. Taking the dataset of Handwritten Digit Recognition as an example, comparison and analysis show that the distributed support vector machines has higher accuracy rate and better generalization.
Key words: distributed support vector machines; multi-classification; genetic algorithm; hyper-parameters
统计学习理论研究有限样本情况下的机器学习问题。支持向量机(Support vector machine, SVM)的理论基础就是统计学习理论,其具有良好的泛化能力。但在实际问题中,基于多分类的大样本数据集普遍存在,例如基因数据集、文本数据、图像数据等。利用统计学习理论对这些大规模样本集学习可以得到更高的准确率并避免过拟合的现象;但是,当训练集规模较大时,SVM在学习过程中寻优速度非常缓慢,而且占用了大量的计算机内存。此外,单一的SVM还会由于噪声存在而导致寻优模型和输入空间不匹配。因此,当训练样本集很大时,研究者[1-3]提出了大量的快速迭代算法以降低训练支持向量机的代价。
集成学习利用单个模型之间的差异,将不同的单个模型组合成一个模型以提高模型的泛化能力。目前,基于数据集的集成方法和基于特征集的集成方法是集成学习领域的研究热点。基于数据集的集成方法使用采样技术获取训练数据,典型的方法有Bagging[4]和Adaboosting[5]。基于特征集的集成方法是通过提取不同的特征子集来训练集成中的个体,其关键内容是划分特征集的方法和对个体结论的融合方法。Ali[6]提出一种基于网格的分布式支持向量机,提供了一种支持分散和并行数据分析的计算和管理数据结构,但缺少对算法效率的分析。Effrosyni等[7]结合传感器网络,将分布式支持向量用于手写数字识别和人脸检测中。但已有文献缺少针对每个特征子集下多分类支持向量机模型的超参数选择,不能保证模型的泛化能力。鉴于此,本文提出一种基于模糊c均值的分布式多分类支持向量机建模方法,即首先通过模糊c均值算法对样本集聚类,分别用不同的子多分类支持向量机模型描述不同类别的子样本集,并采用遗传算法对每个子多分类SVM进行参数选择,保证每个子多分类SVM模型的泛化能力,然后利用一种综合算法将各个子模型的输出整合。
1 分布式多分类支持向量机模型
1.1 模型结构
对大规模样本数据集的核机器建模问题,可根据多模型的思想以及“分而治之”的原则,将输入样本数据集按照一定的原则划分为若干个子集,然后,分别在各个子集上建立核机器子模型,各个子模型的输出再通过一定的算法综合得到整个模型的输出。
可见,分布式多分类支持向量机模型构造的主要内容在于样本空间的划分、多分类支持向量机的实现和综合算法的建立。多分类支持向量机是将多分类问题转化为多个二分类问题来解决,即利用多个二分类支持向量机组合得到一个多分类支持向量机。
1.1.1 聚类算法
采用模糊C均值算法[9](Fuzzy C-means,FCM)对样本数据集进行划分。其本质是基于不同的特征数据集来划分,更强调对同一训练数据集的不同特征表示。给定数据集
,n为样本数。FCM 算法就是求使聚类目标函数最小化的模糊划分矩阵U和聚类中心V。设U={uij}是一个n×c矩阵,V=[v1, v2, …, vc]是一个s ×c矩阵。其中:uij是第i个样本属于第j个聚类中心的隶属度;c 是聚类中心数。
(1)
(2)
(3)
FCM的目标函数:
(4)
式中:
是加权指数;
是样本点xi和聚类中心vk的欧式距离。聚类中心数c和
的选择是通过数次仿真实验得到的。假设原始样本已知的样本类别数为C,则聚类中心数c和已知样本类别数C之间满足c≤C。这是因为,虽然子集划分越多模型性能越好,但同时也增加了模型的计算时间。另外,也可以保证每个子集中都能被分配到样本。
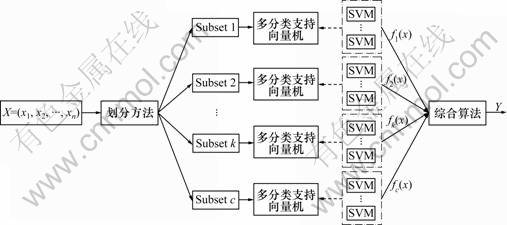
图1 分布式多分类支持向量机模型
Fig.1 Model of distributed multi-classification support vector machines
1.1.2 综合算法
分布式多支持向量机中的综合算法作用与组合策略相同。给定样本数据集(xi, yi), xi
RN, yi
R, (i=1, …, l),划分为c个子集Sk(k=1, …, c)。每个子集的聚类中心为ck,并分别训练构建了c个子模型SVMk,新的样本x对每个子模型的隶属度为{μ1, μ2, …, μc},则整个模型的输出为
(5)
式中:fk(x)为第k个子模型SVMk的输出。隶属度可按如下策略[10]求得:输入x距某一类中心越近,说明其属于该类的可能性越大,因此,它对该类的隶属度也越大。设
,则
(6)
1.2 子支持向量机原理及其超参数选择方法
1.2.1 子支持向量机原理
设样本xi及其所属类别yi表示为(xi, yi), xi
RN, yi
{1, -1}(i=1, …, l)。线性不可分支持向量分类可以归纳为如下的二次规划问题:
(7)
式中:ξi为松弛变量,以使非线性分类问题误分数尽可能小。如果ξi足够大,样本点就总可以满足式(7)的约束条件,需要对其进行限制。为此,在目标函数中设置一个惩罚变量γ>0,其取值越大,对经验误差惩罚越大。目的是在确定的样本集中调节学习机器置信范围和经验风险的比例,以使学习机器的推广能力最好。每个数据子集至少存在一个合适的γ,使得支持向量分类机的推广能力最强。上式对任意的正整数q都是凸规划问题;当q=1, 2时都是二次规划问题。引入松弛变量后,原规划的Wolfe对偶规划变为:
(8)
假定此时的最优解为α*,则有:(1)若α*=0,表示对应的样本在间隔带以外的正确划分区被正确划分,这些样本点对构建最优分类面没有贡献,不是支持向量。(2)若0<α*<γ,表示对应的样本点在间隔带的边界上,对构建最优分类面有直接贡献是支持向量。(3)若α*=γ,表示对应的样本点不在间隔带的边界上,而是在间隔带内,是被误分的样本点,但其是支持向量,对构建最优分类面有贡献。
对于非线性问题,可通过一个变换f:RN→F映射到一个高维特征空间F,在新空间中寻找最优分类面。寻优目标式和分类函数式都只涉及训练样本之间的内积运算xixj,可以用原空间的核函数来实现。只要核函数k(xixj)满足Mercer条件[11],就对应某一变换空间中的内积。因此,在最优分类面中用适当k(xixj),就可以实现从低维空间向高维空间的映射,实现某一非线性变换后的线性分类,且不增加计算复杂度。相应的寻优目标函数式变为
(9)
假设(w*, b*)是q=1时式(7)的解。对偶问题式(9)的解为
,则
。即最优分类面的权系数向量是训练样本中支持向量的 线性组合。相应的分类函数转化为f(x)=
。本文中核函数采用高斯核函数
。因此,影响SVM性能的超参数包括高斯核参数σ和规则化参数γ。
1.2.2 多分类问题解决方法
本文采用最小输出编码方法[12]将多分类问题转化为二分类问题。该方法建立在二进制数基础上,使得对某一多分类问题进行分类时,使所需的二分类器数目最少,并且固定二分类器的顺序。针对M类问题进行分类时,构造一个矩阵A,其行数为M1,列数为M2,列数为能表示所有类别的最小二进制数的位数。依次把数0, …, M1-1写成二进制的形式,空位或者0都写成-1。把矩阵A转置得到矩阵B,则B的行数M2就是所需二分类器的数目。分类原则就是按照每行元素的正负来进行,1代表正类,-1代表负类。
以10类分类问题为例,10类分类问题只需要4个二分类器即可构造分类编码矩阵。如式(10)所示,第1个二分类器以8和9为正类,第2个分类器以4,5,6和7为正类,第3个分类器以2,3,6和7为正类,第4个分类器以1,3,5,7和9为正类。对于一个新的输入进行判别时所使用的编码矩阵就是矩阵A。如果要测试一个输入x的归属,需要首先得到4个决策函数,即得到一个元素为-1和1的长度为4的数列,然后,将这个数列与矩阵A比较,有且仅有一行与所得数列相同,这个行就是输入x的归属类。通过解码过程就可知x的标签值。
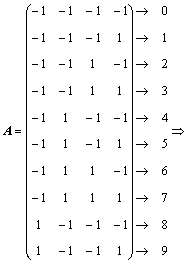
(10)
1.2.3 基于遗传算法的子支持向量机超参数选择方法
每个子支持向量机的性能影响着整个分布式支持向量机的性能,因此,子支持向量机的参数选择很有必要。已知多分类问题的样本类别数为C,则根据最小输出编码方法原理,其需要的二分类器数为能表示C的最小二进制数位数
,即分布式多分类问题需要
个二分类支持向量机。在实际参数优化中,为减少计算量,不需要对每个子二分类支持向量机的超参数都进行选择,而是通过最小输出编码方法的编码和解码过程把
个二分类机作为一个整体,形成一个多分类支持向量机。并把多分类支持向量机的分类正确率作为遗传算法的适应度函数,优化得到的超参数组合也由每个子集下
个二分类支持向量机共用。
(1) 训练集和测试集。根据多分类支持向量机最小输出编码方式原理,为使支持向量机能够在训练时就遍历样本中的所有类别,同时保证训练和测试的正确率,应使训练样本类别数
大于测试样本类别数
,即
。训练集只要涵盖已知所有类别数即可。对于手写数字识别问题,待分类的手写图像数据信息量很大,所以分布式多分类支持向量机中只需对测试样本进行样本空间划分而不需对训练样本进行划分。
(2) 优化问题描述。分布式多分类支持向量机中每个子支持向量机需要选择的超参数为σ和γ。本文采用遗传算法寻找具有最优性能的超参数组合。根据仿真优化问题模型,直接利用子多分类支持向量机分类后的结果,通过计算分类正确率来表示个体的适应度。设m为种群规模。AR(Accuracy Rate)是每一个子多分类SVM模型的性能评价指标:
(11)
(12)
式中:yi为实际值,
为模型预测输出;lk为第k个子集里的样本数,且
;
表示分类结果,分类正确为1,反之为0。
(3) 遗传算法中的进化操作。遗传算法中,进化操作核心考虑个体编码方式、选择算子、交叉算子和变异算子等。每种算子都根据适用场合不同存在多种形式。根据本文优化问题需要,选取进化算子具体如下。
① 个体编码方式。个体编码方式直接影响其它进化算子的选取。本文需要优化的变量为σ和γ,二者均为实数。为减少实数与二进制数之间转换产生的误差,选取实数编码描述个体,记为x=(γ, σ)。
② 选择算子。选择算子根据个体适应度,按照某种准则从当前种群中获得下一代个体。本文采用随机联赛选择和最优个体保留方法相结合的选择算子,具体操作如下。
Step 1:最优个体保留策略。选出当前种群中适应度最高的个体和适应度最低的个体。若当前种群最优个体的适应度大于已保留的最优个体适应度,则用当前种群的最优个体作为新的保留最优个体,并用保留最优个体替代当前种群中的最差个体。
Step 2:联赛选择。从种群中随机选取g(g=2)个个体进行适应度的比较,将适应度最大的个体遗传到下一代种群中。重复该过程m次,得到下一代种群的m个个体。
③ 交叉算子。采用适合于实数编码的算术交叉算子。假设2个配对个体为xa(t)和xb(t),h为[0,1]之间的一个常数,以交叉概率Pc经算术交叉后,2个个体线性组合生成2个新个体。
(13)
④ 变异算子。选取适用于实数编码的非均匀变异算子。以变异概率Pm对随机指定的某一位取值作非均匀变异运算。若x=(γ, σ)经变异后为x′=(γ′, σ),且变异点γ处的取值范围为[γmin, γmax],则有
(14)
式中:Δ(t, e) (e代表γmax-γ和γ-γmin)表示[0, e]范围内符合非均匀分布的随机数。
综上所述,分布式多分类支持向量机的步骤如下。
Step 1:利用模糊c均值算法,通过数次仿真实验,使用适当的加权指数
和初始聚类数c。把测试样本数据集划分为c个子集,并得到相应的聚类中心。
Step 2:通过训练集,并利用遗传算法,分别确定c个测试子集下的c个多分类SVM(即
个二分类机形成的一个多分类支持向量机)所对应的超参数(γi, σi), i=1, …, c。对于每个子集,最适当的SVM模型即为ARsub-SVM最大。
Step 3:对于新的输入数据x,根据式(6)在第k个子模型上得到输出Yk(k=1, 2, …, c)和隶属度μk(k=1, 2, …, c)。
Step 4:根据式(5)将各个子多分类SVM模型的结果合成即是最终结果。
2 手写数字识别
为了验证分布式多分类支持向量机在大规模样本训练问题上的性能,针对手写数字识别问题,采用USPS标准数据库[13]进行仿真实验。该数据库一共有10 类样本(分别为手写数字“0, 1, 2, 3, 4, 5, 6, 7, 8, 9”),其为灰度图像像素值(16*16)。本实验选取训练集和测试集样本数均为7 291,并统计分类正确率,其中每类的样本个数如表1所示。
手写数字识别的样本个数及样本维数较大。通过多次仿真实验,选取加权指数
=10,初始聚类数c=10。采用模糊c均值聚类后每个子样本集内的样本规模如表2所示。可见,对于手写字体识别这类大样本问题,模糊c均值只是用来划分样本空间,并不能保证每个子集中只对应一类数据。
表1 测试样本每类中的样本数
Table 1 Number of testing samples for each class
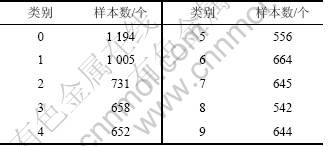
表2 聚类后每个子样本集内的样本规模
Table 2 Number of samples for each subset
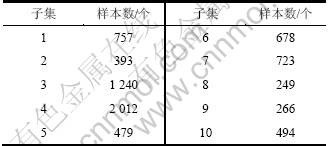
根据问题实际需求,每个子集下多分类支持向量机中超参数σ和γ的变化区域为:σ搜索范围[0.1, 10];γ搜索范围[0.1, 100]。遗传算法的主要参数取值:种群规模m=20;进化终止代数=50;解精度=0.000 1;交叉概率Pc=0.6;变异概率Pm=0.03。每个子模型用遗传算法优化得到的σ、γ以及和其他算法的比较结果如表3所示。表中,AvrAR表示AR的平均值。
可见:没有经过子支持向量机超参数选择的分布式支持向量机的正确率要小于有超参数选择的支持向量机的正确率,所以,子支持向量机的超参数选择是很有必要的。相对于基于样本集重新采样的Bagging和Adaboosting,虽然子集4和子集7的正确率小于Bagging算法的正确率,子集4至子集8的正确率小于Adaboosting算法的正确率,但是10个子集的正确率平均值均大于Bagging和Adaboosting算法的正确率。由于分布式支持向量机是根据多模型的思想以及“分而治之”的原则建立的,所以,在处理手写数字识别这类大样本问题时,它将样本集划分为10个子集;在10个子支持向量机上并行处理,计算时间比单个支持向量机的要少。相对于基于数据集划分的集成算法Bagging和Adaboosting来说,分布式支持向量机运行时间相对较长,但正确率高,模型泛化能力强。
表3 各种方法仿真结果对比
Table 3 Results of different methods
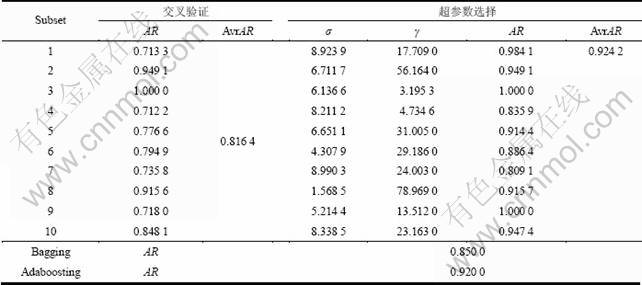
3 结论
单一SVM训练大样本数据集,计算时间长、占用内存大,而分布式支持向量机根据多模型的思想以及“分而治之”的原则,将输入样本集按照一定的原则划分为若干个子集,然后,分别在各个子集上建立支持向量机子模型,各个子模型的输出再通过算法综合得到整个模型的输出。本文针对手写数字识别问题,提出了一种分布式多分类支持向量机模型;并对子多分类支持向量机模型中参数的选择问题,采用遗传算法来寻找具有最优性能的超参数组合,通过和基于数据集重采样的集成算法Bagging与Adaboosting进行比较,该模型具有较高的正确率和较强的泛化能力。
参考文献:
[1] Boser B E, Guyon I M, Vapnik V N. A training algorithm for optimal margin classifiers[C]//Proceedings of the 5th Annual ACM Workshop on Computational Learning Theory. ACM New York, Pittsburgh, PA, USA, 1992: 144-152.
[2] Dong J X, Krzyzak J T, Suen C Y. Fast SVM training on very large datasets[J]. Journal of Machine Learning Research, 2005, 6: 363-392.
[3] Mangasarian O L. A finite Newton method for classification[J]. Optimization Methods and Software, 2002, 17(5): 913-929.
[4] BREIMAN L. Bagging predictors[J]. Machine Learning, 1996, 24(2): 123-140.
[5] Schapire R E. The strenght of weak learnability[J]. Machine Learning, 1990, 5(2): 197-227.
[6] Ali M, Manar A K. A grid-based distributed SVM data mining algorithm[J]. European Journal of Scientific Research, 2009, 27(3): 313-321.
[7] Effrosyni K, Pascal F. Distributed SVM applied to image classification[C]//Proceedings of IEEE International Conference on Multimedia and Expo. Toronto, Ontario, Canada: IEEE Press, 2006: 1-4.
[8] Cheng J, Qian J S, Guo Y N. A distributed support vector machines architecture for chaotic time series prediction[J]. Lecture Notes in Computer Science, 2007, 4922: 892-899.
[9] Dunn J C. Some recent investigations of a new fuzzy partition algorithm and its applications to pattern classification problems [J]. Cybernetics and Systems, 1974, 4(2): 1-15.
[10] 桂卫华, 李勇刚, 阳春华, 等, 基于改进聚类算法的分布式SVM及其应用[J]. 控制与决策, 2004, 19(8): 852-856.
GUI Wei-hua, LI Yong-gang, YANG Chun-hua, et al. Distributed SVM based on improved clustering algorithm and its application[J]. Control and Decision, 2004, 19(8): 852-856.
[11] Scholkopf B, Mika S, Burges C, et al. Input space versus feature space in kernel-based methods[J]. IEEE Transactions on Neural Networks, 1999(5): 1000-1017.
[12] Van Gestel T, Suykens J. Lambrechts. Multiclass LSSVMs: moderated outputs and coding decoding schemes[J]. Neural Processing Letters, 2002, 15: 45-48.
[13] Holland S, Richard N. Classification of handwritten characters by their symmetry features[C]//Proceedings of International Conference on Advances in Computing, Control, and Telecommunication Technologies. Trivandrum, Kerala, India: IEEE Computer Society, 2009: 316-318.
(编辑 袁赛前)
收稿日期:2011-04-15;修回日期:2011-06-15
基金项目:国家自然科学基金资助项目(60805025);江苏省自然科学基金资助项目(BK2010183);中国博士后基金资助项目(20090460328);江苏省青蓝工程
通信作者:郭一楠(1975-),女,山西太原人,教授,博士,从事智能优化算法与智能控制研究;电话:13852000540; E-mail: nanfly@126.com