DOI: 10.11817/j.issn.1672-7207.2015.02.017
基于EMD与多重分形去趋势法的轴承智能诊断方法
贾峰1,武兵1, 2,熊晓燕1, 2,熊诗波1, 2
(1. 太原理工大学 机械电子工程研究所,山西 太原,030024;
2. 太原理工大学 新型传感器与智能控制教育部重点实验室,山西 太原,030024)
摘要:引入经验模态分解(EMD)方法去除故障信号的趋势项,提出EMD-MFDFA(multifractal detrended fluctuation analysis)的多重分形分析方法,并通过仿真分析EMD方法去趋势效果的有效性。然后将采用EMD-MFDFA方法提取的电机滚动轴承振动信号的多重分形特征向量作为训练集,利用混合遗传算法搜索全局最优的能力优化支持向量机(SVM)模型参数,建立电机滚动轴承的智能诊断模型。最后,通过对电机滚动轴承不同状态的振动信号进行分析。研究结果表明:EMD-MFDFA方法能很好地揭示滚动轴承的振动信号多重分形特性,对滚动轴承正常状态、单一故障与多故障耦合等状态具有很强的辨识能力;所建立的智能诊断模型可以有效地诊断滚动轴承不同的故障状态,能够作为滚动轴承故障在线监测的有效工具。
关键词:多重分形;去趋势波分析;经验模态分解;遗传算法;支持向量机
中图分类号:TH165.3 TH17 文献标志码:A 文章编号:1672-7207(2015)02-0491-07
Intelligent diagnosis of bearing based on EMD and multifractal detrended fluctuation analysis
JIA Feng1, WU Bing1, 2, XIONG Xiaoyan1, 2, XIONG Shibo1, 2
(1. Research Institute of Mechatronics Engineering, Taiyuan University of Technology, Taiyuan 030024, China;
2. Key Laboratory of Advanced Transducers and Intelligent Control Systems of Ministry of Education,
Taiyuan University of Technology, Taiyuan 030024, China)
Abstract: The empirical mode decomposition (EMD) method was introduced to remove the fault signal local trends. A modified multifractal analysis method, i.e EMD-MFDFA (multifractal detrended fluctuation analysis), was proposed and the performance of the EMD-MFDFA method was proved by simulation. Then taking the multifractal feature vectors of motor bearing vibration signals extracted by the EMD-MFDFA method as the training sets, an intelligent diagnosis model was established to diagnose motor bearing early faults according to the support vector machine (SVM) theory, in which the parameters of SVM were optimized by using the hybrid genetic algorithm. Finally, the different states of the motor bearing vibration signals were analyzed. The results show that the EMD-MFDFA method can reveal the multifractal characteristics of the motor bearing signals and identify the bearing normal state, a single fault state and multiple faults state accurately. And the established model is able to diagnose bearing different fault effectively and suitable for on-line monitoring for motor bearing faults.
Key words: multifractal; detrended fluctuation analysis; empirical mode decomposition; genetic algorithm; support vector machine
电机滚动轴承运行状态往往直接影响整个系统的性能,因而研究其滚动轴承的故障状态监测诊断技术具有重要价值。电机滚动轴承的振动信号是包含着多种频率成分的不规则信号,但是采集到的电机滚动轴承的振动信号的时域波形和变化趋势会存在明显的相似性即分形性质[1]。利用多重分形方法能精细地刻画分形信号的局部结构,而且多重分形谱能够很好地描述信号的结构特征和局部尺度行为[2],因而,可以将多重分形理论引入电机滚动轴承的故障诊断中。随着多重分形理论的发展,Kantelhardt等[3]于2002年提出一种基于去趋势波动分析(DFA)方法的多重分形分析方法——多重分形去趋势法(MFDFA)。该方法利用DFA消除序列趋势项的能力解决了标准多重分形分析处理非平稳序列的欠缺问题,对非平稳序列的处理更有效[4]。而且对平稳时间序列,用去趋势多重分形方法得到的结果与用标准方法得到的结果相同;而对于非平稳的序列,其计算效果与其他方法相当[5]。因此,多重分形去趋势法是一种更加实用的多重分形分析方法,已被应用于多个研究领域,取得了较好的分析效果。文献[6]将MFDFA引入机械故障诊断领域,给出了DFA与MFDFA方法在分析机械故障信号上的优缺点,表明MFDFA能够更有效地揭示隐藏在多标度非平稳信号中的动力学行为。文献[7]利用MFDFA分析了滚动轴承的故障信号,利用实验数据表明多重分形谱可以有效表征轴承故障信号的内在动力学机制,适合于轴承的故障特征提取。文献[8]在利用MFDFA提取轴承振动信号的多重分形特征之后,使用支持向量机(SVM)建立诊断模型实现了故障诊断,但所建立的SVM模型只是单一特征的诊断模型,通用性较差。通常MFDFA方法假设信号的局部趋势是多项式的形式,在消除序列趋势项都是使用多项式拟合的方法,然而,机械振动信号的局部波动强烈且其局部趋势都不是多项式的形式,所以,使用多项式进行拟合,很难准确地拟合信号的趋势项。而经验模态分解(EMD)是一种自适应的信号分析方法,依据数据自身的时间尺度特征来进行信号分解,能使复杂信号分解为有限个本征模函数(IMF)及1个余项,所分解出来的各IMF分量包含了原信号不同时间尺度的局部特征信号[9],余项即为信号的趋势项。EMD方法理论上可以分解任何类型的信号,相对于多项式拟合,具有非常明显的优势。而且使用MFDFA方法提取的信号多重分形特征有明确的物理意义,可以结合机器学习算法建立智能故障诊断模型,实现对轴承故障的在线诊断。支持向量机(support vector machine, SVM)作为近些年兴起的机器学习方法,与其他方法相比,具有结构简单、学习速度快、全局最优、泛化性好等优点[10]。本文作者针对多项式不能合理拟合机械故障振动信号的趋势项这一问题,引入EMD方法分解故障信号的趋势项,提出了EMD-MFDFA的多重分形分析方法。然后利用EMD-MFDFA方法提取的电机滚动轴承多重分形特征,并结合混合遗传算法优化的SVM模型,建立电机滚动轴承的智能诊断模型。最后通过轴承的仿真信号及实验信号,证明所提出的EMD-MFDFA方法及智能诊断模型的有效性。
1 理论基础
1.1 MFDFA算法
对于时间序列xk(k=1,2,…,N),设xk序列是紧支集,MFDFA过程如下[4]。
1) 构造信号对于均值的累积离差Y(i)为
(1)
式中:i=1,2,…,N;
为xk序列的均值。
2) 将信号Y(i)以尺度s划分为互不重叠的Ns段数据:
(2)
3) 利用最小二乘法拟合每段数据,设yv(j)为第v段数据的r阶拟合多项式,r为拟合多项式的阶数,拟合的阶数反映了数据去趋势的效果,阶数r越高,去趋势的效果越好。然后,求取原v段数据与yv(j)的均方误差F2(s,v):
(3)
式中:j=1,2,…,s;v=1,2,…,Ns。
4) 定义时间序列xk的第q阶波动函数为
(4)
当q=2时,MFDFA就退化为DFA。式(4)的物理意义为不同的q描述不同程度波动对Fq(s)的影响。
5) 当时间序列xk存在自相似特征,且
时,波动函数Fq(s)与尺度s之间存在稳定的幂律关系为
(5)
式中:h(q)为广义Hurst指数,其值可在波动函数Fq(s)与尺度s的对数关系图上估算出来。
在描述多重分形的奇异谱时,h(q)与基于配分函数方法中的标度指数τ(q)的关系为
(6)
再由统计物理中的legendre变换可以得到奇异指数α和多重分形谱f(α):
(7)
(8)
1.2 EMD方法
EMD方法是由美国NASA的黄锷博士提出的一种信号分析方法,它依据数据自身的时间尺度特征来进行信号分解,无需预先设定任何基函数,在处理非平稳及非线性数据上,具有非常明显的优势[9]。因而将其引入MFDFA中,去除信号积累离差的趋势项。其具体方法如下。
1) 找出信号x(t)的局部极值点。
2) 把所有的局部极大值用三次样条光滑地连接起来得到上包络线e+(t),同样地可以得到下包络线e-(t)。则可得到平均包络线:
(9)
3) 求出差值函数zi(t):
(10)
检查zi(t)是否满足IMF条件,若不是,则把zi(t)当作新的待处理量,重复做以上步骤,若zi(t)满足条件,则zi(t)就是第1个IMF,记作l1(t)。
4) 最后信号x(t)被分解为n个基本模式分量lj(t)和一个余项rn(t),即
(11)
余项rn(t)即为信号的趋势项。
1.3 基于EMD的MFDFA算法
由于机械振动信号是由三角函数的叠加与相互调制得到的,且具有非平稳的特性。使用多项式拟合这样的信号,很容易会导致数据过拟合与欠拟合的现象发生,而EMD方法在理论上可以应用于任何类型的信号的分解,在处理非平稳及非线性数据上,具有非常明显的优势。所以,本文改进了MFDFA算法即EMD-MFDFA算法:在MFDFA算法第(3)步使用EMD方法去除信号累积离差量Y(i)的趋势。
设rv(j)是第v段累积离差的EMD分解后所得的余项,则均方误差F2(s,v)为
(12)
1.4 信号仿真
使用仿真的轴承故障振动信号进行仿真实验。仿真信号的表达式为
(13)
式中:f=900 Hz为固有频率,g=0.1为阻尼系数,rand[-1,1]表示在[-1,1]内的随机数。采样频率为20 kHz,采样后数据x1的数量为5 120点,如图1所示。
根据式(1)可得信号x1的累积离差Y1,如图2所示。以图2可以看到:累积离差Y1局部波动很大,若使用多项式拟合Y1,则很容易出现过拟合及欠拟合的现象。
对Y1进行MFDFA及EMD-MFDFA分析,由式(5)可知,在同一q阶波动下,lg2[Fq(s)]与lg2s的关系为线性关系,即符合同一标度不变性原理。图3所示为Fq(s)与s的对数图上,在各q阶波动下,lg2[Fq(s)]与拟合直线的残差的均方根值(RMS)。从图3可以看出:使用EMD方法去趋势后,Fq(s)∝sh(q)的幂律关系更稳定,从而优化了所提取的多重分形的谱特征。
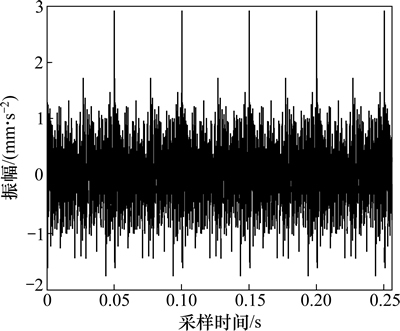
图1 轴承故障仿真信号
Fig. 1 Simulation signal of bearing fault
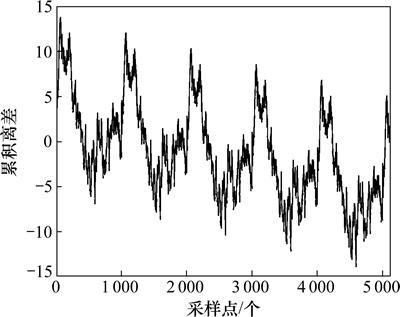
图2 仿真信号的累积离差
Fig. 2 Cumulative deviation of simulated signal
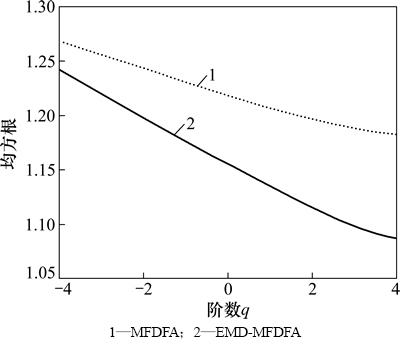
图3 lg2[Fq(s)]及其拟合直线的残差的均方根
Fig. 3 RMS of residuals of lg2[Fq(s)] and its fitted line
2 EMD-MFDFA与SVM结合的智能诊断方法
2.1 EMD-MFDFA的特征提取方法
对信号进行EMD-MFDFA分析后,奇异指数α反映了分形序列在某局部概率测试分布上的不均匀程度,奇异谱f(α)是奇异指数α的分维函数。由α与f(α)可得到描述多重分析谱的一些重要参数,这些参数能精细地表达多重分析时间序列动力学行为[6, 11]:1) 多重分形谱宽
,Δα表示了信号多重分形性的强弱,可以反映振动信号波动的剧烈程度;2) 最大最小概率子集维数差别
,Δf反映了概率测度所构成的子集中最大、最小概率处元素数量的比例,利用Δf可以以度量振动信号中波动平稳与波动剧烈峰值数目的比例;3) 最小奇异指数αmin,αmin越小说明信号局部奇异性越强,即信号局部变化越剧烈,因而αmin在一定程度上也反映了振动信号的局部变化剧烈程度;4) 多重分形谱fmax(α)对应的奇异值α0描述了信号的不规则性。
根据以上分析,在得到信号多重分形谱后,定义如下特征向量以提取多重分形特征:
(14)
2.2 建立智能诊断模型
支持向量机是建立在统计学习理论基础上的一种新算法。自SVM出现以来,在故障诊断方面的应用越来越广泛。SVM首先通过非线性变换将原始的样本空间映射到高维的特征空间,然后在这个新空间中求取最优线性分类面,进而对数据进行分类。而这种非线性变换通过定义适当的内积函数实现[10]。
在得到信号多重分形特征向量H后,将其作为SVM的输入,使用高斯径向基作为SVM的核函数,从而实现轴承故障的智能诊断。由于惩罚因子C与高斯核宽度σ是建立SVM智能诊断模型的重要参数,其取值决定着模型的诊断精度,本文使用遗传算法对参数进行优化[12]。尽管标准遗传算法有很多优点,但算法易陷入局部最优,而混合遗传算法可以跳出局部最优解,尽可能找到全局最优解[13]。因而本文引入混合遗传算法来确定C与σ的最优值,所使用的混合遗传算法的流程图见图4。
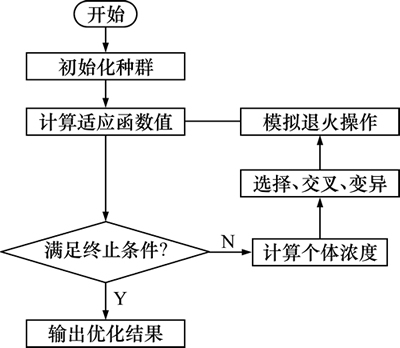
图4 混合遗传算法流程图
Fig. 4 Flow chart of hybrid genetic algorithm
由上述分析,根据EMD-MFDFA与SVM原理,可将计算所得的H作为SVM的输入,建立智能诊断模型,诊断电机滚动轴承的故障。基于EMD-MFDFA的智能诊断模型的流程图如图5所示。
3 实验结果与分析
滚动轴承的故障振动信号是在Y160M2-8型电机轴承故障实验台上采集的。实验是模仿实际工况进行的,电机轴承内圈与电机轴过盈配合,与轴无相对运动;外圈与电机端盖配合,起支撑作用。由于轴承在电机内部,不能直接测量,但轴承振动直接传递到端盖,这里将传感器安装在电机端盖上,实现间接测量。滚动轴承型号为6309,加载器电流为0.5 A,采样频率为10 kHz。实验数据包含正常、滚动体故障、内圈故障、滚动体与内圈的组合故障这4种故障状态。由于垂直地面方向的振动信号包含信息最丰富,因而进行分析时选择了传感器的y轴方向所采集到的信号,采集时间为21 s。图6所示为电机轴承故障实验台示意图,图中传感器为压电式加速度传感器,信号采集装置为DEWETRON数据采集仪(型号为DEWE-2010)。所采集的4种故障振动信号如图7所示。
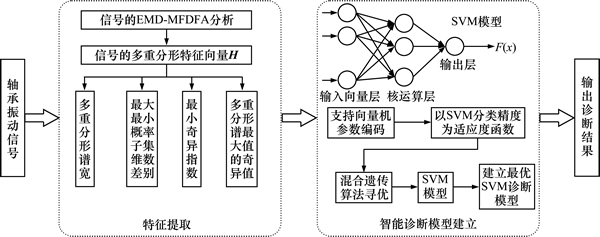
图5 基于EMD-MFDFA的智能诊断模型的流程图
Fig. 5 Flow chart of intelligent diagnostic model based on EMD-MFDFA
选择每类故障状态振动信号的前5 120个数据进行EMD-MFDFA分析,参数s最小值为64,最大值为1 024,取值间隔为48;参数q最小值为-5,最大值5,取值间隔为0.1[14]。EMD方法的停机准则见参考文献[15]。分析结果见图8和图9。
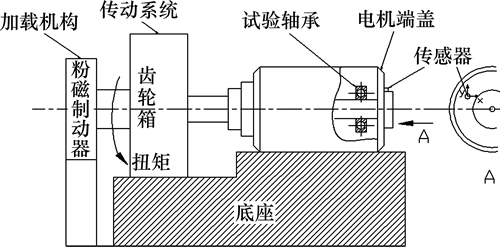
图6 电机轴承故障模拟实验台示意图
Fig. 6 Schematic diagram of motor bearing fault laboratory bench
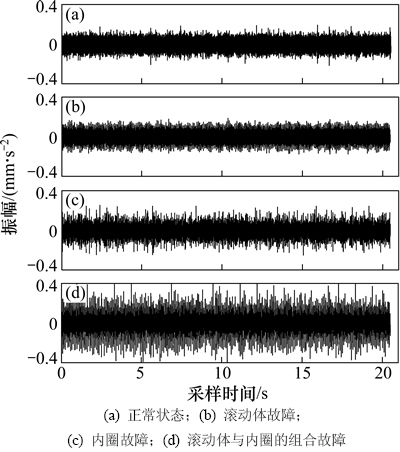
图7 4种电机轴承振动信号
Fig. 7 Four types of motor bearing vibration data
图8所示为4种轴承振动信号的q与广义Hurst指数关系。从图8可以看到:4种信号的广义Hurst指数都是关于q的曲线[14],说明这4种轴承状态的振动信号都存在着多重分形的特性,从而导致振动信号出现多标度分形的特性。图9所示为4种信号的多重分形奇异谱。从图9可以看到:4种信号奇异谱具有不同的形状、位置和谱宽,正是因为4种信号具有不同的多重分形特性,导致其内在的动力学机制不同,产生了谱差异。根据奇异谱的特征,可以提取信号的多重分形特性,从而进行有效的故障诊断。
由以上分析,将每种振动信号分为40段,每段5120个数据点,分别使用EMD-MFDFA方法提取特征。然后与SVM原理结合,将所提取的多重分形特征向量作为SVM的输入,进行电机轴承的智能诊断。
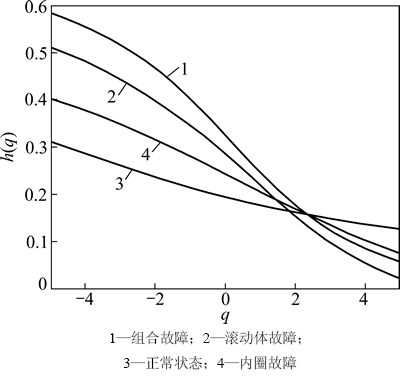
图8 4种电机轴承振动信号的广义Hurst指数
Fig. 8 Generalized Hurst exponent curves of four types of motor bearing vibration signal
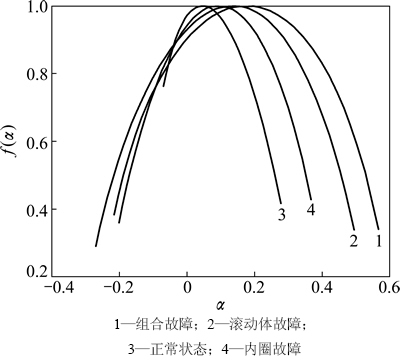
图9 4种电机轴承振动信号的多重分形奇异谱
Fig. 9 Multifractal singularity spectra of four types of motor bearing vibration signal
由于4种振动信号,每种信号有40个样本,可以提取160组多重分形特征向量H。将每种信号15组特征向量采用不同的方法建立不同SVM诊断模型,如表1所示。表1中:n1表示每种信号有n1组特征向量作为训练集,n2表示取每种信号有n2组特征向量作为测试集。
表1 SVM模型信息
Table 1 Information of SVM
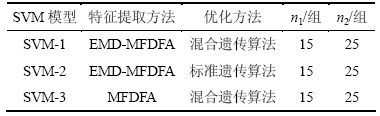
核函数选择高斯径向基核函数,然后对不同的SVM模型训练。图10所示为SVM-1模型使用混合遗传算法寻找最佳参数时适应度函数值的进化曲线。
3个SVM的模型参数C和γ的结果如表2所示。使用训练好的SVM模型,对测试集分类,结果如表3所示。从表3可见:SVM-1可以有效区分正常轴承、单一故障轴承与多故障轴承,证明了EMD-MFDFA方法的有效性。从SVM-1与SVM-2的对比可见:使用EMD-MFDFA提取特征方法所建立的智能诊断模型的诊断精度更高,也说明去趋势时使用EMD方法比使多项式法更适合于机械振动信号。从SVM-1与SVM-3的对比可见:在使用EMD-MFDFA方法建立智能诊断模型时,混合遗传算法相对于标准遗传算法能跳出局部极值点,收敛到全局最优,使SVM-1模型更适宜于在线智能诊断。
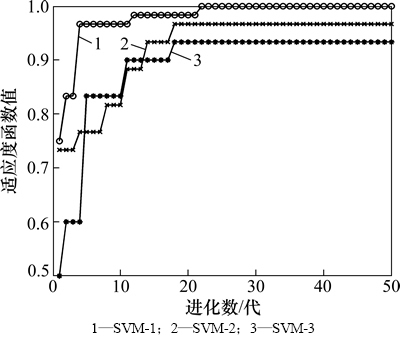
图10 遗传算法寻找SVM最优参数的适应度函数曲线
Fig. 10 Optimal parameters fitness function curve obtained by genetic algorithm
表2 SVM的参数值
Table 2 Parameter values of SVM
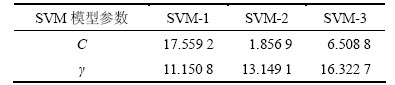
表3 测试集的具体分类情况
Table 3 Specific classification of test sets
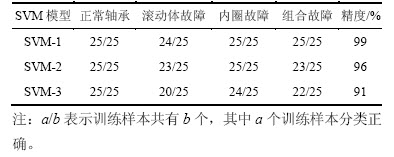
4 结论
1) 利用EMD方法消除滚动轴承时间序列趋势项,相比于多项式去趋势更符合滚动轴承振动信号非平稳的特性,去趋势结果更准确。
2) 使用EMD-MFDFA方法提取滚动轴承不同故障状态下振动信号的多重分形谱特征,与MFDFA方法相比,更能揭示滚动轴承多重分形特性,揭示不同状态信号不同的内在动力学机制。该方法可以有效区分滚动轴承正常状态、单一故障状态与多故障状态,适合作为滚动轴承的特性提取方法。
3) 多重分形特征向量与SVM结合建立的智能诊断模型,可以有效诊断滚动轴承不同的故障状态,使用混合遗传算法优化的模型可以提高模型的诊断精度,且该智能诊断模型计算方便,可以作为滚动轴承故障状态在线监测的工具。
参考文献:
[1] YANG Junyan, ZHANG Youyun, ZHU Yongsheng, et al. Intelligent fault diagnosis of rolling element bearing based on SVMs and fractal dimension[J]. Mechanical Systems and Signal Processing, 2007, 21(5): 2012-2024.
[2] Lopes R, Betrouni N. Fractal and multifractal analysis: A review[J]. Medical Image Analysis, 2009, 13(4): 634-649.
[3] Kantelhardt J W, Zschiegner S A, Koscielny-Bunde E, et al. Multifractal detrended fluctuation analysis of nonstationary time series[J]. Physica A: Statistical Mechanics and its Applications, 2002, 316(1/4): 87-114.
[4] Talebinejad M, Chan A D C, Miri A. Fatigue estimation using a novel multi-fractal detrended fluctuation analysis-based approach[J]. Journal of Electromyography and Kinesiology, 2010, 20(3): 433-439.
[5] Norouzzadeh P, Rahmani B. A multifractal detrended fluctuation description of Iranian rial-US dollar exchange rate[J]. Physica A: Statistical Mechanics and its Applications, 2006, 367: 328-336.
[6] 林近山, 陈前. 基于多重分形去趋势波动分析的齿轮箱故障特征提取方法[J]. 振动与冲击, 2013, 32(2): 97-101.
LIN Jinshan, CHEN Qian. Fault feature extraction of gearboxes based on multifractal detrended fluctuation analysis[J]. Journal of Vibration and Shock, 2013, 32(2): 97-101.
[7] LIN Jinshan, CHEN Qian. Fault diagnosis of rolling bearings based on multifractal detrended fluctuation analysis and Mahalanobis distance criterion[J]. Mechanical Systems and Signal Processing, 2013, 38(2): 515-533.
[8] 李兆飞, 柴毅, 李华锋. 多重分形去趋势波动分析的振动信号故障诊断[J]. 华中科技大学学报(自然科学版), 2012, 40(12): 5-9, 17.
LI Zhaofei, CHAI Yi, LI Huafeng. Diagnosing faults in vibration signals by multifractal detrended fluctuation analysis[J]. Journal of Huazhong University of Science and Technology (Nature Science), 2012, 40(12): 5-9, 17.
[9] 杨世锡, 胡劲松, 吴昭同, 等. 旋转机械振动信号基于EMD的希尔伯特变换和小波变换时频分析比较[J]. 中国电机工程学报, 2003, 23(6): 102-107.
YANG Shixi, HU Jingsong, WU Zhaotong, et al. The comparison of vibration signals’ time-frequency analysis between EMD-based HT and WT method in rotating machinery[J]. Proceedings of the CSEE, 2003, 23(6): 102-107.
[10] Sapankevych N I, Sankar R. Time series prediction using support vector machines: A survey[J]. IEEE Computational Intelligence Magazine, 2009, 4(2): 25-38.
[11] 赵玲. 旋转机械系统故障特征提取中的分形方法研究[D]. 重庆: 重庆大学机械工程学院, 2010.
ZHAO Ling. Research on fractal method of rotary mechanical fault feature extraction[D]. Chongqing: Chongqing University. College of Mechanical Engineering, 2010.
[12] Thomas M. Machine learning[M]. New York: McGraw Hill Higher Education, 1997: 249-273.
[13] WU Chihhung, KEN Yun, HUANG Tao. Patent classification system using a new hybrid genetic algorithm support vector machine[J]. Applied Soft Computing, 2010, 10(4): 1164-1177.
[14] Ihlen E A F. Introduction to multifractal detrended fluctuation analysis in Matlab[J]. Frontiers in Physiology, 2012, 141(3): 1-18.
[15] Rilling G, Flandrin P, Goncalvés P. On empirical mode decomposition and its algorithms[C]//Proc of IEEE EURASIP Workshop on Nonlinear Signal and Image Processing. [S.1.]: IEEE Press, 2003: 9-11.
(编辑 赵俊)
收稿日期:2014-03-10;修回日期:2014-06-22
基金项目(Foundation item):国家自然科学基金资助项目(51035007);山西省自然科学基金资助项目(2012011046-10)(Project (51035007) supported by the National Natural Science Foundation of China; Project (2012011046-10) supported by the Natural Science Foundation of Shanxi Province, China)
通信作者:武兵,博士,副教授,从事机电系统故障诊断、动态测试研究;E-mail:wubing@tyut.edu.cn