DOI: 10.11817/j.issn.1672-7207.2019.03.011
用于卷积神经网络图像预处理的目标中心化算法
董秋成1,吴爱国1,董娜1,冯伟2
(1. 天津大学 电气自动化与信息工程学院,天津,300072;
2. 中国科学院自动化所 模式识别国家重点实验室,北京,100000)
摘要:为解决工业生产中对不同零件进行自动分类的问题,提出一种基于卷积神经网络的模式识别算法,对29种不同尺寸的螺丝、螺母和垫片进行分类。首先采集待分类零件的图像数据,通过数据增强得到数据集,然后设计一种简化的卷积神经网络。提出一种对图像中的目标位置进行中心化的图像预处理算法,它能够提取图像中目标所在的区域并将其移动到图像中心位置。研究结果表明,与不采用目标中心化算法的传统方法相比,总体准确率从97.59%提升至99.96%,具有最低准确率的零件的准确率从85.83%提升至99.67%。使用卷积神经网络对背景纯净且目标明显的图像进行分类时,使用本文提出的目标中心化算法进行图像预处理能够显著提高网络的识别准确率。
关键词:零件;识别;卷积神经网络;数据增强;中心化;目标提取
中图分类号:TP 391.4 文献标志码:A 文章编号:1672-7207(2019)03-0579-08
Target-centralization algorithm used for image preprocessing of CNN
DONG Qiucheng1, WU Aiguo1, DONG Na1, FENG Wei2
(1. School of Electrical and Information Engineering, Tianjin University, Tianjin 300072, China;
2. National Laboratory of Pattern Recognition Institute of Automation, Chinese Academy of Sciences, Beijing 100000, China)
Abstract: To solve the problem of classifying different parts automatically in industrial production, a pattern recognition algorithm based on convolutional neural network was raised and 29 different sizes of screws, nuts and washers were classified. Firstly, image data of the parts that were going to be classified were collected, and the dataset was created by data augmentation. Then, a simplified convolutional neural network was designed. An image preprocessing algorithm to centralize the position of the target in the image was raised, which can extract the target area in the image and move it to the center of the image. The results show that compared with traditional method without target-centralization algorithm, the total error is raised from 97.69% to 99.96, and the accuracy of the part which has the lowest accuracy is raised from 85.83% to 99.67%. When convolutional neural network is used to classify images which has pure background and obvious object, using the target-centralization algorithm raised in this paper to preprocess the images can improve the accuracy of the network significantly.
Key words: parts; recognition; convolutional neural network; data augmentation; centralization; object extraction
随着工业4.0时代的到来,图像识别技术在工业生产中的应用越来越广泛,已被用于产品检测、计数、尺寸测量等许多方面。零件识别是许多工业生产流程中常见且必要的工作任务,它可以把不同种类的目标物体进行正确的分类处理,也可以用于分拣出质量不达标的工件。依靠人工进行零件识别,分类效率低、可靠性差、成本高。为了提高工业生产线自动化、智能化的程度,利用机器视觉设计出能够应用于实际生产的零件自动识别系统成为当前工业自动化领域的重要课题,具有重要的理论意义和实用价值[1-2]。现有的零件识别算法基本都是依赖传统机器学习的基本方式:先提取一个合适的特征集,再将这些特征提供给简单的机器学习算法[3-8]。这些算法的共同特点在于零件的特征均是人工选取的,或是由人工设计的算法进行提取的。然而,特征的选取不仅需要依靠经验,而且适用性往往受到抑制,可扩展性不佳。当需要识别的零件种类较多时,人工提取特征不仅工作量巨大,而且选取的特征不一定是最优的。HINTON等[9]提出了深度学习模型。与手工规则构造特征的方法相比,利用深度学习模型直接从大数据中学习特征,更有利于描述数据本身的丰富内涵信息[10]。最近,深度学习方法在计算机视觉领域表现出巨大的潜力,卷积神经网络[11]作为一种特殊的深度学习架构,凭借其优秀的表现受到广泛的关注,在一系列大规模、细粒度的图像识别任务中取得巨大成功[12]。卷积神经网络是一种新的目标分类识别方法,该方法能够实现自动化的图像特征提取,与分类识别过程融为一体,并通过数据实现自我学习[13-14]。制造业信息化程度高、劳动力密集、成本范围广大,是非常适合人工智能技术发挥的领域。同时,人工智能技术也非常适合解决制造业面临的挑战,如不稳定的质量及良率、生产线设计缺乏灵活性、产能管理困难以及生产成本上升等。人工智能技术可以帮助解决这些问题,改善质检流程,缩短设计周期,消除供应链瓶颈,减少材料和能源浪费,并且提高产量。为了能够将原本用于识别自然图像的卷积神经网络用来识别工业图像,需要注意到自然图像与工业图像的不同之处,并对网络结构与算法做出相应的调整与改进。自然图像(例如ImageNet数据集)普遍色彩丰富、种类繁多,形状、纹理、背景都极为复杂,而工业现场进行图像采集时环境相对稳定,同种零件每次采集到的图像差别不大,且目标突出,背景较为纯净。基于此,本文作者利用深度卷积神经网络的优势,并结合零件识别问题的特点,设计一种简化的卷积神经网络,对29种不同尺寸的螺丝、螺母、垫片进行分类,并且在图像预处理中添加一种基于边缘检测的目标中心化算法,同时验证卷积神经网络对于识别相同种类、不同尺寸的图像的准确率,对于卷积神经网络在尺寸测量方面的应用具有一定的参考价值。
1 图像采集与数据增强
1.1 图像采集
1.1.1 零件种类
待测的零件分为螺丝、螺母、垫片3类,每种零件又分为不同的尺寸,一有共29种零件。其中螺丝18种,垫片8种;螺母为M3,M4和M5共3种。
29种零件的编号及尺寸如表1所示。
表1 零件编号及尺寸
Table 1 Number and size of parts
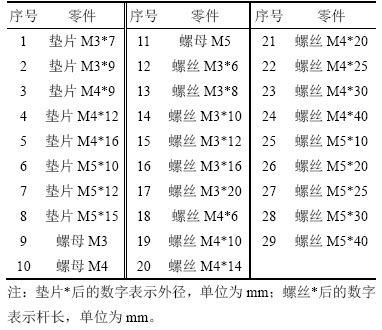
1.1.2 图像采集方法
图像采集时,摄像头拍摄方向垂直零件所处平面,且摄像头与零件的相对位置保持不变,如图1所示。这样既能模拟实际工况中位置固定的摄像头垂直拍摄传送带上运动的零件的情形,同时又能使零件在图像中的大小能够反映图像的实际大小。每种零件变换不同的角度、位置拍摄40次,以模拟实际工况中零件位置和摆放角度都是随机的。
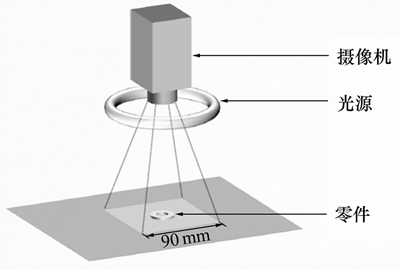
图1 图像采集示意图
Fig. 1 Diagram of image acquisition
1.2 数据增强
为防止网络过拟合,用于训练的数据量应尽可能大,但是,通过人工进行图像采集的方式获取数据的效率很低,想获得足够多的数据需要很长时间。对于图像识别问题来说,使用数据增强来扩大数据量是一种效率很高且行之有效的办法。图像识别问题中常用的数据增强方法有:平移、旋转、翻转、缩放、色彩偏移等。针对生产过程中的实际情况,选取了平移、旋转、亮度偏移、对比度偏移4种数据增强方法,未采用翻转的原因是待分类零件均为左右对称,旋转操作已经可以模拟零件所有角度的情况,没有必要再进行翻转操作;未采用翻转和缩放的原因是在该问题中,图像的大小代表零件的尺寸,缩放相当于改变零件尺寸,从而改变图像类别。
1.3 具体实现
图2所示为29种零件对应的原始图片。原始图像为3 120像素×3 120像素的灰度图像,对应零件所处平面的实际长×宽为90 mm×90 mm,首先对图像进行0°~360°范围随机角度的旋转。如图3所示,中间白色圆形区域为始终会被原图覆盖的区域。然后从图像中心裁切2 048像素×2 048像素的图像,左上角坐标为[460, 610]中的随机整数。这样裁剪既能保证图像外面的黑边会被完全切去,又能使图像中间的零件得到完整保留,同时具有位移上的随机性。再将裁剪后的图像缩放至128像素×128像素,此时,每个像素对应的实际长度为0.46 mm。最后,对该图像进行随机亮度偏移与对比度偏移,偏移比率均为0.9~1.1。每张图片重复100次,得到100张不同的图像。图4所示为其中3张原始图片进行数据增强后得到的部分结果。最终的数据包括29种零件,每张零件对应4 000张图片,共116 000张图片。
2 卷积神经网络
卷积神经网络是由LeCun等提出的一种专门用来处理具有类似网格结构数据的神经网络,例如时间序列数据和图像数据。卷积神经网络在诸多应用领域都表现优异[3]。卷积神经网络通过权值共享和卷积运算直接处理二维图像,避免了传统模式识别算法中复杂的特征提取和数据重建过程[15-18]。
卷积神经网络的基本结构包括卷积层和池化层。卷积层由一组待训练的卷积核构成。根据需要提取特征的密度,卷积核通过固定的步长与输入图像作卷积运算,经由激活函数变换后输出特征图。池化层通常在卷积层后面出现,通过对特征图进行下采样,减少特征维度并抑制干扰。
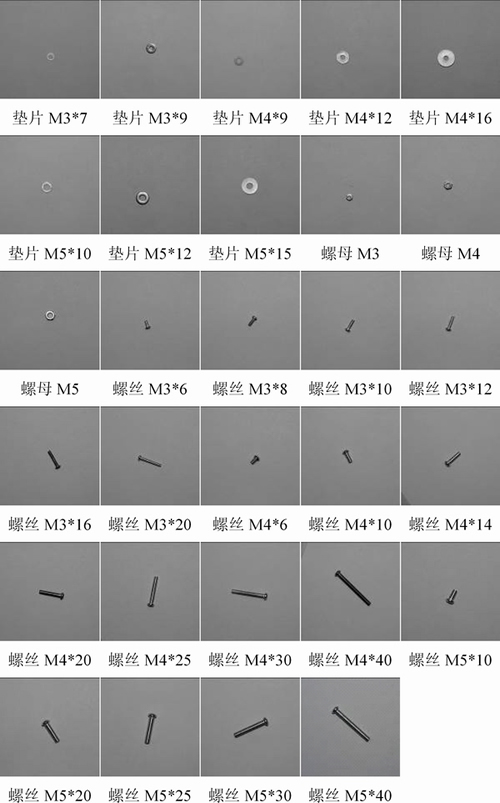
图2 原始零件图片
Fig. 2 Origin images of parts
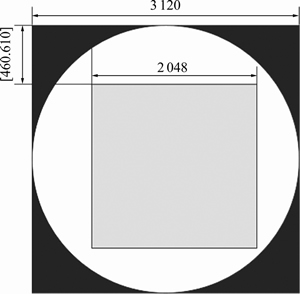
图3 随机裁切示意图(单位:像素)
Fig. 3 Diagram of random crop
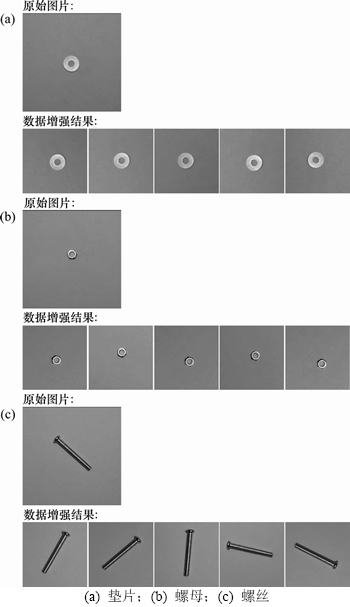
图4 数据增强结果
Fig. 4 Results of data augmentation
2.1 网络结构
根据卷积神经网络的基本结构并结合待分类数据集的特征,构建了如图5所示的卷积神经网络模型。卷积神经网络的搭建参考了AlexNet[19]和VGGNet[20]的结构。因待识别的零件形状较简单,所以,对网络结构进行了较大简化。
SIMONYAN等[20]指出,多个较小卷积核的层层叠加与一个较大卷积核具有相同大小的感受野,且参数更少,非线性更强。因此,在前2个卷积层中采用了2层3×3的卷积核,相当于对输入提供了5×5的感受野。
卷积网络的输入为1×128×128的经过数据增强后的图像;第1个卷积层包含32个3×3的卷积核,输出维度为32×126×126;第2个卷积层同样包含32个3×3的卷积核,输出维度为32×124×124;然后经过一个采样核大小为2×2的最大池化层,输出维度为32×62×62;第3个卷积层包含64个3×3的卷积核,输出维度为64×60×60;然后再经过一个采样核大小为3×3的最大池化层,输出维度为64×20×20;第4个卷积层包含128个3×3的卷积核,输出维度为128×18×18;然后再经过一个采样核大小为2×2的最大池化层,输出维度为128×9×9;再将这个三维向量展开成长度为10 368的一维向量,经过一个输出为256的全连接层,最后经过一个输出为29的全连接层,得到网络的输出。
所有卷积层的步长均为1,无填充;所有最大池化层的步长都与采样核的边长相同,无填充;除输出层的激活函数使用Softmax外,其余所有的激活函数均为整流线性单元。
在第1个卷积层后面和每个最大池化层后面都添加了0.25的Dropout[21],在第2个全连接层后面添加了0.5的Dropout。
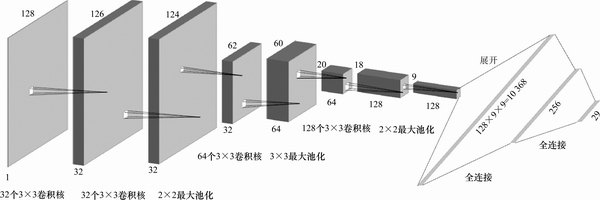
图5 卷积神经网络结构
Fig. 5 Diagrams of convolutional neural network
2.2 训练方法
每种零件的图片由40张原始图片经过100次数据增强得到,即每个种类拥有4 000张图像数据。将前28张原始图片数据增强后得到的2 800个图像作为训练集,后12张原始图片数据增强后得到1 200个图像作为测试集。因为测试集的原始图片与训练集的不同,所以,即使遇到全新的图片,网络的准确率也会与测试集的相同,并不会受到数据增强的影响。
本文训练网络采用的自适应学习率算法为Adam[22],学习率为1×10-4。训练时,每次输入32张图片,并对输入添加批标准化[23]。
3 目标中心化
在绝大多数机器学习算法以及训练神经网络的过程中,都需要对原始数据进行中心化和标准化处理。数据经过中心化和标准化处理后,会成为均值为0、标准差为1的服从标准正态分布的数据。中心化和标准化的意义是能够消除数据所包含的特征之间的差异性,使它们具有相同的尺度。在回归问题中,它能够取消由于量纲不同、自身变异或者数值相差较大所引起的误差;在神经网络的训练过程中,它能加速权重参数的收敛。
在神经网络训练的过程中,对输入添加了批标准化,这是一种针对图像的像素进行中心化和标准化的处理。但是,每张图像中零件的位置是随机出现的。虽然传统观念认为卷积神经网络对图像类别的判断与目标在图像中的位置无关,但是在零件识别问题中,零件所处背景较为纯净,而且相同种类不同大小的零件图像中目标的形状相似甚至相同,仅在大小上存在差别,即不同类别图像间的差距较小,且目标位置的随机性模糊了这种差距。在实际运行结果中也发现,卷积神经网络对于零件在整幅图像中占比较小,且种类相同、尺寸相近零件之间的区分准确率较低,对于某些尺寸较小且形状相似的零件,卷积神经网络的准确率甚至不如传统算法。
为了解决卷积神经网络对于形状、大小相似的目标不敏感的问题,本文提出了将图像中的目标进行中心化的方法。目标中心化算法的思路是:通过将图像中的待识别目标的位置移动到图像的中心处,使相同类别的不同图像间的差距尽可能缩小,从而突出不同类别图像之间的差距。该方法不仅有一定的理论依据作为支撑,在实际运行结果中,该方法也使神经网络的识别准确率得到了显著提升。
3.1 边缘提取
通过上下左右4个方向的Sobel算子提取边缘,这4个算子的形式如下:
(1)
(2)
(3)
(4)
与卷积运算类似,这4个算子分别从左到右、从上到下移动,每次移动的步长为1。设算子Fi覆盖到的原图像素组成的矩阵为x,则输出为
(5)
其中:
为Fi与x的点积(对应元素乘积之和);b为阈值;
为阶跃函数。滤波器选取合适的阈值(本文取值为20),使被激活的像素能覆盖零件的全部。4个算子生成4个边缘提取结果,再取并集,得到最终结果。部分图像的提取结果如图6所示。
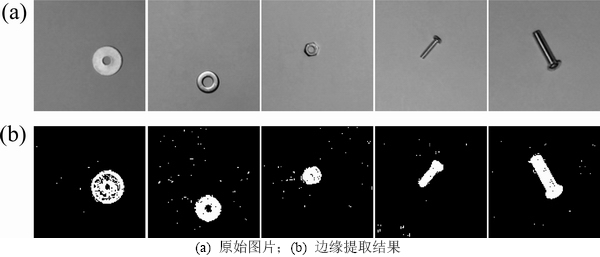
图6 部分图像边缘提取结果
Fig. 6 Results of edge extraction of some images
由图6可见,图像中有许多噪声干扰。为了正确判断零件位置,采用提取图像中的最大连通域的方法。这样防止了传统的滤波操作可能导致的噪声滤除不完全的问题,从而完全避免了噪声的干扰。
找出最大连通域后,得到它的矩形包围边框,提取原始图像边框内的图像,即为原始图像中零件部分的图像。
3.2 目标中心化
为了验证目标中心化算法的正确性,分别制作了3种数据集:第1种数据集仅提取矩形包围框内的图像,不改变图像位置;第2种数据集将提取到的目标置于图像左上角;第3种数据集将提取到的目标置于图像中心。这3种方法得到的数据集的部分图像如图7所示。
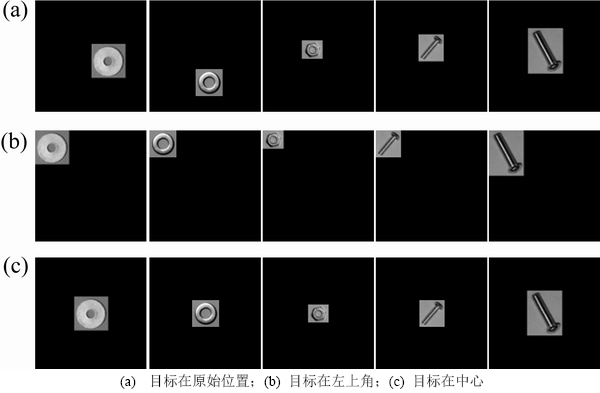
图7 3种数据集的部分图像
Fig. 7 Some images of three datasets
根据前面的理论分析,第1种数据集未改变目标位置,仅去掉大部分背景,相当于突出了目标所在位置,但因为并未改变目标位置,猜测该种数据集的准确率与原始数据集相似或稍有改善;第2种数据集将目标置于图像左上角处,统一了目标位置但并未统一至中心处,相当于一种略微弱化的中心化,与原始数据集的准确率相比应得到较明显的提升,但仍不如第3种数据集,即完全地将目标置于图像中心。
4 实验结果
4.1 网络训练
分别使用原始数据集和目标在原位置、左上角、中心的数据集训练网络,将训练集的全部数据在神经网络上训练80次。将每种数据集中的测试集的损失函数值变化曲线进行比较,结果如图8所示。
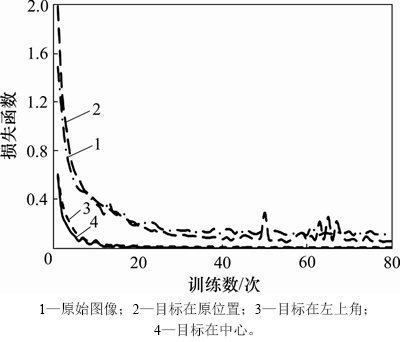
图8 损失函数值变化曲线
Fig. 8 Curves of loss function value
由图8可见,在训练过程中,原始图像和目标在原位置的数据集训练速度几乎相同,而目标在左上角和目标在中心的数据集训练速度远大于原始数据集的训练速度,且目标在中心的数据集的训练速度略大于目标在左上角数据集的训练速度,说明目标中心化能够强化数据的特征,加快网络收敛,在相同训练次数下,损失函数更小,从而达到减少训练时间的目的,且中心化程度越强,训练速度越快。
4.2 准确率
原始数据测试集综合准确率为97.59%,目标在原位置的测试集综合准确率为98.57%,说明仅去掉背景也能提升一定的准确率;而目标在左上角的测试集综合准确率为99.88%,目标在中心的测试集综合准确率为99.96%,说明目标中心化能够大幅提高网络的准确性,且准确率随中心化程度的增加而升高。
各测试集中每种零件的准确率如表2所示。
表2 每种零件的准确率
Table 2 Accuracy of each %
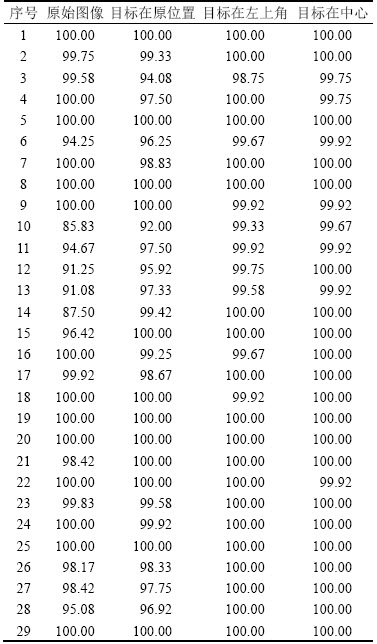
由表2可知:对原始图像进行分类,准确率最低的零件为10号,准确率为85.83%;29种零件中仅有15种零件的准确率大于99.9%;使用目标中心化算法后,每类零件的准确率都在99.5%以上,且26种零件的准确率均大于99.9%,10号零件的准确率也提升至99.67%,说明目标中心化算法能够十分显著地提高网络的准确率。
5 结论
1) 提出了一种基于边缘提取和最大连通域的目标中心化算法。在对29种不同尺寸的螺丝、螺母和垫片的分类问题中,系统的总体准确率从97.59%提升至99.96%。
2) 本文提出的算法能够对背景纯净的工业图像进行准确分类,当目标占图像区域较小且不同种类图像差距不大时,使用目标中心化算法对图像进行预处理能够显著提高网络的准确率。同时,对于识别相同形状、不同尺寸的图像,卷积神经网络也有很高的准确率。
参考文献:
[1] 何晓阳, 徐惠钢, 谢启. 基于LabVIEW与BP神经网络的零件识别系统[J]. 仪表技术与传感器, 2017(1): 119-122.
HE Xiaoyang, XU Huigang, XIE Qi. Recognition system of parts based on LabVIEW and BP neural network[J]. Instrument Technique and Sensor, 2017(1): 119-122.
[2] 何泽强. 基于机器视觉的工业机器人分拣系统设计[D]. 哈尔滨: 哈尔滨工业大学信息与电气工程学院, 2016: 1.
HE Zeqiang. Design of industrial robot sorting system based on machine vision[D]. Harbin: Harbin Institute of Technology. School of Information and Electrical Engineering, 2016: 1.
[3] GOODFELLOW I, BENGIO Y, COURVILLE A. Deep learning[M]. Cambridge, MA: The MIT Press, 2016: 3.
[4] 司小婷, 吴文江, 孙一兰. 基于视觉的零件识别和定位[J]. 组合机床与自动化加工技术, 2016(10): 70-73.
SI Xiaoting, WU Wenjiang, SUN Yilan. The identification and positioning of parts based on machine vision[J]. Modular Machine Tool & Automatic Manufacturing Technique, 2016(10): 70-73.
[5] 刘振宇, 李中生, 赵雪, 等. 基于机器视觉的工业机器人分拣技术研究[J]. 制造业自动化, 2013(17): 25-30.
LIU Zhenyu, LI Zhongsheng, ZHAO Xue, et al. Research of sorting technology based on industrial robot of machine vision[J]. Manufacturing Automation, 2013(17): 25-30.
[6] 吴益红, 许钢, 江娟娟, 等. 基于LBP和SVM的工件图像特征识别研究[J]. 重庆理工大学学报, 2016, 30(1): 77-84.
WU Yihong, XU Gang, JIANG Juanjuan, et al. Research on workpiece image feature recognition based on LBP and SVM[J]. Journal of Chongqing University of Technology (Natural Science), 2016, 30(1): 77-84.
[7] 冯长建, 吴斌, 罗跃纲. 混合KPCA和SVM的机械零件形状识别方法研究[J]. 机械制造与自动化, 2016(4): 132-134.
FENG Changjian, WU Bin, LUO Yuegang. Research on shape recognition of mechanical parts based on hybrid KPCA and SVM[J]. Machine Building & Automation, 2016(4): 132-134.
[8] 李春, 李琳, 邹焱飚, 等. 基于视觉的焊接工件在线识别与分类算法研究[J]. 价值工程, 2016, 35(4): 97-101.
LI Chun, LI Lin, ZOU Yanbiao, et al. Research on on-line recognition and classification of weldment based on machine vision[J]. Value Engineering, 2016, 35(4): 97-101.
[9] HINTON G E, SALAKHUTDINOV R R. Reducing the dimensionality of data with neural networks[J]. Science, 2006, 313(5786): 504.
[10] 赵鹏, 王斐, 刘慧婷, 等. 基于深度学习的手绘草图识别[J]. 四川大学学报(工程科学版), 2016, 48(3): 94-99.
ZHAO Peng, WANG Fei, LIU Huiting, et al. Sketch recognition using deep learning[J]. Journal of Sichuan University (Engineering Science Edition), 2016, 48(3): 94-99.
[11] L
CUN Y, BOTTOU L, BENGIO Y, et al. Gradient-based learning applied to document recognition[J]. Proceedings of the IEEE, 2001, 86(11): 2278-2324.
[12] 杨国国, 鲍一丹, 刘子毅. 基于图像显著性分析与卷积神经网络的茶园害虫定位与识别[J]. 农业工程学报, 2017, 33(6): 156-162.
YANG Guoguo, BAO Yidan, LIU Ziyi. Localization and recognition of pests in tea plantation based on image saliency analysis and convolutional neural network[J]. Transactions of the Chinese Society of Agricultural Engineering, 2017, 33(6): 156-162.
[13] 周云成, 许童羽, 郑伟, 等. 基于深度卷积神经网络的番茄主要器官分类识别方法[J]. 农业工程学报, 2017, 33(15): 219-226.
ZHOU Yuncheng, XU Tongyu, ZHENG Wei, et al. Classification and recognition approaches of tomato main organs based on DCNN[J]. Transactions of the Chinese Society of Agricultural Engineering, 2017, 33(15): 219-226.
[14] SERMANET P, EIGEN D, ZHANG X, et al. OverFeat: integrated recognition, localization and detection using convolutional networks[EB/OL]. [2013-12-21]. https://arxiv.org/ abs/1312.6229.
[15] HE K, ZHANG X, REN S, et al. Deep Residual Learning for Image Recognition[C]// Computer Vision and Pattern Recognition. Piscataway, NJ: IEEE, 2016: 770-778.
[16] 高学, 王有旺. 基于CNN和随机弹性形变的相似手写汉字识别[J]. 华南理工大学学报 (自然科学版), 2014, 42(1): 72-76.
GAO Xue, WANG Youwang. Recognition of similar handwritten Chinese characters based on CNN and random elastic deformation[J]. Journal of South China University of Technology(Natural Science), 2014, 42(1): 72-76.
[17] GLOROT X, BORDES A, BENGIO Y, et al. Deep sparse rectifier neural networks[C]// International Conference on Artificial Intelligence and Statistics. Cambridge, MA: The MIT Press, 2012: 315-323.
[18] ZHOU Y T, CHELLAPPA R. Computation of optical flow using a neural network[C]// IEEE International Conference on Neural Networks. Piscataway, NJ: IEEE, 1988: 71-78.
[19] KRIZHEVSKY A, SUTSKEVER I, HINTON G E. ImageNet classification with deep convolutional neural networks[J]. Communications of the ACM, 2017, 60(2): 2012.
[20] SIMONYAN K, ZISSERMAN A. Very Deep Convolutional networks for large-scale image recognition[EB/OL]. [2014-10-15]. https://arxiv.org/abs/1409.1556.
[21] SRIVASTAVA N, HINTON G, KRIZHEVSKY A, et al. Dropout: a simple way to prevent neural networks from overfitting[J]. Journal of Machine Learning Research, 2014, 15(1): 1929-1958.
[22] KINGMA D P, BA J. Adam: A method for stochastic optimization[EB/OL]. [2014-12-22]. https://arxiv.org/abs/ 1412.6980.
[23] IOFFE S, SZEGEDY C. Batch normalization: accelerating deep network training by reducing internal covariate shift[EB/OL]. [2015-01-11]. https://arxiv.org/abs/1502.0316.
(编辑 赵俊)
收稿日期:2018-03-12;修回日期:2018-04-26
基金项目(Foundation item):国家自然科学基金资助项目(61402374) (Project(61402374) supported by the National Natural Science Foundation of China)
通信作者:吴爱国,教授,博士生导师,从事智能化工艺集成系统开发研究;E-mail:agwu@tju.edu.cn