DOI: 10.11817/j.issn.1672-7207.2017.07.012
基于奇异值分解极限学习机的维修等级决策
刘璟忠1, 2
(1. 湖南大学 信息科学与工程学院,湖南 长沙,410082;
2. 湖南工学院 数理科学与能源工程学院,湖南 衡阳,421002)
摘要:为降低航空发动机维修成本,增强维修等级决策的客观性,提出一种基于奇异值分解的极限学习机(SVD-ELM)算法,推导基于奇异值分解(SVD)的极限学习机(ELM)输出权重计算公式,从而有效地避免普通ELM在求解输出权重时因矩阵奇异而导致无法求逆的问题。将SVD-ELM应用于决策建模过程,提高决策模型的稳定性。研究结果表明:相比于SVM,SVD-ELM和ELM的决策准确率相同,且均比SVM的高,但SVD-ELM的模型稳定性高于ELM,且SVD-ELM和ELM的测试耗时相差不大,说明这2种方法的计算量相当。
关键词:智能决策;极限学习机;模式识别;维修等级;奇异值分解
中图分类号:TP206 文献标志码:A 文章编号:1672-7207(2017)07-1769-05
Maintenance level decision based on singular value decomposition of extreme learning machine
LIU Jingzhong1, 2
(1. College of Computer Science and Electronic Engineering, Hunan University, Changsha 410082, China;
2. School of Mathematics, Physics and Energy Engineer, Hunan Institute of Technology, Hengyang 421002, China)
Abstract: In order to reduce the cost of aviation engine maintenance and enhance the objectivity of maintenance level decision, singular value decomposition based extreme learning machine (SVD-ELM) algorithm was proposed. The output weight formula of extreme learning machine (ELM) was deduced based on singular value decomposition (SVD). Unlike conventional ELM, SVD-ELM effectively avoids the failure of calculating matrix inversion due to matrix singular, during the process of computing output weight. Then SVD-ELM was applied in decision modeling process, which increased decision model stability. The results show that compared with SVM, the decision accuracy of SVD-ELM is the same as ELM, which are both higher than that of SVM. But SVD-ELM stability is greater than ELM. Meanwhile, testing time of SVD-ELM and ELM is similar, indicating that these two methods have the same computing amount.
Key words: intelligent decision; extreme learning machine; pattern recognition; maintenance level; singular value decomposition
发动机在航使用时需要满足一定的适航标准,当检测出其健康状态不适合工作时,必须进行不同等级的维修,以期恢复发动机的性能[1-3]。在进行维修之前,性能工程师首先需要根据发动机的工作状态确定其维修等级[4]。维修成本与维修等级有很强的关联性,维修等级越高,维修耗时和维修成本也随之显著增高。若为了降低本次维修的成本而设定较低的维修等级,则有可能缩减发动机下次的在航时间,使得平均每飞行1 h的维修成本增加。因此,若能够根据发动机的性能指标选择合理的维修等级,则能有效降低航空公司的维修成本。但是,在确定维修等级时可能存在如下困难:1) 由于发动机内部结构复杂,很难根据整机的性能参数确定具体的退化部件及确定相应的维修等级;2) 维修人员缺乏对典型案例的掌握和实践经验的积累,使得很难根据人为经验确定合理的维修等级[5]。神经网络作为一种数据挖掘方法,通过学习已知的案例使其具备对维修等级的决策能力,从而给维修人员提供辅助决策。极限学习机(ELM)作为近年来发展起来的一种单隐层前馈神经网络学习算法[6-8],具有学习速度快、泛化能力强等优点[9-10],已经在时间序列预测[11-14]、模式识别[15-17]等领域得到了应用。本文针对ELM在计算输出权重时因矩阵奇异而导致无法求逆的问题,将ELM引入维修决策建模过程中,推导基于SVD的ELM输出权重计算公式,从而有效避免求逆无效的问题,提高维修决策模型的稳定性。
1 极限学习机
ELM的数学表达式为[18]
(1)
式中:L为隐节点数目;
,
,为隐含层参数;
,为第i个隐节点对于输出节点的权重系数;
为第i个隐节点的输出函数。加性隐节点函数可以表示为
(2)
式中:ai为第i个隐含层节点的输入权重;bi为第i个隐含层节点的偏差;
为激活函数。
假设已知由N组输入输出数据对构成的训练样本
(其中,
为输入变量;
为相应的实际输出),则
(3)
将式(3)变为矩阵形式[19],则
(4)
式中:
,
,
(5)
对于式(3),参数ai和bi利用随机生成方式获得,参数β则根据
计算得出。在解决分类问题时,为了提高ELM的分类准确性,文献[20]引入正则化因子λ,从而可以将求解β看成如下优化问题:
(6)
训练偏差
可以有效避免过拟合问题,依照Karush-Kuhn-Tucker定理[21],式(6)的求解问题可以转化成双重优化问题。
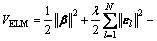
(7)
根据式(7)计算β的最优解为[21]
(8)
ELM的决策方程按下式求得:
(9)
(10)
式中:
为第i个输出节点的值,且有
。
为x对应的标签值。
2 基于奇异值分解的极限学习机
2.1 基于SVD的输出权重计算
由式(8)可以看出:在计算输出权重β时,若
近似为奇异矩阵,则对其求逆
会使得计算结果不稳定。为解决矩阵求解无效问题,本文采用基于SVD的输出权重求解式(8)中的β,下面给出具体推导过程。
基于奇异值分解,ELM中的H矩阵可以表示为
(11)
则
(12)
式中:P为将S中所有非零元素取倒数。
式(8)中的β可以变换为如下形式:

(13)
2.2 SVD-ELM算法流程
给定训练样本集
,其中
为输入向量,
为类别标签,对于发动机维修等级决策问题而言,输入向量为发动机的性能参数,类别标签为对应的维修等级,整理得出基于奇异值分解的极限学习机算法,步骤如下。
步骤1 确定SVD-ELM中的正则化因子λ和隐节点数L。
步骤2 随机产生输入层权值ai和隐含层偏差bi,i=1, 2, …, L。
步骤3 将
,ai和bi代入式(5),计算初始的隐含层输出矩阵H。
步骤4 将H代入式(11),对H进行奇异值分解(SVD),得到矩阵V,P,S和U,然后根据式(13),计算基于SVD的输出权重β。
步骤5 对于待分类的输入向量xk,计算对应的
,代入式(14)得到
,将
代入式(9),得到其所属类别,即为维修等级决策结果。
(14)
3 数据仿真验证
为验证本文方法的有效性,选取6种标准的UCI数据作为实验对象,将归一化后的UCI数据作为训练样本和测试样本。本文选择的样本包括二分类和多分类数据,具体的数据名称为:wdbc,haberman,parkinsons,iris,glass和zoo。
为证明本文提出方法的有效性,利用支持向量机(SVM)和ELM作为对比方法,其中SVM采用LIBSVM工具箱提供的SVM函数。实验运行系统如下:Windows XP,Intel酷睿i3 2120处理器(CPU主频 3.3 GHz),2 GB内存,仿真软件为 MATLAB R2011b。
对于SVM,惩罚参数C和核参数γ影响维修等级决策的准确率;对于ELM和本文提出的SVD-ELM,正则化因子λ和隐节点数L影响决策准确性。为此,对于SVM,令C和γ均为
,根据网格搜索法求出每1对C和γ对应的测试样本决策准确率。同理,对于ELM和SVD-ELM,令λ=
,L=
,计算测试准确率。
利用网格搜索法得到的测试准确率最高值及其对应的参数取值、测试耗时如表1所示。由表1可以看出:对于zoo,ELM和SVD-ELM方法的分类准确率比SVM方法的低;对于parkinsons和glass,3种方法的准确率相同;对于wdbc, haberman和iris,SVD-ELM的准确率比SVM的高。此外,对于上述所有6种UCI数据,SVD-ELM和ELM的分类准确率相同,说明本文采用基于SVD的输出权重计算公式与ELM中采用Moore-Penrose广义逆矩阵求解输出权重矩阵的结果一致,从而证明了SVD-ELM在分类问题中的有效性。
表1 UCI数据测试准确率最高值、对应的参数值以及耗时
Table 1 The highest testing accuracy, corresponding parameters and time consuming for UCI data
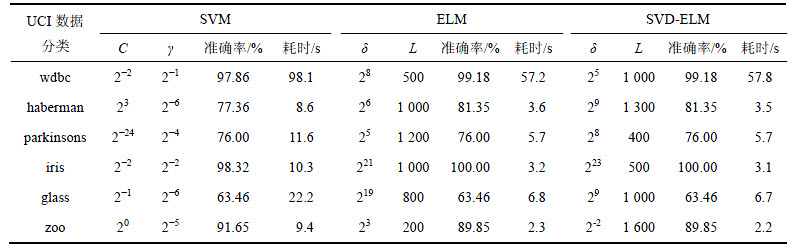
4 实例验证
以某型民用航空发动机维修数据为例,说明本文提出方法的有效性。根据维修经验,发动机的维修等级主要包括一般检查、性能恢复和翻修3种。维修等级主要根据发动机的性能参数确定。能够反映发动机性能的参数包括气体排放温差(C1)、高压转子转速偏差(C2)、低压转子振动偏差(C3)、油料流量偏差(C4)和高压转子振动偏差(C5)等。该维修数据共有93组数据,其中部分性能参数值与对应的维修等级如表2所示。选取其中的50组数据作为训练样本,用于建立决策模型;其余的43组数据作为测试样本,检验建立的决策模型的准确性。
表2 部分性能参数值与维修等级
Table 2 Partial performance parameters and maintenance level
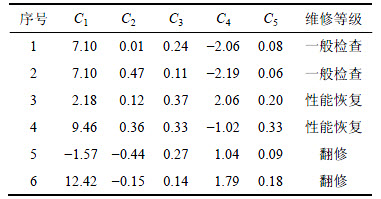
采用上一节中同样的参数寻优方法,所得SVM,ELM和SVD-ELM这3种方法对测试样本的维修等级决策准确率如图1所示,测试准确率最高值、对应的参数值以及耗时如表3所示。
由图1和表3可以看出:ELM和本文提出的SVD-ELM方法的测试准确率均比SVM方法的高,但对于传统的ELM方法(如图1(b)所示),在(λ=2-24 , L=500),(λ=2-23 , L=1 200)和(λ=2-25 , L=1 500) 3种情况下,由于
为奇异矩阵,导致ELM在计算输出权重时求解逆矩阵
失效,从而这3种参数取值时无法建立基于ELM的维修等级决策模型。而本文提出的方法采用基于SVD的输出权重计算公式,避免了因矩阵奇异导致求逆无效的问题,从而保证维修等级决策模型的稳定性,如图1(c)所示。此外,ELM和SVD-ELM方法的耗时相当,说明SVD-ELM方法的计算量与ELM方法的计算量相当。
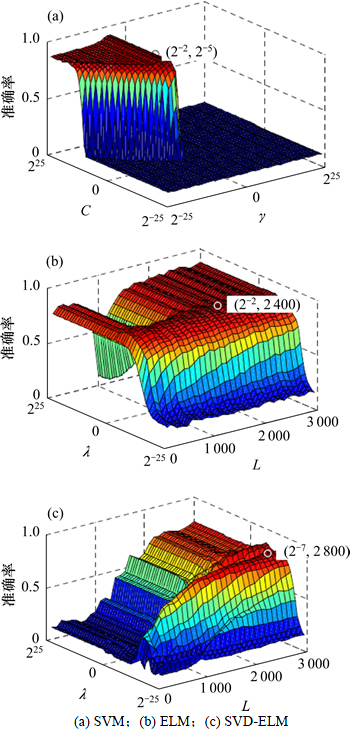
图1 不同参数的维修等级决策准确率
Fig. 1 Maintenance level decision accuracy for different parameters
表3 维修决策准确率最高值、对应的参数值以及耗时
Table 3 The highest testing accuracy, corresponding parameters and time consuming for maintenance decision
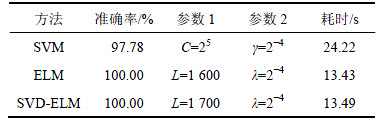
5 结论
1) 为解决发动机维修等级决策问题,提出了一种基于奇异值分解的极限学习机(SVD-ELM)算法。该算法利用ELM能够提高决策准确性的优点,以及SVD能够有效避免因矩阵奇异导致求解输出权重无效的特点,使得SVD-ELM具有更高的维修等级决策准确率,同时能够保证决策模型的稳定性。
2) 本文提出的SVD-ELM的维修等级决策的准确率比SVM的高。对于传统的ELM,在某些参数取值条件下,ELM出现了求解输出权重失效的现象,而SVD-ELM方法则不仅能够保证建模的稳定性,且其算法计算量与ELM的计算量相当,从而证明了SVD-ELM方法的有效性。
参考文献:
[1] 郑波. 基于PSO-SVM的民航发动机送修等级决策研究[J]. 推进技术, 2013, 34(5): 687-692.
ZHENG Bo. Investigation on aeroengine maintenance level decision based on PSO-SVM[J]. Journal of Propulsion Technology, 2013, 34(5): 687-692.
[2] LIANG Zhongao, YANG Miao. Research on maintenance level decision of civil aero-engine module[J]. Applied Mechanics & Materials, 2013, 368/369/370: 1396-1399.
[3] HUYNH K T, BARROS A, BERENGUER C. Multi-level decision-making for the predictive maintenance of -out-of-: F deteriorating systems[J]. IEEE Transactions on Reliability, 2015, 64(1): 94-117.
[4] 付旭云, 陈银, 钟诗胜. 基于寿命件的民航发动机送修目标确定方法[J]. 航空动力学报, 2014, 29(7): 1556-1561.
FU Xuyun, CHEN Yin, ZHONG Shisheng. Approach for civil aero-engine repair objective determination based on life limited parts[J]. Journal of Aerospace Power, 2014, 29(7): 1556-1561.
[5] 付旭云, 陈银, 钟诗胜. 基于性能状态的民航发动机送修目标确定方法[J]. 计算机集成制造系统, 2013, 19(12): 3002-3007.
FU Xuyun, CHEN Yin, ZHONG Shisheng. Civil aero-engine repair objective determination based on performance state[J]. Computer Integrated Manufacturing Systems, 2013, 19(12): 3002-3007.
[6] HUANG Guangbin, ZHU Qinyu, KHEONG S C. Extreme learning machine: theory and applications[J]. Neurocomputing, 2006, 70(1): 489-501.
[7] YUAN Lan, CHAI S Y, HUANG Guangbin. Ensemble of online sequential extreme learning machine[J]. Neurocomputing, 2009, 72(13): 3391-3395.
[8] YANG Bo, DENG Zhidong. An extended SHESN with leaky integrator neuron and inhibitory connection for Mackey-Glass prediction[J]. Frontiers of Electrical and Electronic Engineering in China, 2012, 7(2): 200-207.
[9] FOSSACECA J M, MAZZUCHI T A, SARKANI S. MARK-ELM: application of a novel multiple kernel learning framework for improving the robustness of network intrusion detection[J]. Expert Systems with Applications, 2015, 42(8): 4062-4080.
[10] Akusok A, Veganzones D, Miche Y, et al. MD-ELM: originally mislabeled samples detection using OP-ELM Model[J]. Neurocomputing, 2015, 159: 242-250.
[11] GU Yang, LIU Junfa, CHEN Yiqiang, et al. TOSELM: timeliness online sequential extreme learning machine[J]. Neurocomputing, 2014, 128(27): 119-127.
[12] 张弦, 王宏力. 具有选择与遗忘机制的极端学习机在时间序列预测中的应用[J]. 物理学报, 2011, 60(8): 68-74.
ZHANG Xian, WANG Hongli. Selective forgetting extreme learning machine and its application to time series prediction[J]. Acta Physica Sinica, 2011, 60(8): 68-74.
[13] PAN Feng, ZHAO Haibo. Online sequential extreme learning machine based multilayer perception with output self feedback for time series prediction[J]. Journal of Shanghai Jiaotong University, 2013, 18(3): 366-375.
[14] GU Suicheng, TAN Ying, HE Xingui. Recentness biased learning for time series forecasting[J]. Information Sciences, 2013, 237(13): 29-38.
[15] LUO Xiaozhuo, LIU F, YANG Shuyuan, et al. Joint sparse regularization based sparse semi-supervised extreme learning machine (S3ELM) for classification[J]. Knowledge-Based Systems, 2014, 73: 149-160.
[16] AHILA R, SADASIVAM V, MANIMALA K. An integrated PSO for parameter determination and feature selection of ELM and its application in classification of power system disturbances[J]. Applied Soft Computing, 2015, 32: 23-37.
[17] LUO Xiong, CHANG Xiaohui. A novel data fusion scheme using grey model and extreme learning machine in wireless sensor networks[J]. International Journal of Control, Automation, and Systems, 2015, 13(3): 539-546.
[18] DONG Xiao, WANG Jichun, MAO Zhizhong. The research on the modeling method of batch process based on OS-ELM-RMPLS[J]. Chemometrics and Intelligent Laboratory Systems, 2014, 134(5): 118-122.
[19] LIAN Cheng, ZENG Zhigang, YAO Wei. Ensemble of extreme learning machine for landslide displacement prediction based on time series analysis[J]. Neural Computing and Applications, 2014, 24(1): 99-107.
[20] MARTINEZ-MARTINEZ J M, ESCANDELL-MONTERO P, SORIA-OLIVAS E, et al. Regularized extreme learning machine for regression problems[J]. Neurocomputing, 2011, 74(17): 3716-3721.
[21] HUANG Guangbin, ZHOU Hongming, DING Xiaojian, et al. Extreme learning machine for regression and multiclass classification[J]. IEEE Transactioins on Systems, Man, and Cybernetics. Part B: Cybernetics, 2012, 42(2): 513-529.
(编辑 伍锦花)
收稿日期:2016-09-16;修回日期:2016-11-24
基金项目(Foundation item):国家自然科学基金资助项目(71501068) (Project(71501068) supported by the National Natural Science Foundation of China)
通信作者:刘璟忠,副教授,从事图论、算法、计算机应用、数学建模等研究;E-mail: hnhyls@126.com