
DOI: 10.11817/j.issn.1672-7207.2021.03.001
基于CNN-LSTM的岩爆危险等级时序预测方法
刘慧敏1, 2,徐方远1, 2,刘宝举1, 2,邓敏1, 2
(1. 中南大学 地球科学与信息物理学院,湖南 长沙,410083;
2. 中南大学 湖南省地理空间信息工程技术研究中心,湖南 长沙,410083)
摘要:岩爆灾害是一种典型的矿山动力灾害现象,具有高度不确定性和不可预知性,严重威胁矿井安全生产。为了防止岩爆灾害破坏事故,需要掌握准确可靠的预测预报信息。为了预测岩爆未来时刻危险等级,提出一种基于CNN-LSTM的集成方法。首先,选取微震监测角频率、能量和凹凸体半径等特征量,根据灰色关联方法求出特征量关联度作为权重以标记岩爆危险指数;第二,对具有混沌特性的特征量时序数据进行相空间重构以表达其空间特征;第三,根据卷积神经网络(CNN)提取重构后相空间的空间特征,利用长短期记忆网络(LSTM)学习时间序列特征,预测岩爆特征量的未来状态;最后,使用粒子群算法优化广义神经网络模型(PSO-GRNN),并根据岩爆特征量未来状态评估其危险等级。实验结合冬瓜山铜矿微震监测实际工程,使用CNN-LSTM预测角频率、能量和凹凸体半径等未来状态,并采用PSO-GRNN预测其未来状态的岩爆等级。研究结果表明:本文提出的集成方法能够有效利用微震监测数据提前预测岩爆特征的未来状态及岩爆危险等级;与现有支持向量机和BP神经网络等经典方法相比,本文方法得到的未来状态预测值准确性更高。本研究成果为正确识别矿山岩爆当前活动及未来状态时的危险性提供理论支撑,为及时掌握矿山岩爆活动未来状态提供重要依据,同时,也可利用本文方法为类似岩爆灾害的其他地质灾害预警提供参考。
关键词:微震;相空间重构;CNN-LSTM模型;岩爆未来状态危险性;集成方法
中图分类号:P624.8 文献标志码:A 开放科学(资源服务)标识码(OSID):
文章编号:1672-7207(2021)03-0659-12
Time-series prediction method for risk level of rockburst disaster based on CNN-LSTM
LIU Huimin1, 2, XU Fangyuan1, 2, LIU Baoju1, 2, DENG Min1, 2
(1. School of Geosciences and Info-Physics, Central South University, Changsha 410083, China;
2. Hunan Geospatial Information Engineering and Technology Research Center, Central South University, Changsha 410083, China)
Abstract: Rock burst disaster is a kind of typical mine dynamic disaster phenomenon, which has high uncertainty and unpredictability, and seriously threatens mine safety production. In order to prevent the occurrence of rockburst disaster, it is necessary to master more accurate and reliable forecast information. In order to predict the danger level of rockburst in the future, an integrated method based on CNN-LSTM was proposed. Firstly, according to the grey correlation method, the correlation degree of the angular frequency, energy and concave convex radius were calculated as the weight to mark the rockburst risk index. Secondly, phase space reconstruction was carried out to express the spatial characteristics of these characteristic variables. Thirdly, the spatial features of reconstructed phase space were extracted according to the convolutional neural network(CNN), and the long and short term memory network(LSTM) was used to learn the time series features and predict the future state of rockburst characteristic variables. Finally, Particle swarm optimization(PSO-GRNN) was used to optimize the generalized neural network model, and the risk level of rock burst was evaluated according to the future state of rockburst characteristic variables. In the experiment, in combination with the actual microseismic monitoring project of Dongguashan Copper Mine, CNN-LSTM was used to predict the future state of angular frequency, energy, concave-convex radius, etc., and PSO-GRNN was used to predict rock burst risk level. The results show that the integrated method proposed in this paper can effectively predict the future state of rockburst characteristic variables and the rockburst risk level in advance with the microseismic monitoring data. Moreover, compared with the existing classical methods such as support vector machine and BP neural network, the predicted accuracy obtained by the proposed method is higher. The results provide theoretical support for correctly identifying the risk of rock burst in the current and future state, and provide an important basis for timely mastering the future state of rock burst. Furthermore, the method can also be used to provide reference information for the early warning of other geological disasters similar to rock burst disasters.
Key words: micro-seismic; phase space reconstruction; CNN-LSTM model; future state risk of rock burst; integrated method
矿山岩爆[1-2]一直是矿山行业重大的安全威胁之一,其灾害的发生具有高度不确定性,深井硬岩矿山中的岩爆预测与控制是矿山安全生产的重大理论与技术课题。监测及预测岩爆能够有效控制岩爆或减轻其危害,同时,国内外针对岩爆灾害已投入众多监测资源并获取了大量多源异构监测数据,为更好地对深井岩爆动态把握,需采用预防预警以提供数据支持[3-4]。
岩爆的产生是一个孕育演化的动态过程[4]。赵洪波等[5]采用支持向量机建立冲击地压序列之间的非线性关系,提出冲击地压预测的PSO-SVM模型;谭云亮等[6]建立了冲击地压AE时间序列小波神经网络预测模型。此外,人们通过声发射[7]、电磁辐射[8]及微震监测技术[9]获取岩爆状态数据,分析岩爆发生状态、机制及倾向性。基于声发射和电磁辐射等时间序列监测数据的预测模型非常多,如经典的神经网络、突变理论及灰色系统理论等[7-11]。岩爆和冲击地压预测研究领域中,彭琦等[12]使用现场岩爆监测的声发射时间序列数据,基于小波神经网络进行非线性拟合预测。同时,XIE等[13-14]证实岩爆的孕育和发生是动力学行为且具有混沌特性。预测冲击地压时,考虑到冲击地压发生过程具有混沌特征,陶慧等[15]提出基于多变量时间序列相空间重构GRNN模型,利用强逼近能力与快学习速度的广义神经网络GRNN模型进行预测,结合遗传优化算法寻找重构相空间参数嵌入维m、延迟时间τ及GRNN光滑因子σ的最佳组合,使用多状态量重构后的相空间作为输入,完成了冲击地压预测实验,具有创新性和工程适应性。
岩爆危险性预测是岩爆研究领域的中另一重要方向。智能岩石力学理论提出将人工智能方法与岩石力学交叉、融合,从岩体问题的实际出发,全面系统地研究岩石力学问题。白云飞等[16]将Fisher判别分析(FDA)理论应用到深部硬岩岩爆预测中,选取洞壁围岩最大切向应力、岩石单轴抗压强度、抗拉强度和弹性能量指数4项指标,选取国内外15个深部岩体实例作为训练样本,并利用训练好的模型对冬瓜山硬岩矿山和某煤矿三水平西大巷深部开拓巷道岩爆进行预测;贾义鹏等[17]选取上述4项指标,引入粒子群算法PSO和广义回归神经网络模型GRNN研究岩爆预测,取得了较好的实验效果。在选取反映岩爆特征因子时,大部分研究是从岩爆孕育产生过程中的岩石力学角度出发,以能量理论为基础,从各种相关因素中筛选出岩爆主要特征变量如岩体强度、洞壁围岩应力、抗压强度、抗拉强度以及弹性能量指数等[18-20]。这些特征能从物理层面反映岩爆危险性,然而其很难实现实时监测预警。
综上所述,现有单一时序预测方法的无法精确地刻画岩爆孕育演化的非线性动态过程,虽然部分研究结论的拟合预测精度较高,但是并没有考虑具有时序特性的岩爆未来状态多特征量的预测;同时,在岩爆危险性方面,传统的岩爆静态数值分析方法忽略了对未来状态下岩爆危险指数的识别,为此,大量学者从微震、电磁辐射、声发射和红外辐射等时序性监测数据出发进行研究,以克服岩爆静态分析的不足,但仍然存在单一特征量不足以精确反映岩爆孕育过程的问题。
本文提出一种集成学习方法预测岩爆特征量及未来状态下的岩爆危险等级。首先,考虑岩爆多特征量的混沌特性对微震监测时序数据进行相空间重构,并利用卷积神经网络CNN提取重构后相空间的空间特征以获取特征时间序列。进而,考虑到岩爆发生过程的动态演化特性,本研究基于长短期记忆网络LSTM学习各变量时间序列特征并预测岩爆未来状态。此外,本文综合考虑多类岩爆特征量,如角频率、能量指数和凹凸体半径等,综合反映岩爆的危险等级。为了识别多特征量的未来状态下岩爆的危险等级,本研究使用改进的粒子群算法优化GRNN模型,识别岩爆未来状态下的危险指数。
1 基于相空间重构的岩爆状态值预测
针对岩爆特征变量具有的混沌特性,首先重构特征量时序数据的状态相量空间,求出重构相空间的2个参数即序列嵌入维度m和序列延迟时间τ,并以此还原原始空间;然后,将序列嵌入维度m和序列延迟时间τ作为CNN模型的输入以提取空间特征的序列,进而通过LSTM模型预测出岩爆特征量t+1时刻状态,其流程如图1所示。
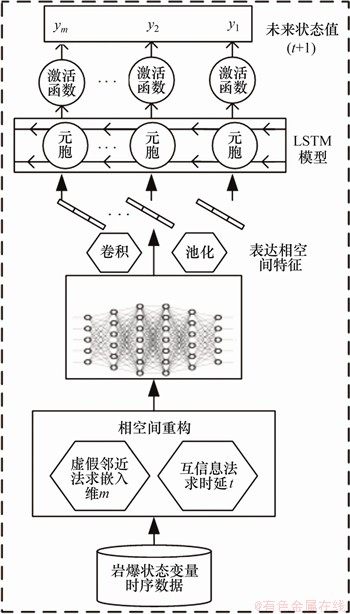
图1 CNN-LSTM模型的岩爆特征量的未来状态值预测流程图
Fig. 1 Prediction flow chart of future state of rock burst by CNN-LSTM model
1.1 岩爆特征量的相空间重构
现场获取的监测时间序列数据具有明显混沌特性,为了还原原始空间[21-22],需要计算重构岩爆状态变量相空间的参数嵌入维度和延迟时间。基于混沌理论使用互信息法求取延迟时间τ,基于虚假邻近点法FNN求嵌入维度m。然后,利用以上参数进行原始监测数据的相空间重构,还原其真实空间。依据嵌入定理,对于监测时序数据
(
1,2,
,
;I为特征变量数,N为时间序列的长度),第i个特征变量的重构空间状态为

(1)
式中:Xi,n为第i个特征变量相空间;
为第i个特征变量相空间上的一点;mi
第i个特征变量嵌入维度;τi为第i个特征变量延迟时间;n∈[1,2,…,N ]。经过相空间重构后,存在映射函数F:H m→H m,使得
(2)
式中:l为预测步数,即可根据重构后的状态变量相空间来预测时间序列Xn+1即未来l步的状态。
1.2 基于CNN-LSTM模型的岩爆特征量预测
利用卷积神经网络对重构后的相空间进行空间特征提取,卷积神经网络(convolutional neural networks,CNN)是一种包含卷积计算且具有深度网络的模型,其针对空间数据具有很强的表达能力。岩爆的发生是一个孕育演化的渐变过程,也是一个非线性变化的动力学过程,岩爆失稳前后岩石中的应力存在一个明显的由蕴含到释放的转变阶段。为更好地刻画这种非线性过程,采用长短期记忆网络模型(long short-term memory,LSTM)[23]预测岩爆特征量。LSTM能够动态记忆历史信息,已广泛应用在如煤矿瓦斯预测预警[24]和轨迹预测[25]等领域并取得了较好效果。利用LSTM从原始岩爆监测数据中学习新信息,同时能够保持监测数据历史信息的状态,在岩爆危险等级时序预测的应用能够充分发挥其在分析时间序列方面的天然优势[26-27]。
LSTM网络的基本单元中包含遗忘门、输入门和输出门[28]。
遗忘门中上一时刻单元状态的输入与状态记忆单元、中间输出共同决定状态记忆单元遗忘部分。
输入门中的状态分别经过sigmoid和tanh函数变化后共同决定状态记忆单元中保留向量。其中,中间输出由更新后的记忆单元与输出共同决定。
本文利用LSTM模型对卷积神经网络提取重构相空间的特征序列建模,通过学习该特征时间序列,表达岩爆演化的非线性过程,更加准确地预测岩爆特征量的未来值即t+1时刻岩爆特征量,同时为预测岩爆特征量未来值的危险等级做好准备。
构建的CNN-LSTM模型可以看成2个部分:卷积神经网络CNN部分和长短时记忆网络LSTM部分。其中,前者用于底层表达及抽取重构相空间的空间特征信息,以作为LSTM的输入;后者用于接收CNN提取特征的输出,并利用其在时序上具有的记忆特性准确提取时间序列特征,预测未来岩爆状态值,产生最终预测结果。其中,CNN-LSTM模型预测岩爆特征量未来状态值的主要过程如下。
1) CNN部分以重构的相空间状态二维矩阵Xi,n=[Xi,n,Xi,n-τi,…,Xi,n-(mi-1)τi]作为输入,Xi,n是一个二维矩阵,为[n-(mi-1)τi,m],表示还原系统的相空间,其中一行表示一个长度为m的相空间点,一列表示重构后相空间的时间序列长度,将该二维矩阵输入到CNN部分,经过式(3)所示的卷积函数H(x)提取高维特征信息,然后经过池化层选取重要特征,再经过Flatten层将输入压平为一维向量。输出具有高维特征信息的时间序列。
(3)
式中:f和g均为可积函数;x和u为函数中的变量。
2) LSTM部分以CNN的输出时间序列作为输入,传统的RNN在序列较长时容易出现梯度消失或梯度爆炸问题。为了克服长距离记忆带来的问题,LSTM引入门控机制,极大地提高了RNN的数据表达能力。本文中LSTM采取单层结构进行特征的时间序列预测,在模型训练过程中训练数据的不足常常会导致过拟合,为此,本文采用L2参数正则化方法(权重衰减)向目标函数添加一个正则项,通过衰减权重使其更加接近原点。优化目标的权重衰减函数可表达为
(4)
式中:λ为超参数;W为神经网络的链接权重。
3) CNN-LSTM模型训练。首先将数据集划分为训练集和测试集,利用训练数据对LSTM模型进行学习训练,提取重构相空间数据演化的时间特征。训练模型时,需要设置的超参数包括批处理epoch大小和损失函数。优化器的设置包括模型权重和偏置更新方式、学习率。
4) 获得训练好的CNN-LSTM神经网络,输入测试集到模型中,获取预测结果。
5) 判断步骤4)预测结果的准确率,若准确率超过阈值,则执行步骤6);若准确率低于阈值,则返回步骤3),直至模型收敛为止。
6) 利用训练完成的模型预测t+1时刻岩爆演化状态值。
2 基于PSO-GRNN模型的岩爆危险性预测
岩爆危险性预测流程如图2所示,可以分为2个部分。
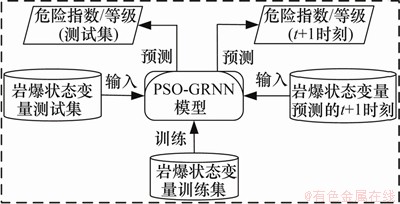
图2 PSO-GRNN模型的岩爆危险等级预测流程图
Fig. 2 Flow chart of rock burst future state and future state risk prediction by PSO-GRNN
第1部分是岩爆危险等级的量化判别,即t+1时刻岩爆的危险性判别。首先,将CNN-LSTM模型得出的特征量未来状态值即t+1时刻岩爆特征量的状态值作为输入;然后,基于粒子群算法优化广义神经网络(PSO-GRNN)模型,回归得t+1时刻岩爆危险等级。
第2部分是未来时刻岩爆危险等级预测。为预测监测变量测试集的岩爆危险等级,将测试集数据作为输入,得到测试集的岩爆危险等级未来状态值,同时将其作为对比实验,验证本文方法在预测岩爆未来状态危险等级的有效性。
本文采用粒子群算法优化广义神经网络模型(PSO-GRNN)。GRNN是一种使用径向基函数作为激活函数的人工神经网络,相较于BP神经网络,具有更强的逼近能力和学习能力,即使在样本数据少的情况下,同样能够很好地识别出岩爆系统的危险性指数,其中,引入粒子群智能优化算法是为获取更加稳定的回归预测模型,采用手工调整方法选取单纯的GRNN模型参数光滑因子spring存在效率低和精度低的问题。光滑因子的确定实质上是一个优化问题,即通过寻找1个最优的光滑因子,使得训练样本的输出值与实际值的均方差最小,由此可以得出最佳光滑因子spring。
粒子群算法是模拟鸟类相互协作寻找事物的智能算法。其求解过程可视为粒子在解空间中寻找最优值,与经典的遗传算法相比较,其能更快收敛于最优解[29]。假定目标优化问题的解为d维向量,粒子群中的每一个粒子都代表一个问题的可能解,通过求出粒子群中每个粒子的适应度F可以对每个结果解进行评价,以得到d维空间中的一个含有最优解的粒子Lbest,由此可以将光滑因子的确定问题转化为通过粒子群算法寻找Lbest。
假定在d维空间中随机散布n个粒子,其位置为Li=(lb1,lb2,…,lbd)(i=1,2,3,…,n),将Li代入函数F(L)并计算其适应度Fi。根据适应度得到第i个粒子在运动过程中所经历过的最优位置Pid=(Pi1,Pi2,…,Pid)、群体中所有粒子经历过的最优位置Gb=(Pg1,Pg2,…,Pgd)(g=1,2,3,…,N),从而各个粒子依据当前粒子所处的位置Li、自身所发现的最优位置Pid以及群体所发现的最优位置Ggb更新个体位置。
初始运行时,粒子群算法需要较大飞行速度以跳过局部最优解。随着迭代次数增加,粒子群在接近最优解时,粒子飞行速度如果过大,会造成粒子很难精确地落在最优解上,为减少粒子在最优解附近的波动,本文增加自适应的惯性因子w,如式(7)。随着迭代次数增加,自适应的惯性因子w逐渐变小,意味着粒子飞行速度降低,从而确保获得最佳光滑因子spring,最终获得更加准确的预测结果。其可描述为:
(5)
(6)
(7)
式中:
为第k次粒子群在寻找食物时粒子i的速度向量;c1和c2均为学习因子;r1和r2均为(0,1)区间随机数;w为惯性因子;Wmax和Wmin分别为惯性因子上界和下界;I和Imax分别为当前迭代数和最大迭代数。模型达到迭代条件后,将以全局中最优位置处粒子为Gb作为目标问题的最优解。
3 实例应用
3.1 数据介绍
基于实际深部矿山岩爆预警工程,数据来源于冬瓜山铜矿微震监测平台。该平台自2005年运行以来不间断地监测微震活动,并进行了大量分析和研究,已经掌握大量矿山微震数据分析技术与利用监测数据进行矿山地压活动分析和评价的方法[30],在此基础上,本文研究重心为矿山岩爆预测预警与危险性识别。本项目监测时间2018-01-01—2018-01-31。监测状态量有震级、震源、能量、角频率、平均位移、地震矩及凹凸体半径等34个字段。特征变量较多,其中有些特征量与岩爆危险性关联程度低,为此,需要剔除这种无关变量。
在微震监测数据的预处理过程中,将少量缺失的微震监测结果根据前后均值法进行弥补,xi=(xi-1+xi+1)/2。此外,监测样本中存在少部分残差大于3σ的异常值,考虑矿山岩爆的特殊环境,这些异常监测值可能是由复杂的地下岩层自然运动活动引发的随机噪声,也可能是由矿井开采过程中产生的小范围崩塌和裂纹引起的正常波动。因此,提出一个控制参数μ以修正少量的残差大于3σ的记录数据(xi=μxi,μ=0.85),保证监测数据时间序列相对的稳定性和预测结果的可靠性。实验中取能量指数lnE,E=Ep+Es,其中,Ep为p波能量,Es为s波能量。lnE反映了岩爆发生时蕴含的能量指数。实验中角频率ω为p波角频率与s波角频率之比,反映了矿山发生岩爆时的震动频率。实验中的凹凸体半径能够从物理层面反映震源及其影响半径,凹凸体和障碍体模型认为矿震产生的原因就是凹凸体破裂使得应力在断层面趋于均匀[31]。
3.2 岩爆危险性标记
综合考虑角频率、能量指数和凹凸体半径等多类岩爆特征量计算每个时刻各变量所反映的岩爆危险性综合指数[32],反映岩爆的危险等级。岩爆综合危险性指数计算主要包括以下步骤。
1) 求单项指标危险系数,首先确定岩爆特征多变量的单项指标危险系数Wi(t),
。其中,A(t)为t时刻监测指标(角频率、能量指数或凹凸体半径)的幅值,
为正常水平下监测指标幅值的平均值,Amax为各指标最大值。
2) 确定单项指标危险系数权重因子并依据该危险系数求解特征多变量的岩爆综合危险系数Wz(t),
,然后由岩爆综合危险指数确定其危险等级。如表1所示,危险等级分成危险、较危险、较安全和安全4类,结合表1可以求出冬瓜山铜矿相应的岩爆危险指数,如表2所示。
表1 变量指数权重和岩爆综合危险指数及危险等级确定表
Table 1 Risk index weight of single variable and risk level of rockburst determination table

3.3 基于相空间重构的岩爆状态值预测
3.3.1 岩爆特征量的相空间重构
本文数据来源于实际工程微震监测数据,具有明显混沌特性。首先对表2中的特征变量角频率、能量指数和凹凸体半径等岩爆状态变量序列进行相空间重构,主要获取嵌入维度m和延迟时间τ这2个参数。使用虚假邻近点法FNN求出特征变量角频率、能量指数和凹凸体半径等序列的嵌入维度m;使用互信息法计算求解状态变量角频率、能量指数和凹凸体半径等序列的延迟时间步长τ。其中,嵌入维度数需满足判据中虚假邻近率最低的要求,由图3可知,角频率的嵌入维度m取4,能量指数及凹凸体半径的嵌入维度m取3;角频率、能量指数及凹凸体半径的延迟时间步长τ取1 s。
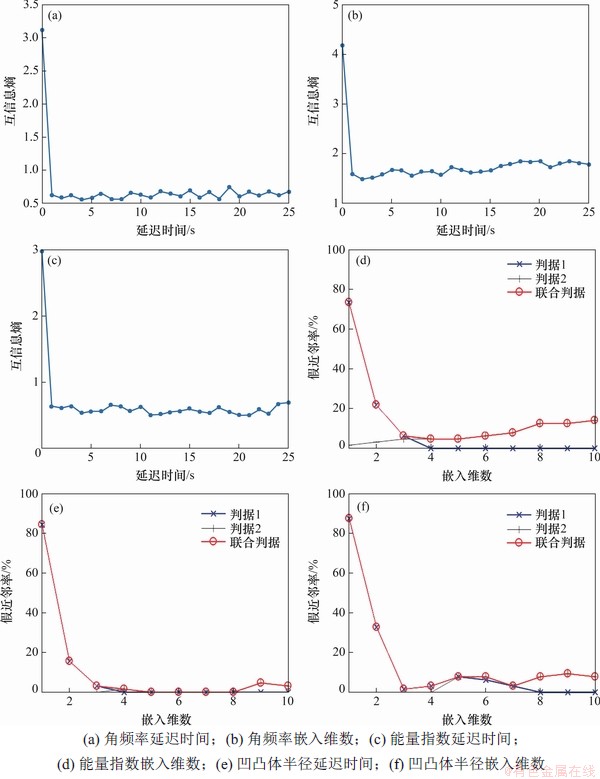
图3 角频率、能量指数及凹凸体半径等状态量的嵌入维度和延迟时间
Fig. 3 Embedding dimension and delay time of angular frequency, energy index and convex body radius
表2 岩爆危险指数确定表(N表示相应变量的归一化值)
Table 2 Rock burst risk index determination table
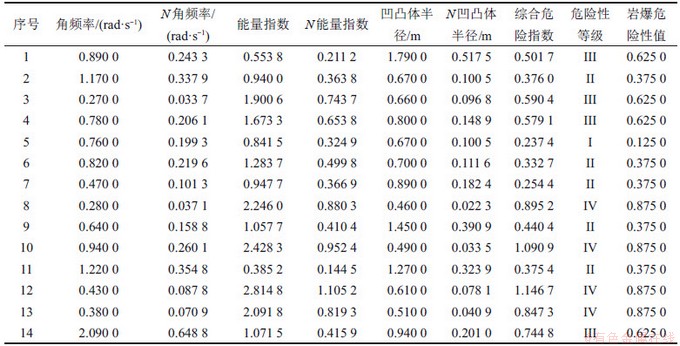
3.3.2 基于CNN-LSTM模型的岩爆特征量预测
本文使用的LSTM采取单层结构,进行特征时间序列预测。神经元数量设为100,超参数λ为0.01。采用CNN-LSTM模型训练,首先按9:1将数据集划分为训练集和测试集,同时利用训练数据对LSTM模型进行学习训练,提取重构相空间数据演化的时间特征。其中,在训练模型过程中,训练时间步长为嵌入维度m,激活函数为Relu,CNN-LSTM模型的优化器选择Adam,参数epoch设置为100,学习率为0.01;实验时间步长取1 h。
首先,获得训练好的LSTM-CNN神经网络,输入测试集到模型中以获取最终预测结果。
然后,评估预测结果的准确性:若准确性小于阈值,则可进行最后一步预测阶段,否则,返回模型训练阶段,直至模型收敛。
最后,利用训练完成的模型进行t+1时刻岩爆演化状态值的预测,获得t+1时刻岩爆各演化状态值预测结果。模型结果评估函数采用经典的均方误差和绝对值误差以衡量结果的准确性及预测误差,CNN-LSTM模型及其他方法的对比预测结果如表3所示。
由表3可知:在利用不同方法进行上述岩爆状态预测场景中,本模型预测误差更小,稳定性更好,说明在预测岩爆演化状态中,针对其具有的混沌特性,在还原原始空间之后,使用本模型预测可获得更好效果。
3.4 基于PSO-GRNN模型的岩爆危险性预测
本文基于改进的PSO-GRNN模型,结合多特征量以综合反映岩爆危险等级。为验证本文方法有效性,设置2组对比实验,其中将PSO-GRNN模型对测试集反映岩爆危险等级的过程称为岩爆危险等级判别,而将PSO-GRNN模型对由CNN-LSTM模型得到的特征预测值反映岩爆未来时刻危险等级的过程称为岩爆的危险等级预测。
3.4.1 岩爆危险等级判别
岩爆危险性判别过程并结合角频率、能量指数和凹凸体半径3个微震监测特征变量,综合反映岩爆危险等级。为充分利用数据获得最优模型,实验采用四折交叉验证方法,训练集和测试集按9:1的比例划分。本算法参数设置如下:粒子数目为10,学习因子为0.2,惯性权重为0.7,迭代停止条件为循环步数大于100或训练误差低于0.001 0,以所得的权值阈值为初始值,用GRNN算法训练网络。
图4所示为适应度函数优化曲线和光滑因子优化曲线。采用单步预测,预测值与测试值的均方误差作为适应度。适应度越小,则反映学习数据时模型的训练效果越好,获得最优适应度为0.001 4。粒子群在寻找最优光滑因子时常陷入局部最优解,本文提出的改进粒子群算法能有效地寻找到全局最优解。
由图4(b)可见:本方法借鉴鸟群接近食物时自适应地降低寻找飞行速度,能有效地避免错过最优解;在迭代到19次时,出现一次较大的上升趋势,可以理解为粒子群跳出了局部解,最优光滑因子表示为模型学习数据训练时获得最优,最终获得最优光滑因子为0.084 9。使用优化后的广义神经网络,根据角频率、能量指数和凹凸体半径等特征量判别岩爆目前状态的危险等级,结果如表4所示。
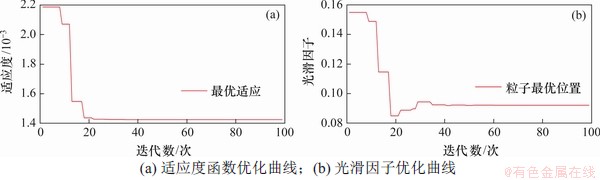
图4 适应度函数优化曲线和光滑因子优化曲线
Fig. 4 Fitness function optimization curve and Spring optimization curve
3.4.2 岩爆危险等级预测
为预测未来状态值的岩爆等级,首先利用CNN-LSTM模型预测出特征量的未来状态值,进而使用PSO-GRNN模型预测特征量的未来状态值即t+1时刻岩爆危险等级。为保持方法一致性,训练集和验证集不变,但是,预测数据集由预测的岩爆特征量的未来状态值构成,并采用四折交叉验证,参数设置相同。
表3 冬瓜山铜矿岩爆未来状态量CNN-LSTM模型和其他方法预测结果
Table 3 CNN-LSTM model and other methods for predicting the future state of rock burst in Dongguashan copper mine
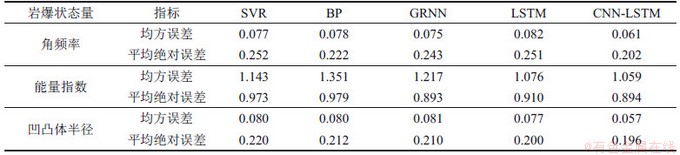
表4 冬瓜山铜矿岩爆特征量未来状态值下的岩爆等级预测结果
Table 4 Prediction results of rockburst grade according the future state value of rockburst characteristic variables in Dongguashan Copper Mine
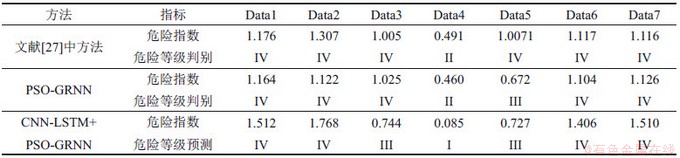
图5所示为未来状态下适应度函数优化曲线和未来状态下光滑因子优化曲线。由图5(a)可见:采用单步预测,预测值与测试值的均方误差作为适应度,获得最优适应度为0.018 4。适应度越小,表明学习数据时模型的训练越好。
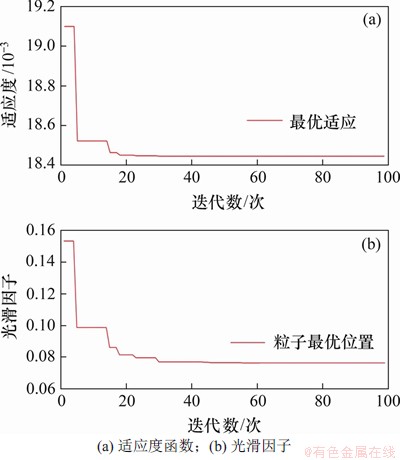
图5 未来状态下适应度函数优化曲线和未来状态下光滑因子优化曲线
Fig. 5 Fitness function with future state of rock burst optimization curve and Spring with future state of rock burst optimization curve
由图5(b)可见:粒子群得到的最优光滑因子为0.076,与上述实验结果接近,表明算法具有一定鲁棒性。最后,本文采用优化的GRNN模型预测综合多岩爆特征量的未来时刻岩爆危险等级,结果如表4所示。
由表4可知:结合冬瓜山铜矿的实际微震监测工程,考虑深部矿井硬岩所蕴含地应力的非线性动态变化过程,在实时的微震监测数据基础上,本文方法不仅能够准确地拟合岩爆和冲击地压演化状态,把握硬岩失稳前后的过程,同时能实现硬岩岩爆和冲击地压的危险等级预测及岩爆特征量的未来状态值岩爆危险等级预测。由表4所示结果可知岩爆特征量的未来状态值危险等级预测准确性较高,研究成果对于及时掌握矿山岩爆活动未来状态提供重要依据,为准确识别矿山岩爆当前活动及未来状态的危险性提供参考依据。
4 结论
1) 本文提出了一种基于混沌性时间序列的CNN-LSTM深度学习模型岩爆状态量预测的方法,针对于岩爆状态量演化过程的混沌性,将状态量进行相空间重构还原原始系统,并通过卷积神经网络有效地抽取其时空特征,将抽取及扁平化后的时序数据作为LSTM模型的输入,预测未来时刻岩爆状态数值。
2) 提出了一种集成方法预测岩爆特征量的未来状态下的危险等级,将岩爆特征量的未来状态值与PSO-GRNN模型判别的岩爆未来危险等级相结合,实现矿山岩爆活动的动态预测。同时,本文综合考虑多类岩爆特征量,如角频率、能量指数和凹凸体半径等,综合反映岩爆的危险等级,突破了以单一特征量评价岩爆状态及等级导致的精度不高的局限。
3) 本文提出的集成方法不仅适用于微震监测数据,而且可为包括声发射、电磁辐射和红外辐射等矿山岩爆或冲击地压监测数据的时序预测开拓思路,并能够为深井硬岩类似岩体工程的预测预警提供指导。
参考文献:
[1] 何满潮, 谢和平, 彭苏萍, 等. 深部开采岩体力学研究[J]. 岩石力学与工程学报, 2005, 24(16): 2803-2813.
HE Manchao, XIE Heping, PENG Suping, et al. Study on rock mechanics in deep mining engineering[J]. Chinese Journal of Rock Mechanics and Engineering, 2005, 24(16): 2803-2813.
[2] WANG J A, PARK H D. Comprehensive prediction of rockburst based on analysis of strain energy in rocks[J]. Tunnelling and Underground Space Technology, 2001, 16(1): 49-57.
[3] 唐礼忠, 潘长良, 杨承祥, 等. 冬瓜山铜矿微震监测系统及其应用研究[J]. 金属矿山, 2006(10): 41-44.
TANG Lizhong, PAN Changliang, YANG Chengxiang, et al. Establishment and application of microseismicity monitoring system in Dongguashan copper mine[J]. Metal Mine, 2006(10): 41-44.
[4] 周辉, 杨凡杰, 张传庆, 等. 岩爆和冲击地压数值模拟与评估预测方法[M]. 北京: 科学出版社, 2015: 14-81.
ZHOU Hui, YANG Fanjie, ZHANG Chuanqing, et al.Numerical simulation and evaluation of rock burst and rock burst pressure[M]. Beijing: Science Press, 2015: 14-81.
[5] 赵洪波, 茹忠亮. 冲击地压预测的PSO-SVM模型[J]. 岩石力学与工程学报, 2007, 26(S1): 3479-3483.
ZHAO Hongbo, RU Zhongliang. PSO-SVM model for prediction of rock burst[J]. Chinese Journal of Rock Mechanics and Engineering, 2007, 26(S1): 3479-3483.
[6] 谭云亮, 孙中辉, 杜学东. 冲击地压AE时间序列小波神经网络预测模型[J]. 岩石力学与工程学报, 2000, 19(S1): 1034-1036.
TAN Yunliang, SUN Zhonghui, DU Xuedong. A wavelet neural network predicting model for acoustic emission time series of rock burst[J]. Chinese Journal of Rock Mechanics and Engineering, 2000, 19(S1): 1034-1036.
[7] 张艳博, 杨震, 姚旭龙, 等. 基于声发射信号聚类分析和神经网络识别的岩爆预警方法实验研究[J]. 岩土力学, 2017, 38(S2): 89-98.
ZHANG Yanbo, YANG Zhen, YAO Xulong, et al. Experimental study of rockburst early warning method based on acoustic emission cluster analysis and neural network identification[J]. Rock and Soil Mechanics, 2017, 38(S2): 89-98.
[8] FRID V, VOZOFF K. Electromagnetic radiation induced by mining rock failure[J]. International Journal of Coal Geology, 2005, 64(1/2): 57-65.
[9] 马天辉, 唐春安, 唐烈先, 等. 基于微震监测技术的岩爆预测机制研究[J]. 岩石力学与工程学报, 2016, 35(3): 470-483.
MA Tianhui, TANG Chun'an, TANG Liexian, et al. Mechanism of rock burst forcasting based on micro-seismic monitoring technology[J]. Chinese Journal of Rock Mechanics and Engineering, 2016, 35(3): 470-483.
[10] 李长洪, 张立新, 张磊, 等. 灰色突变理论及声发射在岩爆预测中的应用[J]. 中国矿业, 2008, 17(8): 87-90.
LI Changhong, ZHANG Lixin, ZHANG Lei, et al. Application of grey catastrophe theory and acoustic emission in rock burst prediction[J]. China Mining Magazine, 2008, 17(8): 87-90.
[11] FRID V. Calculation of electromagnetic radiation criterion for rockburst hazard forecast in coal mines[J]. Pure and Applied Geophysics, 2001, 158(5/6): 931-944.
[12] 彭琦, 张茹, 谢和平, 等. 基于AE时间序列的岩爆预测模型[J]. 岩土力学, 2009, 30(5): 1436-1440.
PENG Qi, ZHANG Ru, XIE Heping, et al. Prediction model for rockburst based on acoustic emission time series[J]. Rock and Soil Mechanics, 2009, 30(5): 1436-1440.
[13] XIE Heping, CHEN Zhida. Fractal geometry and fracture of rock[J]. Acta Mechanica Sinica, 1988, 4(3): 255-264.
[14] 黄滚, 尹光志. 冲击地压粘滑失稳的混沌特性[J]. 重庆大学学报, 2009, 32(6): 633-637.
HUANG Gun, YIN Guangzhi. Chaotic behavior of stick slip in rock bursts[J]. Journal of Chongqing University, 2009, 32(6): 633-637.
[15] 陶慧, 马小平, 乔美英. 基于多变量混沌时间序列的冲击地压预测[J]. 煤炭学报, 2012, 37(10): 1624-1629.
TAO Hui, MA Xiaoping, QIAO Meiying. Rock burst prediction on multivariate chaotic time series[J]. Journal of China Coal Society, 2012, 37(10): 1624-1629.
[16] 白云飞, 邓建, 董陇军, 等. 深部硬岩岩爆预测的FDA模型及其应用[J]. 中南大学学报(自然科学版), 2009, 40(5): 1417-1422.
BAI Yunfei, DENG Jian, DONG Longjun, et al. Fisher discriminant analysis model of rock burst prediction and its application in deep hard rock engineering[J]. Journal of Central South University(Science and Technology), 2009, 40(5): 1417-1422.
[17] 贾义鹏, 吕庆, 尚岳全. 基于粒子群算法和广义回归神经网络的岩爆预测[J]. 岩石力学与工程学报, 2013, 32(2): 343-348.
JIA Yipeng, LU Qing, SHANG Yuequan. Rockburst prediction using particle swarm optimization algorithm and general regression neural network[J]. Chinese Journal of Rock Mechanics and Engineering, 2013, 32(2): 343-348.
[18] 张光存, 高谦, 杜聚强, 等. 基于人工神经网络及非线性回归的岩爆判据[J]. 中南大学学报(自然科学版), 2013, 44(7): 2977-2981.
ZHANG Guangcun, GAO Qian, DU Juqiang, et al. Rockburst criterion based on artificial neural networks and nonlinear regression[J]. Journal of Central South University(Science and Technology), 2013, 44(7): 2977-2981.
[19] 周辉, 杨凡杰, 张传庆. 岩爆和冲击地压数值模拟与评估预测方法[M]. 北京: 科学出版社, 2015: 206-243.
ZHOU Hui, YANG Fanjie, ZHANG Chuanqing, et al. Numerical simulation and evaluation of rock burst and rock burst pressure[M]. Beijing: Science Press, 2015: 206-243.
[20] 李宁, 王李管, 贾明涛. 基于粗糙集理论和支持向量机的岩爆预测[J]. 中南大学学报(自然科学版), 2017, 48(5): 1268-1275.
LI Ning, WANG Liguan, JIA Mingtao. Rockburst prediction based on rough set theory and support vector machine[J]. Journal of Central South University(Science and Technology), 2017, 48(5): 1268-1275.
[21] 张春涛, 马千里, 彭宏, 等. 基于条件熵扩维的多变量混沌时间序列相空间重构[J]. 物理学报, 2011, 60(2): 118-125.
ZHANG Chuntao, MA Qianli, PENG Hong, et al. Multivariate chaotic time series phase space reconstruction based on extending dimension by conditional entropy[J]. Acta Physica Sinica, 2011, 60(2): 118-125.
[22] 宋维源, 潘一山, 苏荣华, 等. 冲击地压的混沌学模型及预测预报[J]. 煤炭学报, 2001, 26(1): 26-30.
SONG Weiyuan, PAN Yishan, SU Ronghua, et al. Forecast and chaos model of rockburst[J]. Journal of China Coal Society, 2001, 26(1): 26-30.
[23] GREFF K, SRIVASTAVA R K, KOUTNIK J, et al. LSTM: a search space odyssey[J]. IEEE Transactions on Neural Networks and Learning Systems, 2017, 28(10): 2222-2232.
[24] 李伟山, 王琳, 卫晨. LSTM在煤矿瓦斯预测预警系统中的应用与设计[J]. 西安科技大学学报, 2018, 38(6): 1027-1035.
LI Weishan, WANG Lin, WEI Chen. Application and design of LSTM in coal mine gas prediction and warning system[J]. Journal of Xi'an University of Science and Technology, 2018, 38(6): 1027-1035.
[25] 李明晓, 张恒才, 仇培元, 等. 一种基于模糊长短期神经网络的移动对象轨迹预测算法[J]. 测绘学报, 2018, 47(12): 1660-1669.
LI Mingxiao, ZHANG Hengcai, QIU Peiyuan, et al. Predicting future locations with deep fuzzy-LSTM network[J]. Acta Geodaetica et Cartographica Sinica, 2018, 47(12): 1660-1669.
[26] GERS F A, SCHRAUDOLPH N N, SCHMIDHUBER J. Learning precise timing with LSTM recurrent networks[J]. Journal of machine learning research, 2002, 3(1): 115-143.
[27] SONG Shengli, HUANG Haitao, RUAN Tongxiao. Abstractive text summarization using LSTM-CNN based deep learning[J]. Multimedia Tools and Applications, 2019, 78(1): 857-875.
[28] GRAVES A, MOHAMED A R, HINTON G. Speech recognition with deep recurrent neural networks[C]// 2013 IEEE International Conference on Acoustics, Speech and Signal Processing. 2013, Vancouver, BC, Canada: IEEE, 2013: 6645-6649.
[29] ZHAN Zhihui, ZHANG Jun, LI Yun, et al. Adaptive particle swarm optimization[J]. IEEE Transactions on Systems, Man, and Cybernetics, Part B (Cybernetics), 2009, 39(6): 1362-1381.
[30] 陈资南. 冬瓜山铜矿微震监测系统扩展与矿震研究[D]. 长沙: 中南大学资源与安全工程学院, 2014: 11-52.
CHEN Zinan. Expansion of microseismic monitoring system and study on mine seismic in Dongguashan copper mine[D]. Changsha: School of Resources and Safety Engineering. Central South University, 2014: 11-52.
[31] 唐礼忠, 汪令辉, 张君, 等. 大规模开采矿山地震视应力和变形与区域性危险地震预测[J]. 岩石力学与工程学报, 2011, 30(6): 1168-1178.
TANG Lizhong, WANG Linghui, ZHANG Jun, et al. Seismic apparent stress and deformation in a deep mine under large-scale mining and areal hazardous seismic prediction[J]. Chinese Journal of Rock Mechanics and Engineering, 2011, 30(6): 1168-1178.
[32] 陈国祥, 窦林名, 曹安业, 等. 电磁辐射法评定冲击矿压危险等级及应用[J]. 煤炭学报, 2008, 33(8): 866-870.
CHEN Guoxiang, DOU Linming, CAO Anye, et al. Assessment of rock burst danger and application on electromagnetic emission method[J]. Journal of China Coal Society, 2008, 33(8): 866-870.
(编辑 秦明阳)
收稿日期: 2020 -04 -26; 修回日期: 2020 -06 -10
基金项目(Foundation item):国家自然科学基金重点资助项目(41730105);湖南省重点研发计划项目(2018SK2052);中南大学研究生自主探索创新项目(2019zzts647,2018zzts198) (Project(41730105) supported by the National Natural Science Foundation of China; Project(2018SK2052) supported by the Key Research and Development Program of Hunan Province; Projects(2019zzts647, 2018zzts198) supported by the Fundamental Research Funds of Central South University)
通信作者:刘宝举,博士,从事时空大数据挖掘和岩土工程研究;E-mail: baojuliu@csu.edu.cn
引用格式: 刘慧敏, 徐方远, 刘宝举, 等. 基于CNN-LSTM的岩爆危险等级时序预测方法[J]. 中南大学学报(自然科学版), 2021, 52(3): 659-670.
Citation: LIU Huimin, XU Fangyuan, LIU Baoju, et al. Time-series prediction method for risk level of rockburst disaster based on CNN-LSTM[J]. Journal of Central South University(Science and Technology), 2021, 52(3): 659-670.