J. Cent. South Univ. (2016) 23: 1778-1785
DOI: 10.1007/s11771-016-3231-9

Vehicular emissions and concentrations in school zones: A case study
ALZUHAIRI Ali1, ALDHAHERI Mustafa1, SUN Zhan-bo(孙湛博)1, 2, OH Jun-Seok1, KWIGIZILE Valerian1
1. Department of Civil and Construction Engineering, Western Michigan University, Kalamazoo, 49008-5316 MI, USA;
2. School of Transportation and Logistics, Southwest Jiaotong University, Chengdu 610031, China
Central South University Press and Springer-Verlag Berlin Heidelberg 2016
Abstract: Recent research has revealed that human exposure to air pollutants such as CO, NOX, and particulates can lead to respiratory diseases, especially among school-age children. Towards understanding such health impacts, this work estimates local-scale vehicular emissions and concentrations near a highway traffic network, where a school zone is located in. In the case study, VISSIM traffic micro-simulation is used to estimate the source of vehicular emissions at each roadway segment. The local-scale emission sources are then used as inputs to the California line source dispersion model (CALINE4) to estimate concentrations across the study area. To justify the local-scale emissions modeling approach, the simulation experiment is conducted under various traffic conditions. Different meteorological conditions are considered for emission dispersion. The work reveals that emission concentrations are usually higher at locations closer to the congested segments, freeway ramps and major arterial intersections. Compared to the macroscopic estimation (i.e. using network-average emission factors), the results show significantly different emission patterns when the local-scale emission modeling approach is used. In particular, it is found that the macroscopic approach over-estimates emission concentrations at freeways and under-estimations are observed at arterials and local streets. The results of the study can be used to compare to the US environmental protection agency (EPA) standards or any other air quality standard to further identify health risk in a fine-grained manner.
Key words: human health; vehicular emissions; VISSIM microscopic simulation; California line source dispersion model (CALINE4); local-scale modeling
1 Introduction
Traffic on roads has dramatically increased in the U.S. and elsewhere over the last few decades. As the level of congestion and duration of congestion keep going up, vehicular emissions and concentrations also increase accordingly, especially near congested freeways and arterials. Vehicular emissions, including carbon monoxide (CO), carbon dioxide (CO2), volatile organic compounds (VOCs) or hydrocarbons (HCs), nitrogen oxides (NOX), particulate matters (PMs) and among others have been attributed to the major source of air pollutants [1]. Recent research has revealed that long time exposure to air pollutants can cause short-term health problems, such as headaches, nausea, skin and eye irritation, nose, throat, and lung inflammation; and long-term respiratory and cardiovascular health problems, such as asthma and heart disease [2]. SABIN et al [3] reported that people who spend long time close to major roads are prone to health issues. And evidences showed that such health impacts are particularly significant on children. According to Ref. [3], children are particularly vulnerable to airborne pollution because of their narrower airways and because they breathe more air per pound of body weight than adults, which has increased their exposure to air pollutants. Despite the findings, there has been comparatively little work done in analyzing the health impacts on school-age children in school zones.
To assess such health impacts, the current practice is to compare the emission concentrations collected by community-wide monitors to the air quality standards, such as US Environmental Protection Agency (EPA) Air Quality Standards. For example, according to the latest EPA’s primary health-related standards [4], the concentration of carbon monoxide should not exceed 9×10-6 (9 ppm) and 35×10-6 (35 ppm), for one-hour period and eight-hour period, respectively. Despite the reductions in ambient carbon monoxide concentrations over the past decade, it was reported by WITTENBERG and WITTENBERG [5] that approximately 20 million people live in areas of the United States that exceed the carbon monoxide standard.
The current state of the practice for air quality evaluation heavily relies on the emission concentration information collected by fixed-location air quality monitors. These fixed-location measures, however, are usually restricted to very few and sparse locations, which cannot be used to identify the emission hot spots, e.g., near major roadways. SABIN et al [3] showed that near road (within about 50 m) concentrations of NO2 have been measured to be approximately 30% to 100% percent higher than concentrations away from major roads. And in-vehicle concentrations could be even higher [6]. Due to these reasons, we consider a more robust yet cost-effective two-step approach to estimate emission concentrations close to highways.
The first step is to estimate the source of emissions, which is a critical task since the source of emissions may vary drastically across the network. Existing literature showed that highway class, traffic control, and traffic flow characteristics can significantly affect the amount of emissions on roadways. ABOU-SENNA et al [7] showed that a large amount of vehicular emissions occurs at speed equal and less than 32 km/h. It was reported that the behavior of the driver, his position in the queue, lane volume, and posted link speed are the factors that can significantly influence the emission rates [8-10]. Frequent acceleration and deceleration movements on the link were found to have significant impacts on the total emissions [11-12]. To capture these variations, an intuitive approach is to decompose the traffic network into homogeneous areas such as road segments and estimate the source of emissions for each road segment. In this work, we refer to this as a local-scale emissions estimation approach.
Based on the estimated emission factors, the second step is to model how these pollutants spread away from the highway source. This task is known as emission dispersion modeling. Various types of emission dispersion models have been developed in the atmospheric environment field, including Gaussian plume model [13], box model [14], Lagrangian model [15], and others [16]. Among these models, Gaussian dispersion model is the most commonly used one due to its simplistic nature. To date, Gaussian model-based software packages such as CALINE4, HIWAY2 have been widely adopted to estimate emission concentrations and exposures near freeways and major arterials [17-19].
The aforementioned two-step approach is used in a case study to analyze vehicular emissions and concentrations near a highway traffic network, where a school zone is located in. In the first step, road-level emissions are estimated using VISSIM traffic simulation, which adopts TNO’s (the Netherlands Organization for Applied Scientific Research) VERSIT+ emissions model for emissions estimation [20]. Note that in the cases that vehicle characteristics and operational status (e.g., time-speed profiles [12]) are available, road-level emissions can be estimated more accurately using modal-based emissions models, such as the comprehensive modal emissions model (CMEM) and EPA’s motor vehicle emission simulator (MOVES). However, the modal-based emissions models are not used in this work mainly due to the lack of vehicle characteristic and operational information. In the second step, the estimated emission rates are directly used as the input to CALINE4, to estimate local-scale emission concentrations at 15 selected points within the study area. Various traffic conditions and meteorological parameters (direction of wind, wind speed) are considered in the work to account for the temporal variation of emission source and meteorological conditions.
2 Methodology
To analyze the health impacts of emissions, current practices heavily rely on the emission concentration data collected at fixed-locations. These data collection sites, however, are usually located far away from traffic hotspots and cannot be used to describe emission and its spatial variation in a fine-grained manner. To address this issue, a local-scale approach is proposed in this work.
2.1 Estimate source of emissions
In the first step, the source of emissions is estimated for each road segment using VISSIM traffic simulation. The reason of using micro-simulation in this work is mainly for demonstration purposes. More accurate emission models (e.g., CMEM or MOVES) can be applied in the cases that detailed operational status (e.g., time-speed profiles) and vehicle characteristics are available. VISSIM traffic simulation (Version 7) adopts TNO’s (the Netherlands Organization for Applied Scientific Research) VERSIT+ emissions model, which is a macroscopic emission model fitted using empirical data from approximately 12000 emission tests [21]. The model is able to estimate road-level emissions as a function of vehicle parameters, time-speed profile, vehicle miles traveled (VMT), among other factors.
The road-level emissions and VMT obtained from VISSIM simulation are used to calculate the emission factors for each road segment, and each time interval, as denoted in Eq. (1). Where Ek,t is the estimated emission for each segment k, during time interval t; Qk, t is the traffic volume observed in simulation; Lk is the length of the segment; and fk, t is the calculated emission factor, in grams per VMT.
(1)
To compare the local-scale approach and the conventionally used macroscopic approach, we calculate the network-wide average emission factors, fAVE, t, using Eq. (2).
(2)
The emission factors, along with the segment traffic volumes are directly used as the inputs to the emission dispersion model, as described in the subsequent section.
2.2 Emission dispersion modeling
The California line source dispersion model (CALINE4) is used to simulate emission dispersion and estimate the concentration levels within the traffic network. CALINE4 assumes that each road segment can be divided into a few small elements, and each element is treated as a homogeneous line source perpendicular to the wind direction. The emission concentration level at a receptor location can be calculated using the finite line source Gaussian dispersion model for each homogeneous line source. Modeling details can be referred to Ref. [4].
The main input data for CALINE4 include: 1) link activity include traffic volume and emission factors for each road segment; 2) link geometry include link type, and the length of each link; 3) run conditions such as wind speed, wind direction, wind direction, atmospheric stability, mixing height, aerodynamic roughness, ambient temperature, among others; and 4) receptor positions. Given the input information, CALINE4 is able to simulate the emission dispersion process and estimate emission concentrations (CO and NOX) at selected receptor locations in the study area.
3 Site description and simulation
In the case study, a real traffic network was selected and used to demonstrate the proposed local-scale emissions modeling approach. The selected network is in Chicago IL, where an elementary school is located in. This site has been chosen for two main reasons: 1) the school zone is right next to the I-290 freeway, children are expected to spend more than 8 hours in the school; 2) High traffic volumes are observed on the freeway and adjacent arterials during the school days. In addition to the school zone, a residential zone also locates in the study area, where a significant amount of home-based trips is generated.
The network is comprised of a segment of I-290 freeway, two major arterials, three collectors, in addition to a few one-way local streets. The segment of freeway has 3-4 lanes in each direction, with a peak hour volume of 12875 vehicles per hour for both directions. The variations of freeway hourly traffic volumes are shown in Fig. 1. The network was coded in VISSIM simulation software, as in Fig. 2(a). A streamlined model calibration and validation procedure [22] was adopted to fine-tune the simulation parameters so that the simulation can reflect the real world situations in a realistic manner, details are not presented here. To better understand the emission patterns under various traffic conditions, three scenarios were considered and tested on the simulation network. Eight-hour simulation runs were performed for each scenario, and road level emissions factors (CO and NOX) were then collected.
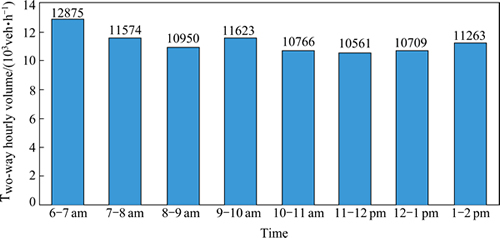
Fig. 1 School day hourly traffic volumes on I-290 (06:00 am– 02:00 pm)
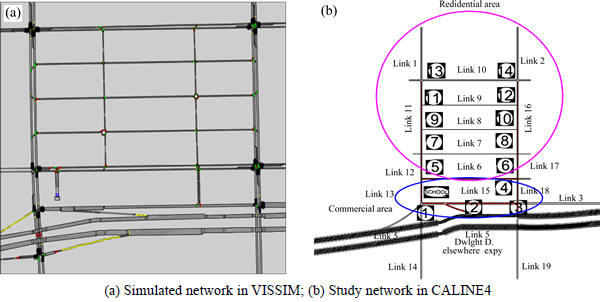
Fig. 2 Study network:
3.1 Scenario A: Normal traffic condition
This scenario considers eight-hour traffic (6:00 am to 2:00 pm) during a normal school day, including a two-hour peak period. The traffic volume information was obtained from Illinois Department of Transportation, U.S.A [23].
3.2 Scenario B: Demand surge
This scenario considers an imaginary demand surge situation (e.g., due to school-related events), in which the traffic volumes on school-related origin-destination (OD) pairs increased by 20%. Significant stop-and-go traffic behavior and large travel time delay were observed in simulation.
3.3 Scenario C: Lane closure
It simulates an imaginary scenario with one-lane closed on the freeway segment (e.g., due to traffic accident). The traffic volumes in the normal condition were used in this scenario. In the case study, the authors also examined the impacts of different meteorological conditions by considering various combinations of wind speed (0.5 m/s, 1.0 m/s, up to 2.0 m/s) and wind direction (0, i.e., wind blowing from the north, 15°, 30°, up to 345°). Eight-hour emission dispersion simulations were conducted and the emission concentration levels (CO and NOX) were then collected at 15 receptor points in the network. The receptor points were symmetrically deployed in the network to capture the spatial variation of the emission concentration pattern. As shown in Fig. 2(b), in addition to the receptor point at the school location, there are three receptor points (1-3) adjacent to the freeway, which are located in a commercial area; eleven points (4-15) within a residential area. Other parameters in CALINE4 were set using default/standard values, details are not presented here.
4 Numerical results
The simulated traffic volume and collected emission factors are illustrated in Table 1. Column 3 represents the peak hour traffic volume for each segment; column 4 and column 5 indicate the estimated road level emissions (CO and NOX); column 6 and column 7 are the emissions factors calculated using Eqs. (1) and (2). As listed in Table 1, although freeway segments have higher traffic volumes compared to local and arterial segments, they usually correspond to smaller emission factors (in grams/vehicle-mile). This is mainly due to the large turbulences with local and arterial traffic, such as stop-and-go behavior at traffic signals. To compare the local-scale approach with the conventionally used macroscopic approach, we also considered the network-average emission factors. As shown later in the work, it is found that compared to the macroscopic approach, the local-scale approach yields significantly different emission patterns.
Table 1 Peak hour volume and emission rates in Vissim (Scenario A)
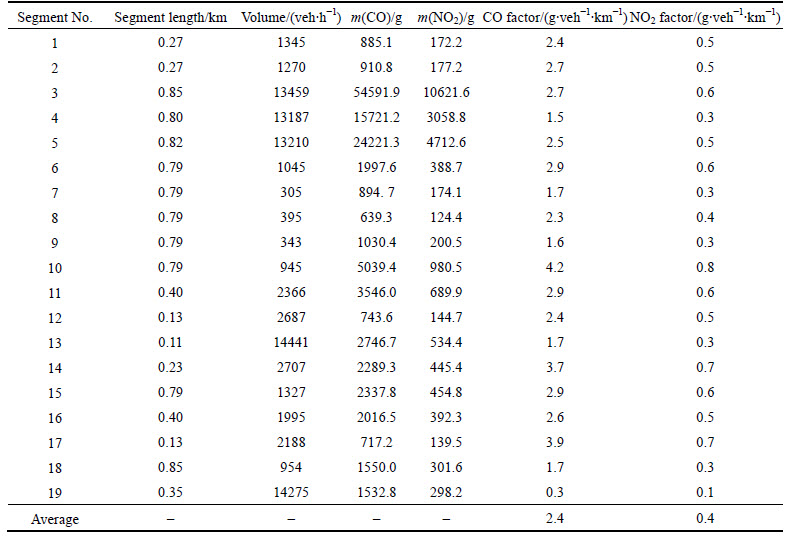
The collected traffic volumes and emission factors were then used as the input information to CALINE4, and the emission concentration levels were collected at the 15 receptor points. In Figs. 3 and 4, we first show that the concentration levels at the school location and the worst location (point 3) in the network, under different wind speed and wind direction.
Figures 3 and 4 indicate the emission concentration levels in Scenario A under different meteorological conditions. It is observed that the concentration levels are usually higher when wind speed is low. The dispersion of air pollutants is low under this condition. With respect to the school zone, the highest concentrations occur when the direction of wind is in the range of 210°-240° (0 indicates wind blowing from the north, increasing clockwise), blowing from the southwest. That is, the worst case occurs when the school zone is at a downwind location adjacent to the freeway and arterials segments (Link 5, Link 13, and Link 14). In this case, the estimated CO concentration is about 1×10-6 (1 ppm), which is less than the EPA standard (9×10-6, 9 ppm). The estimated NO2 concentration is 0.2×10-6, 0.2 ppm; however, it is larger than the EPA standard (0.15×10-6, 0.15 ppm), which is considered to be detrimental to human health. The highest concentration is at point 3, which is close to a few freeway segments and major arterials. As shown in Figu. 4, the estimated CO concentration is still smaller than the EPA standard. However, the CO2 concentration is more than twice the EPA standard in the worst case, when wind blows from the east (90°).
The worst-case network-wide emission concentrations are shown in 3D plots in Fig. 5; where x-axis stands for the north-south dimension and y-axis represents the east-west dimension of the study area. It is found that emission concentration levels are usually higher at the area that is close to the freeway segment (i.e. x close to zero). Compared to Scenario A, the CO concentrations in the demand surge and lane closure scenarios (i.e. Figs. 5(c) and 5(e)) are consistently higher. The air quality is poor and can be even considered detrimental (close to the 9×10-6 (9 ppm) EPA standard) in the area close to the freeway segments. With respect to the NO2 mass fraction, similar trends are revealed in the NO2 plots (i.e., Figs 5(b), 5(d), and 5(f)); the increments in NO2 are not as significant as CO. In all the three scenarios, the estimated NO2 concentrations close to the freeway segments (including the school zone) are higher than the 0.15×10-6 (0.15 ppm) EPA standard.
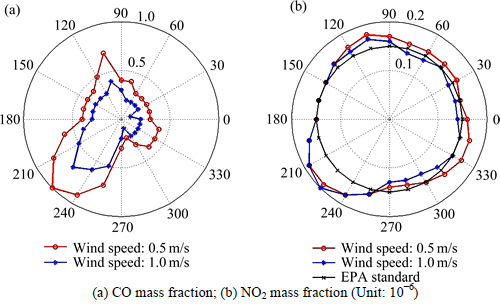
Fig. 3 Scenario A (Emission concentrations at school zone):
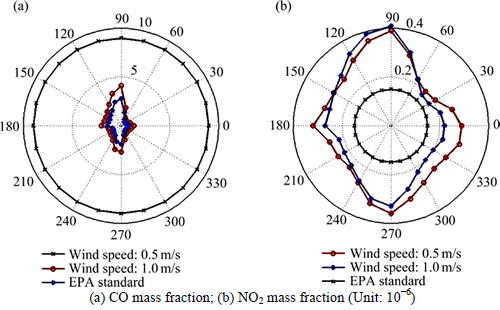
Fig. 4 Scenario A (Emission concentrations at Point 3):
The comparisons between the local-scale approach and the macroscopic approach (network-average) are presented in Fig. 6. It can be observed that the emission concentration patterns estimated by these two approaches are significantly different. In particular, it is found that the macroscopic approach over-estimates the emission concentrations near freeway segments, where the emission factors are over-estimated by the network- average; and it under-estimates the mass fraction levels near arterial and local streets, where the emission factors are under-estimated by the network-average.
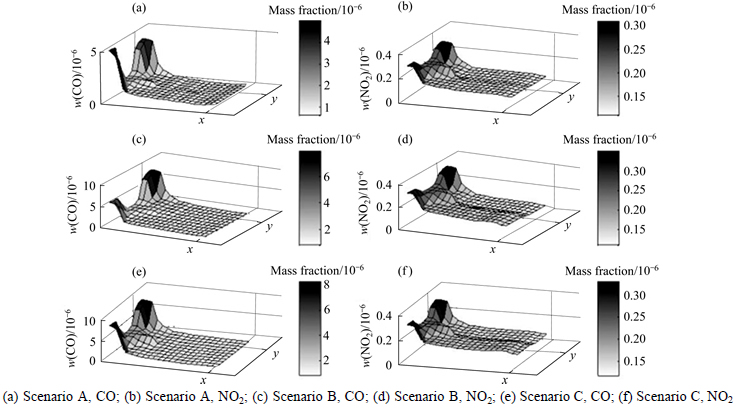
Fig. 5 3D contours of emission concentrations:
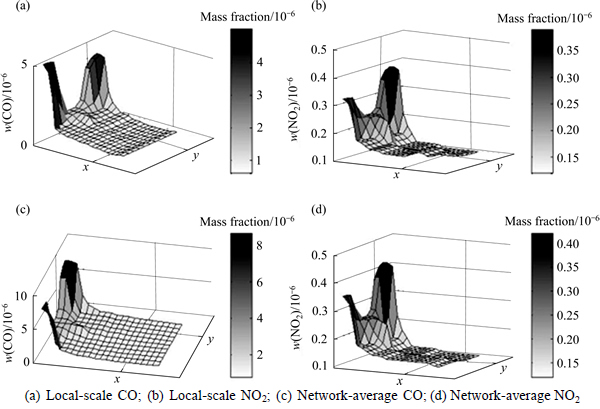
Fig. 6 3D contours of emission concentration of Scenario A:
5 Conclusions
1) This work proposes a two-step local-scale approach to estimate vehicular emissions concentrations in a school zone area. In the first step, we decomposes the traffic network into homogeneous road segments and estimate the source of emissions on each segment. In the second step, the estimated emission rates are directly used as the input to CALINE4, to estimate local-scale emission concentrations at 15 selected points within the study area. Various traffic conditions and meteorological parameters are considered in the work to account for the temporal variation of emission source and meteorological conditions such as different wind speeds and wind directions.
2) The method is able to capture the variations of emission patterns in a fine-grained manner. The results reveal that emission concentrations are usually higher at locations closer to the congest links (e.g., freeway segments). The authors also observed significantly different emission patterns between the local-scale estimation and a macroscopic estimation (using network-average emission factors). In particular, we find that the macroscopic approach tends to over-estimate emission concentrations at freeways and under-estimate emission concentrations at arterials and local streets. Since there is a lack of field collected traffic and emission concentration data, we are not able to directly justify that the proposed local-scale approach yields more accurate results. However, the author believes that in the cases that the source of emissions can be accurately estimated (e.g., based on the actual traffic condition and detailed driving states), the local-scale approach should provide more reliable emission concentration results. Further experiments and justifications will be provided in the future research.
3) The estimates from the study can be used to compare to the EPA standard or any other air quality standard to identify the health risk within the school zones or other areas. This information can assist city planners in making informed decisions (e.g., land use development), and it can also provide valuable trip making suggestions to the impacted groups (e.g., ask asthmatic children to stay indoor).
References
[1] US National Research Council. The congestion mitigation and air quality improvement program: Assessing 10 years of experience [M]. Washington D C: Transportation Research Board, 2002.
[2] DONHAM K J, LEISTIKOW B, MERCHANT J, LEONARD S. Assessment of US poultry worker respiratory risks [J]. American Journal of Industrial Medicine, 1990,17(1): 73-74.
[3] SABIN L D, KOZAWA K, BEHRENTZ E, WINER A M, FITZ D R, PANKRATZ D V, FRUIN S A. Analysis of real-time variables affecting children’s exposure to diesel-related pollutants during school bus commutes in los angeles [J]. Atmospheric Environment, 2005,39(29): 5243-5254.
[4] US Environmental Protection Agency (EPA). National ambient air quality standards (NAAQS) [EB/OL]. [2010-11-17]. https://www3. epa.gov/ttn/naaqs/criteria.html.
[5] WITTENBERG B A, WITTENBERG J B. Effects of carbon monoxide on isolated heart muscle cells [J].Research Report Health Effects Institute, 1993,62: 1-12.
[6] US Environmental Protection Agency (EPA). Fact sheet proposed revisions to the national ambient air quality standards for nitrogen dioxide [EB/OL]. [2009-07-22]. https://www3.epa.gov/airquality/ nitrogenoxides/pdfs/20090722fs.pdf.
[7] ABOU-SENNA H, RADWAN E, WESTERLUND K, COOPER C D. Using a traffic simulation model (VISSIM) with an emissions model (MOVES) to predict emissions from vehicles on a limited-access highway [J]. Journal of the Air & Waste Management Association, 2013,63(7): 819-831.
[8] CHU H C, MEYER M D. Methodology for assessing emission reduction of truck-only toll lanes [J].Energy Policy, 2009,37(8): 3287-3294.
[9] de VLIEGER I. On board emission and fuel consumption measurement campaign on petrol-driven passenger cars [J]. Atmospheric Environment, 1997, 31(22): 3753-3761.
[10] HALLMARK S L, GUENSLER R, FOMUNUNG I. Characterizing on-road variables that affect passenger vehicle modal operation [J].Transportation Research Part D: Transport and Environment, 2002,7(2): 81-98.
[11] NESAMANI K S, CHU L, MCNALLY M G, JAYAKRISHNAN R. Estimation of vehicular emissions by capturing traffic variations [J]. Atmospheric Environment, 2007,41(14): 2996-3008.
[12] SUN Z, HAO P, BAN X, YANG D. Trajectory-based vehicle energy/emissions estimation for signalized arterials using mobile sensing data [J].Transportation Research Part D: Transport and Environment, 2015,34: 27-40.
[13] BEYCHOK M R.Fundamentals of stack gas dispersion [M]. Newport Beach, CA: Milton R BETCHOK, 1994.
[14] ARYA S P. Air pollution meteorology and dispersion [M]. Oxford: Oxford University Press, 1999.
[15] ULIASZ M, BARTOCHOWSKA M, MADANY A, PIWKOWSKI H, PARFINIEWICZ J, ROZKRUT A. Application of the mesoscale dispersion modeling system to investigation of air pollution transport in southern Poland [M]. New York, US: Springer, 1994.
[16] SHARMA N, CHADHRY K K, CHALAPATI RAO C V. Vehicular pollution prediction modelling: A review of highway dispersion models [J].Transport Reviews, 2004,24(4): 409-435.
[17] LIN J, GE Y E. Impacts of traffic heterogeneity on roadside air pollution concentration [J]. Transportation Research Part D: Transport and Environment, 2006, 11(2): 166-170.
[18] SAMARAAYAKE S, GLASER S, HOLSTIUS D, MONTEIL J, TRACTON K, SETO E, BAYEN A. Real-time estimation of pollution emissions and dispersion from highway traffic [J]. Computer-Aided Civil and Infrastructure Engineering, 2014, 29(7): 546-558.
[19] ZHANG K, STUART B. Air pollution and health risks due to vehicle traffic [J].Science of the total Environment, 2013,450: 307-316.
[20] SMIT R, SMOKERS R, RABE E. A new modelling approach for road traffic emissions: VERSIT+ [J]. Transportation Research Part D: Transport and Environment, 2007,12(6): 414-422.
[21] BENSON P.CALINE4-A dispersion model for predicting air pollutant concentrations near roadways [R]. No. FHWA/CA/TL- 84/15, 1984.
[22] BAN X, SUN Z.Simulation-based decision-making tool for adaptive traffic signal control on tarry town road in the city of white plains [R]. NY: New York State Department of Transportation, C-10-03, 2013.
[23] Illinois Department of Transportation. Transportation Data Management System [EB/OL]. [2013-11-26]. http://idot.ms2soft. com/tcds/tsearch.asp?loc=Idot&mod.
(Edited by DENG Lü-xiang)
Received date: 2015-09-20; Accepted date: 2016-03-01
Corresponding author: SUN Zhan-bo, PhD, Assistant Professor; Tel: +1-269-276-3203; E-mail: zhanbo.sun@wmich,edu