基于数据流挖掘技术的入侵检测模型与算法
毛伊敏1, 2,杨路明1,陈志刚1,刘立新1
(1. 中南大学 信息科学与工程学院,湖南 长沙,410083;
2. 江西理工大学 应用科学学院,江西 赣州,341000)a
摘要:针对目前基于数据流关联规则挖掘技术的入侵检测系统响应速度不够快和检测精度不够高的问题,提出一个基于数据流最大频繁模式的入侵检测系统模型MMFIID-DS;设计各种剪枝策略,挖掘经过训练学习后的正常数据集、异常数据集和当前检测数据流的最大频繁项集,建立系统的正常行为模式、异常行为模式和用户行为模式,达到极大缩小搜索空间的目的,提高系统的响应速度;结合误用检测和异常检测2种入侵检测方法进行实时在线检测入侵,提高系统的检测精度。理论与实验结果表明:MMFIID-DS入侵检测系统具有较好的性能。
关键词:数据流;最大频繁项集;异常检测;误用检测
中图分类号:TP 399 文献标志码:A 文章编号:1672-7207(2011)09-2720-09
An intrusion detection model based on data mining over data
MAO Yi-min1, 2, YANG Lu-ming1, CHEN Zhi-gang1, LIU Li-xin1
(1. School of Information Science and Engineering, Central South University, Changsha 410083, China;
2. Institute of Applied Science, Jiangxi University of Science and Technology, Ganzhou 341000, China)
Abstract: Aiming at the current problems of inadequacy in intrusion-detection system response speed and detecting precision of data mining techniques based on association rules of data streams, an intrusion detection system model of MMFIID-DS based on maximal frequent pattern of data streams was proposed. A variety of pruning strategies were proposed to mine the maximal frequent itemsets on trained normal data set, abnormal data set and current data streams to establish normal and abnormal behavior pattern as well as user behavior pattern of the system in order to improve response speed of the system by greatly reducing search space. Besides, misuse detection and anomaly detection techniques were combined to implement online real-time intrusion detection and improve detection precision of the system. Both theoretical and experimental results indicate that the MMFIID-DS intrusion detection system is fairly sound in performance.
Key words: data streams; maximal frequent itemsets; anomaly detection; misuse detection
随着计算机网络技术的快速发展与日益普及,越来越多的信息通过网络来传输与存储,网络安全问题日益突出。入侵检测(Intrusion detection)作为一种主动的防御技术被期望提供能实现对网络攻击的全方位检测[1]。由于关联规则分析技术能很好地获知发生攻击断言的原因或发生攻击的判断过程,能加深报警信息的理解,方便入侵检测系统的规则书写,已经广泛用于入侵检测系统的设计和构造中。传统的基于关联规则分析技术的入侵检测模型难以准确地描述网络数据随时间变化的情况,因此,利用动态的数据流挖掘技术构建网络入侵检测模型具有重要的理论和应用价值。自1980年Aderson提出入侵检测概念[2]以来,入侵检测方法得到了深入研究,采用关联规则技术来建立入侵检测模型在入侵检测领域发挥了日益重要的作用,其中经典的算法有ADAM[3],MADAMID[4]和MINDS[5]。ADAM算法应用单层关联规则挖掘模式寻找连接记录各属性之间的关联关系,应用多层关联规则挖掘模式寻找连接记录关于IP地址的高层抽象的关联规则,设计一些全程变量记录某些特征属性的统计值,这些值都是基于对已知攻击特征的理解和分析,并不能为发现新的入侵特征提供支持;MADAMID算法利用挖掘到的频繁模式进行特征构造,为连接记录添加新的构造特征,将分类学习算法用于包含新的构造特征的连接记录数据集上进行入侵检测。但是,所构造的特征只是有限的几个统计模式,没有构造出新的特征和未知的模式;MINDS系统使用无监督技 术,对每个网络连接设置一个值,用来反映每个连接的异常度,对标记很高异常度的网络连接进行关联模式分析发现异常行为。MINDS系统存在需要训练集进行分类和只分析数据的头部而没有分析负载数据的问题。由于传统的基于关联规则技术的入侵检测算法是对全部历史数据进行等同学习,不能准确反映网络的行为特征,另外,网络数据具有海量、数据持续到达等特点,上述算法进行关联模式挖掘需多次扫描数据信息,无法适应网络数据流信息的特点。因此,采用数据流模型描述随时间变化的网络数据并对其进行入侵检测处理得到了广泛的研究,其中经典的工作如下:Rahman等[6]提出“Apriori-frequent”算法,此算法不像传统的Apriori算法[7]那样由频繁项逐步生成频繁项集,它对每个无线网络连接预处理记录设置1个值,运用这个值分析不频繁与频繁模式来决定异常模式。但是,该算法的最小支持度设置太低,产生大量的频繁项集和少量的不频繁项集,导致有些攻击未被发现,系统的误报率会提高;Liu等[8]采用“交叉特征分析”方法从训练集中建立系统的正常行为模式,使用“关联规则分析”方法从检测数据集中建立当前行为模式,通过异常检测方法对整个系统进行入侵检测。但是,该系统没有把当前行为模式判断为新的正常行为模式放入到系统的正常行为模式中,会导致系统的误报率提高;毛国军等[9]采用MaxFP-Tree表示网络数据流的模式和生成数据流最近时期数据所隐含的最大频繁项目集,有机融合误用检测和异常检测2种入侵检测方法对系统进行入侵检测,达到提高检测精度的目的。但是,通过维护MaxFP-Tree结点挖掘网络数据流的模式,实时在线挖掘效果差,当网络数据流数据增大时,系统维护MaxFP-Tree结点数目也会增大,系统的响应时间大。在此,本文作者在对相关工作进行研究和分析的基础上,提出一个基于数据流最大频繁项集的入侵检测系统模型MMFIID- DS(Mining maximal frequent itemsets for intrusion detection over data streams), 设计各种剪枝策略,挖掘经过训练学习后的正常数据集、异常数据集和当前检测数据流的最大频繁项集,建立系统的正常行为模式、异常行为模式和用户行为模式,将误用检测和异常检测两种入侵检测方法有机地结合,进行实时在线检测入侵,达到提高检测精度和系统响应速度的目的。
1 基于数据流挖掘的入侵检测模型
网络数据的行为特征随时间不断变化,而数据流(Data stream)是指持续、快速到达的数据序列[10],因此,可用数据流表示随时间变化的网络数据,通过对数据流信息的正常或异常模式的挖掘,建立对应的网络访问模式库,利用已建立的各网络访问模式库,实现对未来网络访问数据的入侵分析。基于数据流挖掘的入侵检测模型如图1所示。
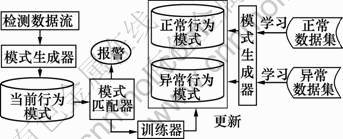
图1 入侵检测模型
Fig.1 Intrusion detection model
图1所示的入侵检测模型由实时入侵检测与系统模式库建立2部分构成,前者用于对当前到达的数据记录进行在线检测,由模式生成器与模式匹配器构成;后者主要用于正常行为模式库、异常行为模式库的建立,由模式生成器、正常行为模式库和异常行为模式库构成。
实时入侵检测的工作原理是:利用模式生成器把检测数据流信息的行为特征挖掘出来,形成当前行为模式;通过模式匹配器将当前行为模式与正常行为模式、异常行为模式库的模式进行匹配,把结果报告给用户,同时如果发现当前产生的行为模式与正常行为模式与异常行为模式的相似度均小于用户给定的阀值,则把当前行为模式放入训练器学习,经更新器更新修改正常行为模式与异常行为模式库。系统模式库的建立工作原理是:利用模式生成器把正常训练集与异常训练集的行为特征挖掘出来,构建正常行为模式库与异常行为模式库。
2 基于数据流挖掘的入侵检测算法
采用关联规则算法进行入侵检测的方法[11]如下:在正常数据集中频繁出现的行为是系统的正常行为模式,在短时间内频繁发生的行为不被系统正常行为模式覆盖被称为异常行为模式。因为最大频繁项集中隐含了所有的频繁项集,所以,本文采用最大频繁模式挖掘算法刻画入侵检测的正常、异常行为模式。其思路是:正常数据集与异常数据集中的最大频繁项集的集合作为系统的正常行为模式与异常行为模式,当前时间段内网络数据中的最大频繁项集的集合与系统的正常行为模式、异常行为模式进行相似度匹配,根据匹配原则把结果报告给用户或修改相应的系统行为模式库。
2.1 基本概念
包含攻击的网络数据集D可以看作大小无限的事务数据流序列DS,DS={T1, T2, …, Ti, …}。其中:Ti= {I1, I2, …, Im, …};Ii(i=1, 2, …, p)称为项目。滑动窗口SW(Sliding Window)包含数据流DS上最近的N个事务的数据。
定义1 项目集
,事务集
,定义映射关系:g: 2I→2T,g(x)={
|事务t支持项目x},称g(x)为项集
的支持集。f: 2T→2I,f(T)={
|T中的任何事务均支持,项目c},称f(T)是
的公共项集。
定义2
的绝对支持度为
,相对支持度是
除以
。
定义3 项目集
,项目集x的包含索引是:
。
定义4 给定生成子
,其中Y是一个频繁1-项集,
,定义生成子的支持度后序集:
定义5 给定生成子
,其中Y是一个频繁1-项集,
,定义生成子的支持度前序集:
定义6 若频繁项集(frequent itemsets,FI) X的所有超集都是非频繁项集,则称X为最大频繁项集。将所有最大频繁项集组成的集合称为最大频繁集(Maximum frequent itemsets,MFI)。
定义7 设A和B是逻辑同构的2个数据集合,各自的最大频繁项目集记为:MFA={(A1, CA1), (A2, CA2), …, (Am, CAm)}和MFB={(B1, CB1), (B2, CB2), …, (Bm, CBm)},其中:1≤i≤m,(Ai, CAi)是A的第i个最大频繁项集和它的支持数;1≤j≤n,(Bj, CBj)是B的第j个最大频繁项集和它的支持数。那么,A和B的相似性定义为:
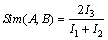
式中:
;
;
。
定义8 设
和
是用户给定的阀值,A是正常行为模式库中最大频繁项集,B是异常行为模式库中最大频繁项集,C是当前行为模式库中最大频繁项集。若Sim(C, B)>
,则C是异常模式,入侵发生;若Sim(C, A)>
,则C是正常模式;若Sim(C, A)<
并且Sim(C, B)<
,则C是异常模式,入侵发生并将C加入异常规则库。
2.2 数据结构
Li等[12]提出用二进制滑动技术表示数据流信息,极大地提高了算法的时间效率,但是,这种方法在窗体滑动时,每个项目先进行二进制位左移操作,然后新增事务的值添加到最右边的位置,极大耗费维护代价。本文提出用项目字典二进制位置换技术置换过时的事务,新增当前的事务,无需左移操作,直接设置新增事务的值,减小维护代价,提高时间效率。网络数据流事务的项目按照字典序列排列,设项目xi,如果xi在当前事务中出现则用1表示,否则用0表示。当窗口移动时,用最近事务所包含项目的二进制位表示置换过时事务包含项目的二进制位。依据项目字典二进制位置换技术思想,提出一个新的数据结构— BV-MIL(Maximal itemsets lattice with bit-vectors),其特点如下:
(1) 它由一个BV-array(A array of bit-vectors of array)、FI-list(Frequent item list)和MFI-tree(Maximal frequent item tree)组成;
(2) FI-list的每个项包含4个域:itemnane,count,rownum和link。itemname代表项目名;count代表项目的频繁支持度计数;rownum记录项目在BV-array中列的位置;link指向CFI-tree根结点为itemname值的首结点的指针;
(3) MFI-tree的每一个结点由2个域组成:itemname,和next。itemname代表最大频繁项目名;count代表闭频繁项目的支持度计数;next为指向下一个结点,当下一个结点不存在时,next为null。
2.3 系统行为模式库的建立
根据网络数据流正常数据集与异常数据集的信息,经数据预处理及学习后,挖掘其最大频繁项集并将其存入正常行为模式库与异常行为模式库。最大频繁项集挖掘过程如下:首先发现频繁1-项集所产生的支持度最大的最长频繁项集,然后使用各种剪枝策略快速挖掘最大频繁项集。
定理1 对于
,若spt(x1)<spt(x2),则x1比x2所生成的频繁项集少。
证明:
spt(x1)=|g(x1)|,spt(x2)=|g(x2)|,
又
spt(x1)<spt(x2),
|g(x1)|<|g(x2)|。
根据定义2知:g(x1)<g(x2)。即支持x1的事务数少于支持x2的事务数。故x1比x2所生成的频繁项 集少。
由定理1与定义4可知:对频繁1-项集按支持度由低到高进行排序,每个1-项集所产生的包含索引含有的频繁项集多于不按支持度排序所产生的包含索引含有的频繁项集。
定理2 在MMFIID-DS算法流程中,若 spt(x)>s,则
,且
。
证明:(1) 当
时,
,
又
,
。
(2) 当
时,
,
即支持1-项集x的subsume(x)的事务与支持1-项集x的事务一样。
支持1-项集x的事务与支持
的事务也一样。
即:
(1)
,
, (2)
又
spt(x)>s,
>s。
即:
。
由式(1)和(2)得:
。
定理3 在MMFIID-DS算法流程中,对于
-项集,
,若
>s,
。
证明:

,

≤
。

>s,

>s,
即:
。
由定理3的分析可知:每个1-项集所产生的包含索引不是最大频繁项集,还需对其进行扩展操作,本文称“深度扩展策略”(Depth extended strategy, DES)。
定理4 在MMFIID-DS算法流程中,对于
-项集,
,若
,则
。
证明:设
。
,
。
由定义6可知:
≥s。
由定理3可知:
≥s,
即:
,
。问题得证。
由定理4的分析可知:若每个频繁1-项集与它的包含索引的并集是已发现最大频繁项集的子集,则此频繁1-项集产生的所有枚举项目都是最大频繁项目的子集,因此不需对其搜索,本文称“不扩展策略”(No extended strategy, NES)。
通过以上分析,把网络数据流的正常数据集与异常数据集的信息储存在BV-array中,在此存储结构基础上应用各种策略发现最大频繁项集的过程如算法1所示。
算法1 MiningMFI-DS。
输入:存储正常数据集、异常数据集事务的BV-array;
输出:MFI;
For each
FIlist, i=1, 2, …, n
If
>s then
/*计算所有包含项目xi的事务的交
If
then
Search(
, post_sets);
Endif
Else
If
then

;
Endif
Endif
Endfor
Procedure Search(mfi_set,post_sets)
While
do
;
;

If
>s then

For all
do
If
≥s then

Else
Exit
Endif
Endfor
;
Else
If
then

Endif
Endif
Endwhile
2.4 当前行为模式库的建立
根据网络数据流当前检测数据集的信息,经数据预处理后,挖掘其最大频繁项集。最大频繁项集挖掘过程如下:首先,把当前检测数据流的事务作为当前窗口的事务,按照上述系统行为模式库建立的方法挖掘最大频繁项集;然后,随着网络数据流地流动,过时的事务从当前窗口流出,新的事务流进当前窗口,应用各种搜索、剪枝策略,实时在线挖掘当前窗口所有事务的最大频繁项集。其详细过程如算法2和算法3所示。
定理5 在MMFIID-DS算法流程中,
,
,若
<s,则
<s。
证明:由算法1可知:项集mi存在2种情况:
(1) 当
时,
由定理2可知:项集mi为xi,其
。
若
<s,则
<s。
(2)
时,
由定理2可知:
,
。 (3)
,
≤
。 (4)
若
<s,由式(3)和(4)得:
<s。
由定理5的分析可知:随着时间的流失,若项目xi的支持度从大于用户规定的最小支持度变化到小于用户规定的最小支持度,则xi所生成的最大频繁项集的支持度也会小于用户规定的最小支持度,因此,这些最大频繁项集都要被剪枝,本文称“不频繁项剪枝策略”(Non-frequent items pruned strategy, NFIPS)。
定理6 在MMFIID-DS算法流程中,
(Td为将要删除的事务),
,若删除Td,则
。
证明:
,由2.2的项目集表示知,在Td的事务中,xi设置为0。
。 (5)
,
Td被删除,由式(5)得:
。
由定理6的分析可知:删除过时事务Td,若
中的项目,不对xi生成的所有最大频繁项集进行重新搜索,不会影响整个最大频繁项目集的正确输出,本文称“不搜索策略”(NSS,dynamic non-search strategy)。
通过上述分析,删除过期检测网络事务数据流及添加检测网络事务数据流的过程如算法2和算法3所示。
算法2 DeleTrans。
输入:BV-MIL;
输出:MFI;
第(tid mod N)位全置0;
Foreach
, i=1, 2, …, p/*Td为过时事务
Updata spt(xi);
If spt(xi)≥s then
从BV-MIL 中删除xi的所有生成子;

Search(
, post_sets);
Else
从BV-MIL 中删除xi的所有生成子;
Endif
Endfor
算法3 InseTrans。
输入:BV-MIL ;
输出:MFI;
Foreach
, i=1, 2, …, p/*Tnew为新增事务
第(tid mod N)位置1;
Update spt(xi);
Endfor
Resort(FIlist);
Foreach
, i=1, 2, …, p/*Tnew为新增事务
If spt(xi)≥s then
从BV-MIL 中删除xi的所有生成子;

Search(
, post_sets);
Endif
Endfor
2.5 网络访问数据流中的入侵检测算法
正常数据集的数据信息经数据预处理及学习后,挖掘其最大频繁项集并存入数据集MFA中,同理,挖掘经数据预处理及学习后的异常数据集的最大频繁项集并存入到MFB中,挖掘当前经数据预处理后的网络检测数据流的最大频繁项集,存入数据集MFC中,根据定义8的判定标准,检测网络入侵情况,见算 法4。
算法4 CheckIntrusion。
输入:
,
,MFA,MFB,MFC
输出:入侵检测情况
计算Sim(MFC, MFA),Sim(MFC, MFB);
If Sim(MFC, MFB)>
then
输出异常模式,结束
Else
If Sim(MFC, MFA)>
then
输出正常模式,结束
Else
当前数据流放入训练器
Endif
Endif
3 实验研究
3.1 实验数据
为了更准确地对入侵检测模型进行评价,采用的数据集应体现出随时间变化的特点,本文选择KDD CUP 1999数据集[13]作为测试集,对MIT Lincoln实验室[14]提供的3周纯净训练数据和含有攻击的训练数据对MMIID-DS进行验证。KDD CUP 1999是入侵检测领域广泛使用的标准测试集,由41条属性构成1条记录,其中有21个为关键属性。测试数据集中正常数据记录占73.8%,已知入侵类型数据占16.76%,未知入侵类型数据占9.44%。由于KDD原始数据集过于庞大,为了对系统进行验证,本文选择了其中具有代表性的15%数据集作为测试集。
3.2 性能评价指标
入侵检测系统使用的相关性能评价指标有:报警率(Alarm rate)、预警率(Warning rate)与误报率(False positive rate),其中:
报警率=(正确检测到的入侵记录数/入侵记录总数)×100%;
预警率=(检测到的入侵记录数/数据集总记录 数)×100%;
误报率=(被误报为入侵的正常记录数/正常记录总数)×100%。
3.3 实验结果和分析
将KDD数据集作为从起始时刻开始接收到的数据流,假设数据匀速到达。实验在2种情况下评估系统的各项性能:(1) 实验在测试数据流流进初始窗口的所有数据为训练集,对系统进行攻击检测(以下简称第1种情况);(2) 实验在检测数据流为当前窗口的所有数据为训练集,即实验在连续100个过时的网络检测数据流流出当前窗口,连续100个新的网络检测数据流流进当前窗口,对系统进行实时在线攻击检测(以下简称第2种情况)。
3.3.1 系统的运行时间评估
实验在数据量为3 000,5 000,10 000,30 000,50 000,70 000和100 000条记录时,最小支持度(s)分别设为0.01%,0.1%和0.5%评估系统在第1种情况和第2种情况的处理时间变化,如图2所示。
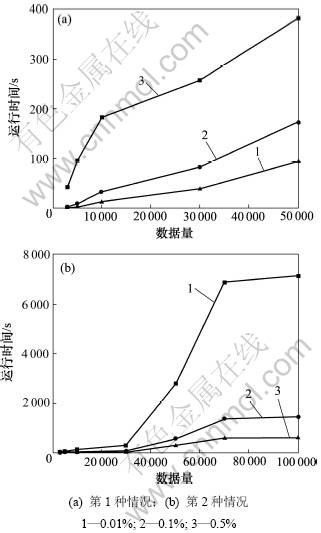
图2 随数据增加的系统运行时间变化
Fig.2 Processing time with increasing data
从图2可以看出:在不同的支持度下,随着数据流量的增长,MMFIID-DS系统在第1种情况下系统的运行时间呈线性增长,在第2种情况下系统的运行时间在一定数值范围内上下波动。这是因为在窗口初始阶段,初始窗口的尺寸增大,系统处理的数据量增大,运行时间就会变大。在窗口滑动阶段,系统处理的数据量会随时间的变化进行线性增长变化,但是数据量达到7 000条左右时,系统的运行时间趋于稳定值,这是因为用户的正常行为模式趋于完善。
3.3.2 系统的检测指标评估
实验首先在数量为3 000条记录时,评估系统在第1种情况下报警率、预警率和误报率的变化。然后,数据量不断增大,评估系统在第2种情况下数据量为 5 000,10 000和30 000条记录时系统的报警率、预警
率和误报率的变化。其中实验使用的最小支持度分别为0.2%,1%和5%,测试结果分别如表1~3所示。
表1 s=0.2%系统的检测指标
Table 1 Detection metric of system with s=0.2%
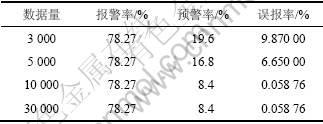
表2 s=1%系统的检测指标
Table 2 Detection metric of system with s=1%
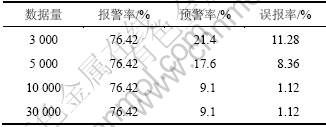
表3 s=5%系统的检测指标
Table 3 Detection metric of system with s=5%
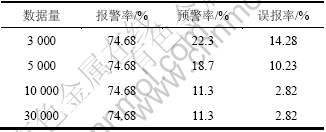
从表1~3可以看出:随着用户最小支持度s的增大,系统的误报率也增大,这是由于用户最小支持度设置过高导致系统挖掘出最大频繁项集的数量减少,系统的正常行为模式库与异常行为模式库还处于不太完善状态。但是,随着网络数据流量的增多,系统的误报率逐渐趋于一个较合理的值,因为数据集中未发现正常记录与入侵记录对应的模式逐渐存入正常行为模式库及异常行为模式库中,系统的正常行为模式库与异常行为模式库趋于完善。
3.3.3 不同系统的运行时间比较
系统运行时间的比较。实验在当前窗口的数据流出,连续100个新的当前网络检测数据流流进当前窗口,对系统进行实时在线攻击检测。实验的最小支持度分别设为0.2%,0.4%和0.6%来评估本系统与文献[9]中系统的运行时间,结果如表4所示。
表4 系统运行时间的比较
Table 4 Comparison of processing time of system s
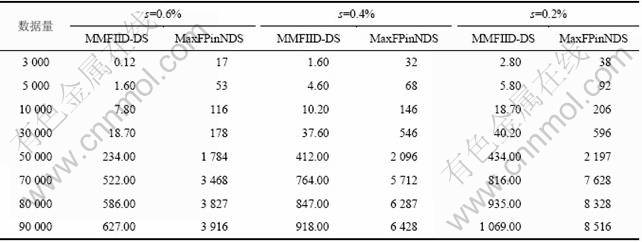
从表4可以看出:在不同的支持度下,本系统比文献[9]中MaxFPinNDS系统的运行时间短。这是因为本系统采用了多种剪枝策略,极大地缩小搜索空间,快速挖掘最大频繁项集,提高了系统的响应时间。
图3所示为系统误报率的比较结果。当数据量在 3 000,5 000,10 000和30 000条记录时,检测本系统与文献[9]中系统的误报率,其中实验的支持度为0.2%,文献[9]中衰减率为0.999。
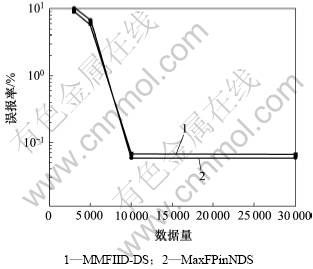
图3 误报率的比较
Fig.3 Comparison of false positive rate
从图3可以看出:网络数据量达到10 000个记录之前,本系统的误报率比MaxFPinNDS系统的误报率略高,但是随着网络数据量的增大,2个系统的误报率都会降得很低。这是因为2个系统都采用误用检测和异常检测2种检测方法对系统进行检测。
4 结论
(1) 针对目前采用数据流关联规则分析技术的入侵检测系统响应速度不够快和检测精度不够高的问题,提出一个基于数据流最大频繁模式的入侵检测系统模型MMFIID-DS。
(2) 通过运用“深度扩展策略”、“不扩展策略”、“不频繁剪枝策略”和“不搜索策略”,快速挖掘经过训练学习后的正常数据集、异常数据集和当前检测数据流的最大频繁模式建立系统的正常行为模式、异常行为模式和用户行为模式。
(3) 综合使用误用检测和异常检测2种入侵检测方法,实时在线检测入侵。
参考文献:
[1] 罗守山, 温巧燕, 杨义先. 入侵检测[M]. 北京: 北京邮电大学出版社, 2004: 47-48.
LUO Shou-san, WEN Qiao-yan, YANG Yi-xian. Intrusion detection[M]. Beijing: Beijing University of Posts and Telecommunications Press, 2004: 47-48
[2] Anderson J P. Computer security threat monitoring and surveillance[EB/OL]. [1980-05-18]. http://csrc.nist.gov/ publications/history/ande80.pdf.
[3] Barbará D, Couto J, Jajodia S. ADAM: A testbed for exploring the use of data mining in intrusion detection [J]. SIGMOD, 2001, 30(4): 15-24.
[4] Stolfo S J, Lee W, Chan P K, et al. Data mining-based intrusion detectors: An overview of the Columbia IDS project[J]. SIGMOD Record, 2001, 30(4): 5-14.
[5] Ertoz L, Eilertson E, Lazarevic A. The MINDS-Minnesota intrusion detection system in next generation data mining [EB/OL]. [2004-08-09]. http://www.cs.umn.edu/research/ MINDS/MINDS_papers.htm.
[6] Rahman A, Ezeife C I, Aggarwal A K. WiFi Miner: An online apriori-infrequent based wireless intrusion based wireless intrusion detection system[C]//Proceeding of the 2nd International Workshop on Knowledge Discovery from Sensor Data. Las Vegas, American, 2008: 63-72.
[7] Agrawal R, Srikant R. Fast algorithms for mining association rules[C]//Proceedings of the 20th International Conference on Very Large Data Bases. Santiago, Chile, 1994: 487-499.
[8] LIU Yu, YANG Li, MAN Hong. A hybrid data mining anomaly detection technique in ad hoc networks[J]. International Journal of Wireless and Mobile Computing, 2009, 2(1): 37-46.
[9] 毛国军, 宋东军. 基于多维数据流挖掘技术的入侵检测模型与算法[J]. 计算机研究与发展, 2009, 46(4): 602-609.
MAO Guo-jun, ZONG Dong-jun. An intrusion detection model based on mining multi-dimension data streams[J]. Journal of Computer Research and Development, 2009, 46(4): 602-609.
[10] Dong G, Han J, Lakshmanan L. Online mining of changes from data streams: Research problems and preliminary result[C]//Proc of the 2003 Workshop on Management and Processing of Data Streams. New York, American, 2003: 225-236.
[11] 王卉. 最大频繁项集挖掘算法及应用研究[D]. 武汉: 华中科技大学计算机科学与技术学院, 2005: 68-71.
WANG Hui. Algorithms of maximal frequent itemset mining and their applications[D]. WuHan: Huazhong University of Science and Technology. School of Computer Science & Technology, 2005: 68-71.
[12] LI Hua-fu, Lee S Y. Mining frequent itemsets over data streams using efficient window sliding techniques[J]. Exper Systems with Applications, 2009, 36(2): 1466-1477.
[13] Center for Machine Learning and Intelligent System[EB/OL]. [1999-10-28]. http://www. Kdd.ics.uci.edu/databases/kddcup99/ kddcup99. html
[14] Massachusetts Institute of Technology[EB/OL]. [2009-06-05]. http://www.11.mit.edu/IST/ideval/data/1998/1998_data_index.html
(编辑 杨幼平)
收稿日期:2010-11-03;修回日期:2011-02-24
基金项目:国家自然科学基金面上资助项目(60873082)
通信作者:毛伊敏(1970-),女,新疆伊宁人,博士研究生,从事数据挖掘研究;电话:13767717480;E-mail: mymlyc@163.com